Transformer-Based Streaming ASR with Cumulative Attention
IEEE International Conference on Acoustics, Speech, and Signal Processing (ICASSP)(2022)
摘要
In this paper, we propose an online attention mechanism, known as cumulative attention (CA), for streaming Transformer-based automatic speech recognition (ASR). Inspired by monotonic chunk-wise attention (MoChA) and head-synchronous decoder-end adaptive computation steps (HS-DACS) algorithms, CA triggers the ASR outputs based on the acoustic information accumulated at each encoding timestep, where the decisions are made using a trainable device, referred to as halting selector. In CA, all the attention heads of the same decoder layer are synchronised to have a unified halting position. This feature effectively alleviates the problem caused by the distinct behaviour of individual heads, which may otherwise give rise to severe latency issues as encountered by MoChA. The ASR experiments conducted on AIShell-1 and Librispeech datasets demonstrate that the proposed CA-based Transformer system can achieve on par or better performance with significant reduction in latency during inference, when compared to other streaming Transformer systems in literature.
更多查看译文
关键词
End-to-end ASR,Transformer,online attention mechanism,cumulative attention
AI 理解论文
溯源树
样例
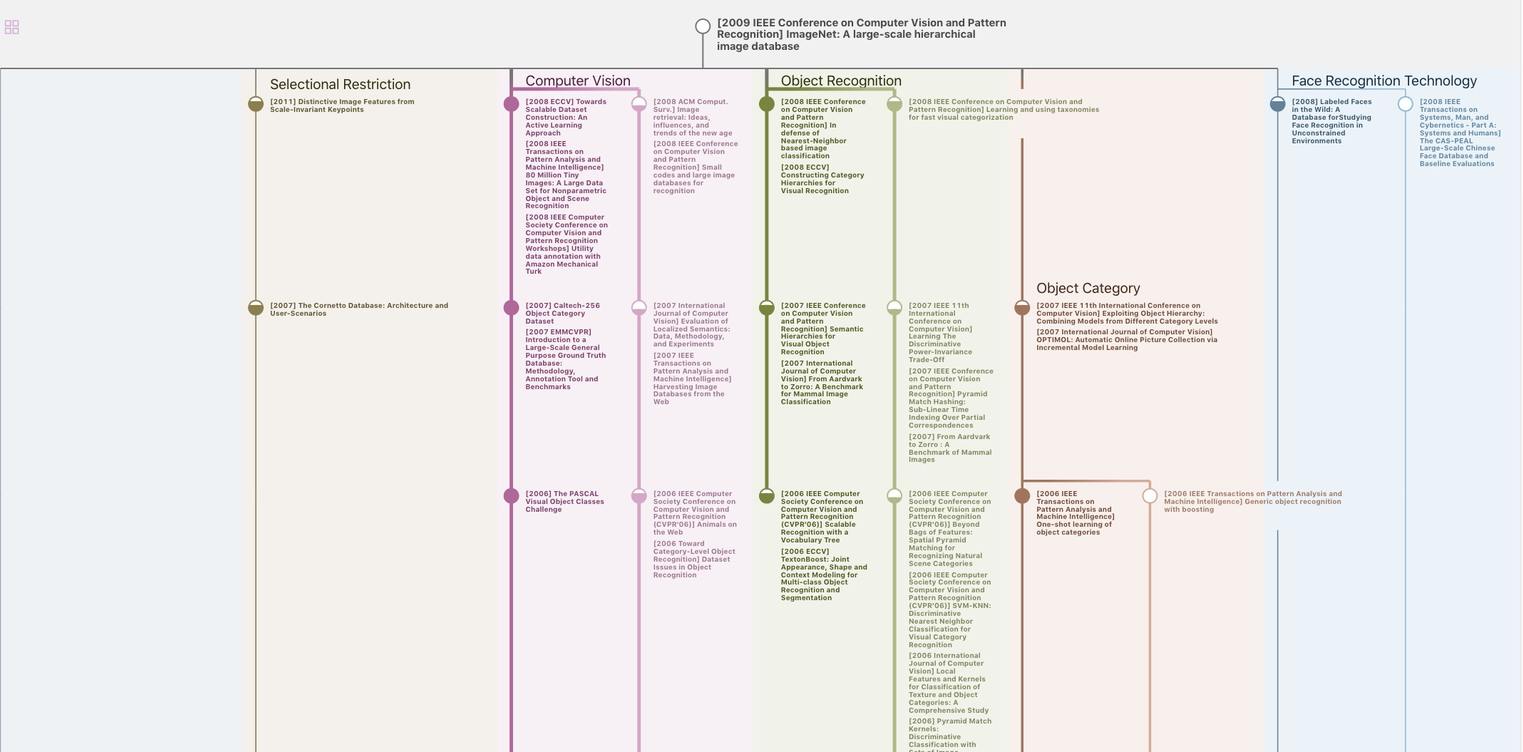
生成溯源树,研究论文发展脉络
Chat Paper
正在生成论文摘要