Traffic congestion detection: data-based techniques
Road Traffic Modeling and Management(2022)
摘要
Traffic congestion has a negative impact on traffic performance because it increases travel time and air pollution. Therefore detecting traffic congestion is a key element in facilitating the development of efficient intelligent transportation systems. Motivated by the high capacity of principal component analysis (PCA) methods in describing the correlation structure underlying multivariate data, the aim of this chapter is demonstrating the performance of PCA-based methods in monitoring traffic flow. First, in this chapter, we present two commonly applied denoising methods that can be used for data pre-filtering, as traffic flow data are usually contaminated with noise. Then we present the basic steps needed to model multivariate traffic data using PCA. We also show five well-known procedures that are frequently used to determine the PCA model order, namely, cumulative percent variance, cross-validation, scree test, parallel analysis, and eigenvalue 1 rule. Essentially, to detect traffic congestion, PCA is constructed using congestion-free data. Then the new data are referenced to this model, where any anomalous traffic condition can be detected. Here we present four monitoring indices commonly used with PCA: SPE, T2, combined SPE and T2, and amalgamated exponential smoothing schemes. Then we assess the effectiveness of the presented PCA-based monitoring techniques using traffic measurements from the Old Bayshore Hwy on the south of Interstate 880 (I880) in California and Ashby Ave from the west of Interstate 80 (I80) highway in the San Francisco Bay Area. The results highlight that the PCA with nonparametric thresholds provided improved detection performance compared with the conventional PCA-based schemes. Finally, we discuss the limitations of the presented monitoring approaches and offer some plausible directions to rectify these limitations.
更多查看译文
关键词
traffic congestion detection,data-based
AI 理解论文
溯源树
样例
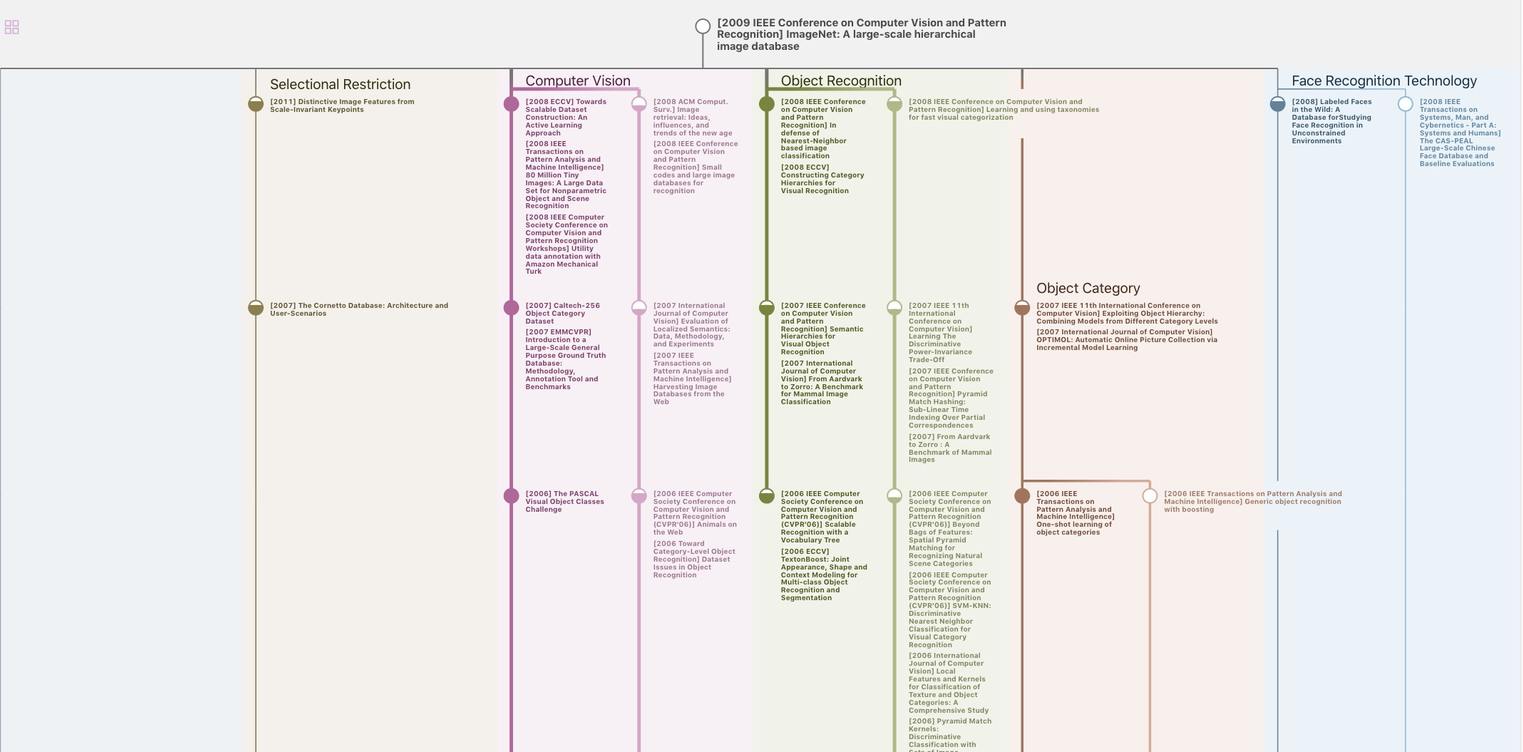
生成溯源树,研究论文发展脉络
Chat Paper
正在生成论文摘要