Identifying Ethereum traffic based on an active node library and DEVp2p features
Future Generation Computer Systems(2022)
摘要
With the prevalence of blockchain technology, Ethereum, as the second-largest blockchain, with the capabilities of running smart contracts, grows fast. A user on the Ethereum platform can implement smart contracts as well as remit its cryptocurrency. Cyber-attacks often target cryptocurrency. Besides, Ethereum may be exploited by malicious users for launching attacks. Therefore, the demand for Ethereum network supervision is increasing. To protect interior users on Ethereum from attacks, an Internet Service Provider (ISP) should supervise the Ethereum traffic from or to its internal users. Ethereum traffic identification is the first step towards supervision. Ethereum is a peer-to-peer communication network implemented by DEVp2p. DEVp2p is designed to realize data interaction and adopts encryption to enhance anonymity on Ethereum. It is difficult to identify Ethereum traffic accurately at line speed in a high-speed network. So far, Ethereum traffic identification has not been explored yet. This paper designs an efficient Ethereum traffic identification system with high identification accuracy, dubbed ETI (Ethereum Traffic Identification), for an ISP to supervise its internal users on Ethereum without Deep Packet Inspection (DPI). ETI first constructs an active node library consisting of core nodes on Ethereum and active Ethereum peers in the ISP. Then potential Ethereum flows are filtered by checking if their sources or destinations are in the active node library. As active Ethereum nodes may generate non-Ethereum traffic, machine learning classifiers are further adopted to judge if the potential Ethereum traffic is actually from Ethereum. The classification is based on the unique packet/payload-length and port number-related traffic features on Ethereum implemented by DEVp2p. Our experimental studies validate that ETI outperforms state-of-the-art network traffic classification methods in identification accuracy and time efficiency. ETI achieves an identification accuracy of 0.9998 and relatively high time efficiency.
更多查看译文
关键词
00-01,99-00
AI 理解论文
溯源树
样例
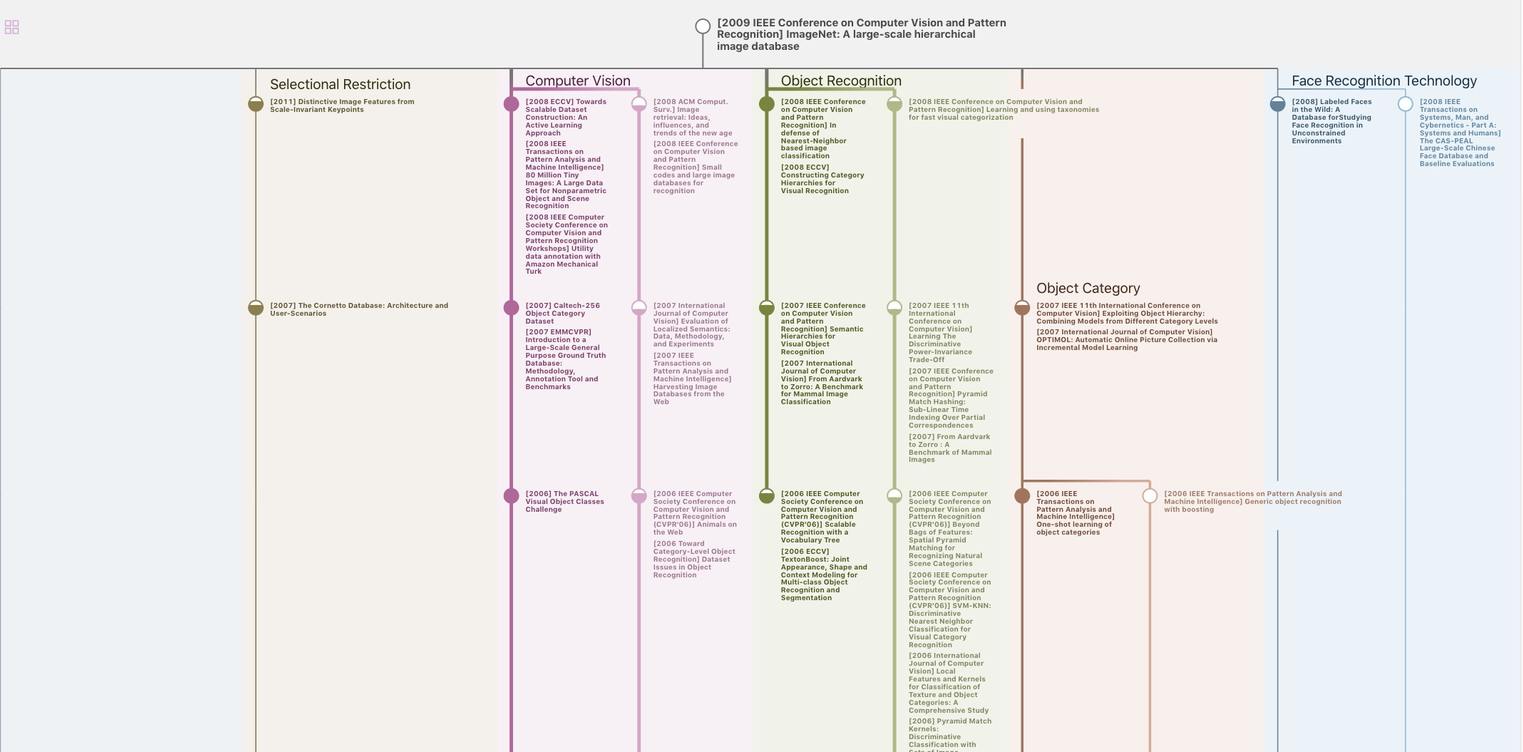
生成溯源树,研究论文发展脉络
Chat Paper
正在生成论文摘要