SNIP-FSL: Finding task-specific lottery jackpots for few-shot learning
Knowledge-Based Systems(2022)
摘要
Foresight pruning is an effective approach for deriving a compact sub-network in resource-constrained scenarios. However, most existing methods neglect the fact that scarce resources usually imply insufficient trainable data, thereby considerably affecting the pruning performance. In this paper, we propose a novel single-shot pruning method, named SNIP-FSL, for these few-shot tasks to achieve a trade-off between resources and data scale. Considering the pretrained weights as a special initialization state, we argue that there are task-sensitive high-performance sparse subnetworks in the few-shot learning process, termed “task-specific lottery jackpots.” By designing an effective parameter significance criterion, we obtained these jackpots without additional time-consuming searches and iterations. Furthermore, SNIP-FSL is designed to distinguish task-specific lottery jackpots based on historical experience; thus, it can be easily integrated into most transfer- and meta-based methods. For example, we obtain a winning submodel that has only 30% parameters without compromising accuracy in vanilla ProtoNet. Extensive experimental results reveal that SNIP-FSL attains excellent performance compared with several state-of-the-art foresight pruning methods under both transfer- and meta-paradigms.
更多查看译文
关键词
Network pruning,Meta learning,Few-shot learning,Fine-tuning,Transferable parameters
AI 理解论文
溯源树
样例
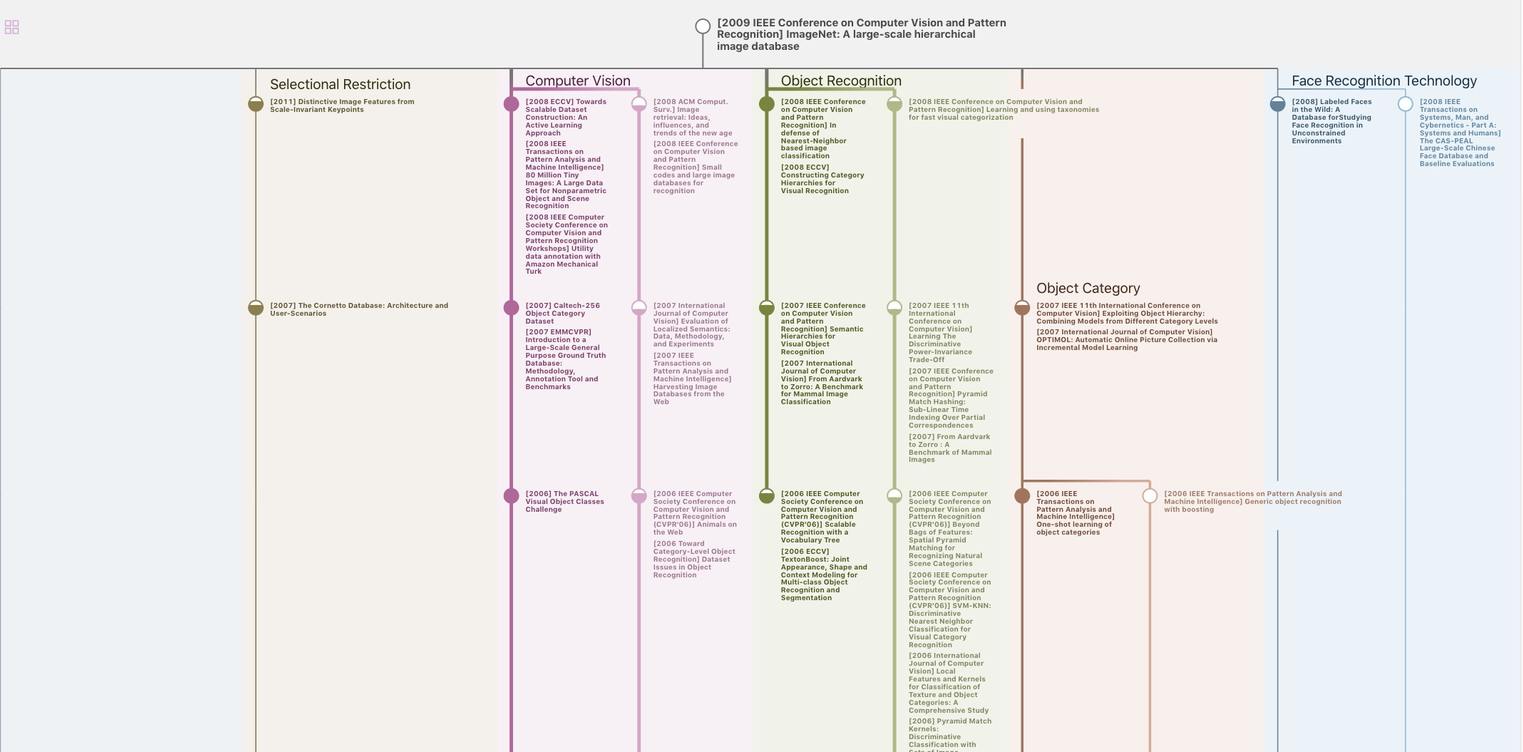
生成溯源树,研究论文发展脉络
Chat Paper
正在生成论文摘要