Optimizing Electrocaloric Effect in Barium Titanate-based Room Temperature Ferroelectrics: Combining Landau Theory, Machine Learning and Synthesis
SSRN Electronic Journal(2022)
摘要
The search for ferroelectrics with optimized electrocaloric effects is often hindered due to the large compositional space and lack of reliable property data. Phenomenological theory, such as the Landau approach, then becomes useful, provided such models can be reliably parametrized with experimental data. We propose an approach in which we combine a surrogate model formed from widely available polarization data to predict entropy changes using polarization from the machine learned model as input to the Landau model. Our objective is to search for room temperature BaTiO3 based calorics, and we validate our approach by synthesizing compounds guided by optimal experimental design using the surrogate model for polarization. We iterate our design loop four times, predicting and synthesizing four compounds, the electrocaloric effects of which are estimated using both indirect and direct characterization. The compositions show competitive electrocaloric performance at room temperature with a large adiabatic temperature change ΔT ∼0.6 K at 20 kV/cm in a wide temperature window (∼30 K with 90%ΔT). Moreover, we find that the homogeneous Landau models are largely predictive in the linear regime where the change in entropy varies quadratically with polarization. We discuss the role of nonlinear and diffusive effects for larger entropy behavior. The proposed approach shows promise in the use of theory or physics based models to bridge with output from machine learning methods when limited data is available for a property of interest.
更多查看译文
关键词
Machine learning,Landau theory,Experimental design,Electrocaloric effect
AI 理解论文
溯源树
样例
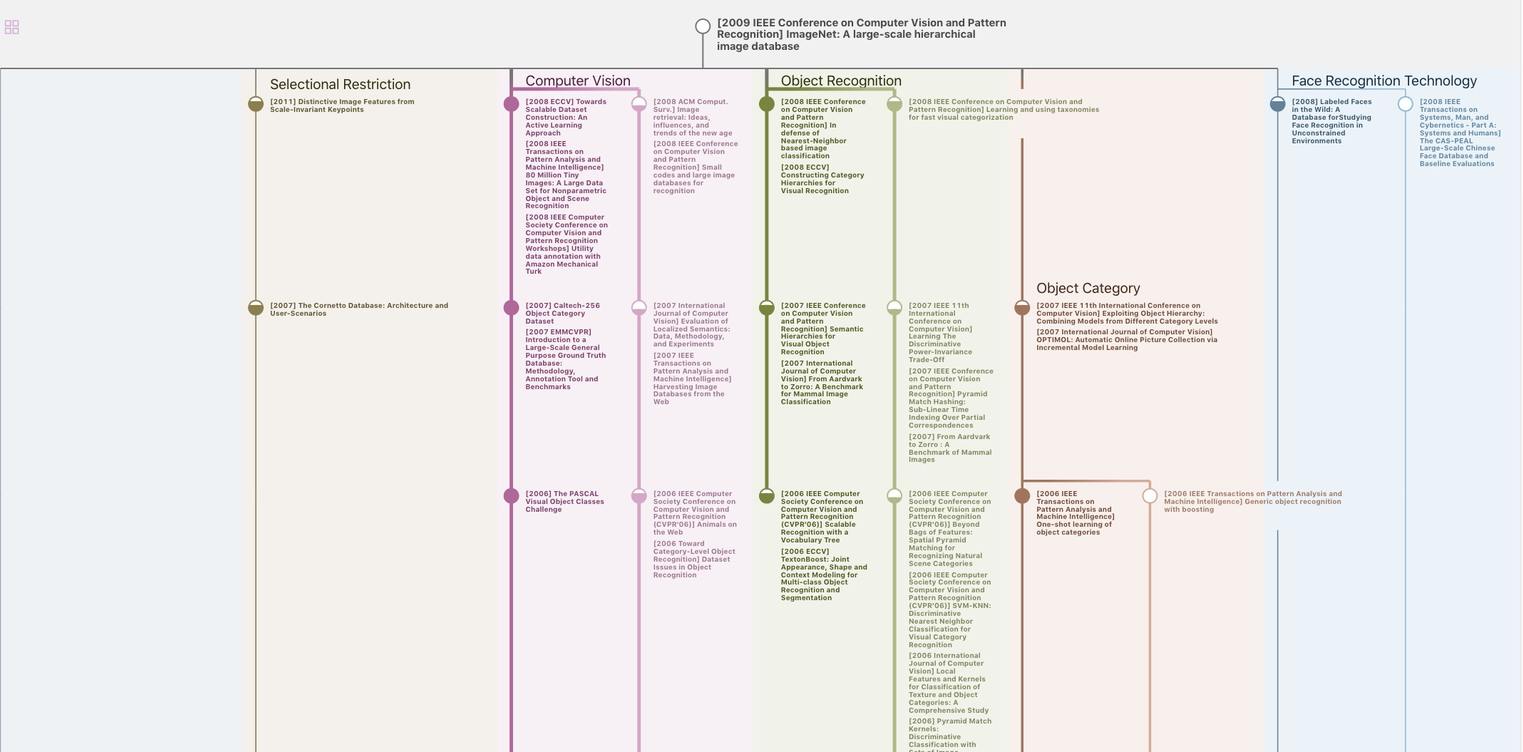
生成溯源树,研究论文发展脉络
Chat Paper
正在生成论文摘要