Physics augmented classification of fNIRS signals
Biosignal Processing and Classification Using Computational Learning and Intelligence(2022)
摘要
Background. Predictive classification favors performance over semantics. In traditional predictive classification pipelines, feature engineering is often oblivious to the underlying phenomena. Hypothesis. In applied domains, such as functional near infrared spectroscopy (fNIRS), the exploitation of physical knowledge may improve the discriminative quality of our observation set. Aim. Give exemplary evidence that intervening the physical observation process can augment classification. Methods. We manipulate the observation process in four ways independently. First, sampling and quantization are designed to enhance class-related contrast. Second, we show how selection of optical filters affects the cross-talk, in turn, affecting classification. Third, we regularize the inverse problem to maximize sensitivity to any gradient that would later support the classification. And fourth, we introduce a catalyst covariate during experiment design to exacerbate response differences. Results. For each of the proposed manipulations, we show that the performance of the classification exercise is altered in some way or another. Conclusions. Exploitation knowledge of physics even before acquisition can support classification, alleviating otherwise blind feature engineering. This can also enhance interpretability of the classification model.
更多查看译文
关键词
signals,classification
AI 理解论文
溯源树
样例
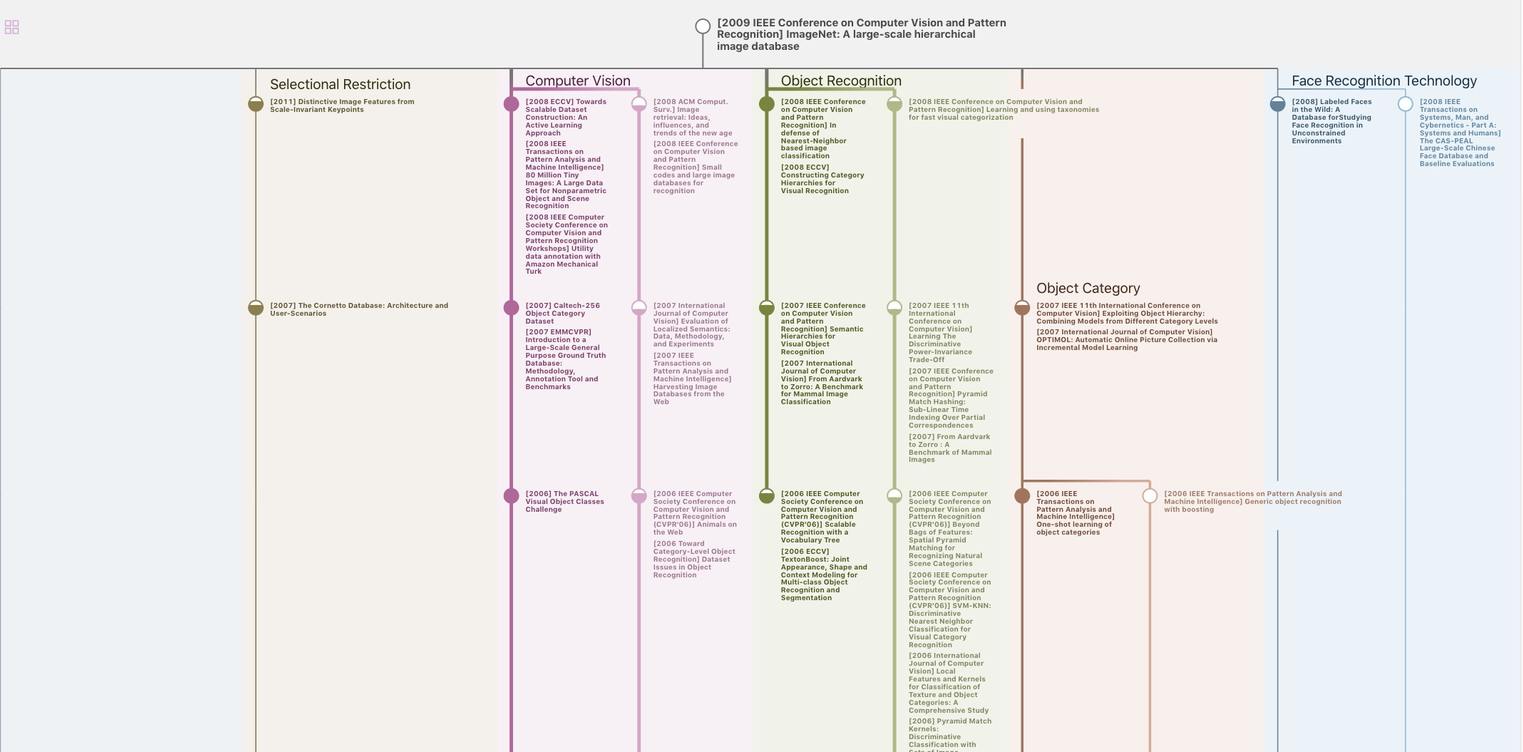
生成溯源树,研究论文发展脉络
Chat Paper
正在生成论文摘要