GAGIN: generative adversarial guider imputation network for missing data
Neural Computing and Applications(2022)
摘要
Missing data imputation aims to accurately impute the unobserved regions with complete data in the real world. Although many current methods have made remarkable advances, the local homogenous regions, especially in boundary, and the reason of the imputed data are still the two most challenging issues. To address these issues, we propose a novel Generative Adversarial Guider Imputation Network (GAGIN) based on generative adversarial network (GAN) for unsupervised imputation, which is composed of a Global-Impute-Net (GIN), a Local-Impute-Net (LIN) and an Impute Guider Model (IGM). The GIN looks at the entire missing regions to generate and impute data as a whole. Considering the reason of the GIN results, IGM is assigned to capture coherent information between global and local and guide the LIN to look only at a small area centered at the missing focused regions. After processing these three modules, the local imputed results are concatenated to those global imputed results, which impute the rational values and refine the local details from rough to accurate. The comprehensive experiments demonstrate our proposed method is significantly superior to the other three state-of-the-art approaches and seven traditional methods, and we achieve the best RMSE surpass the second-best method on both numeric datasets (17.3%) and image dataset (24.1%). Besides, the extensive ablation study validates the superior performance for dealing with missing data imputation.
更多查看译文
关键词
Missing data imputation,Imputation guider,Local refine,Deep learning
AI 理解论文
溯源树
样例
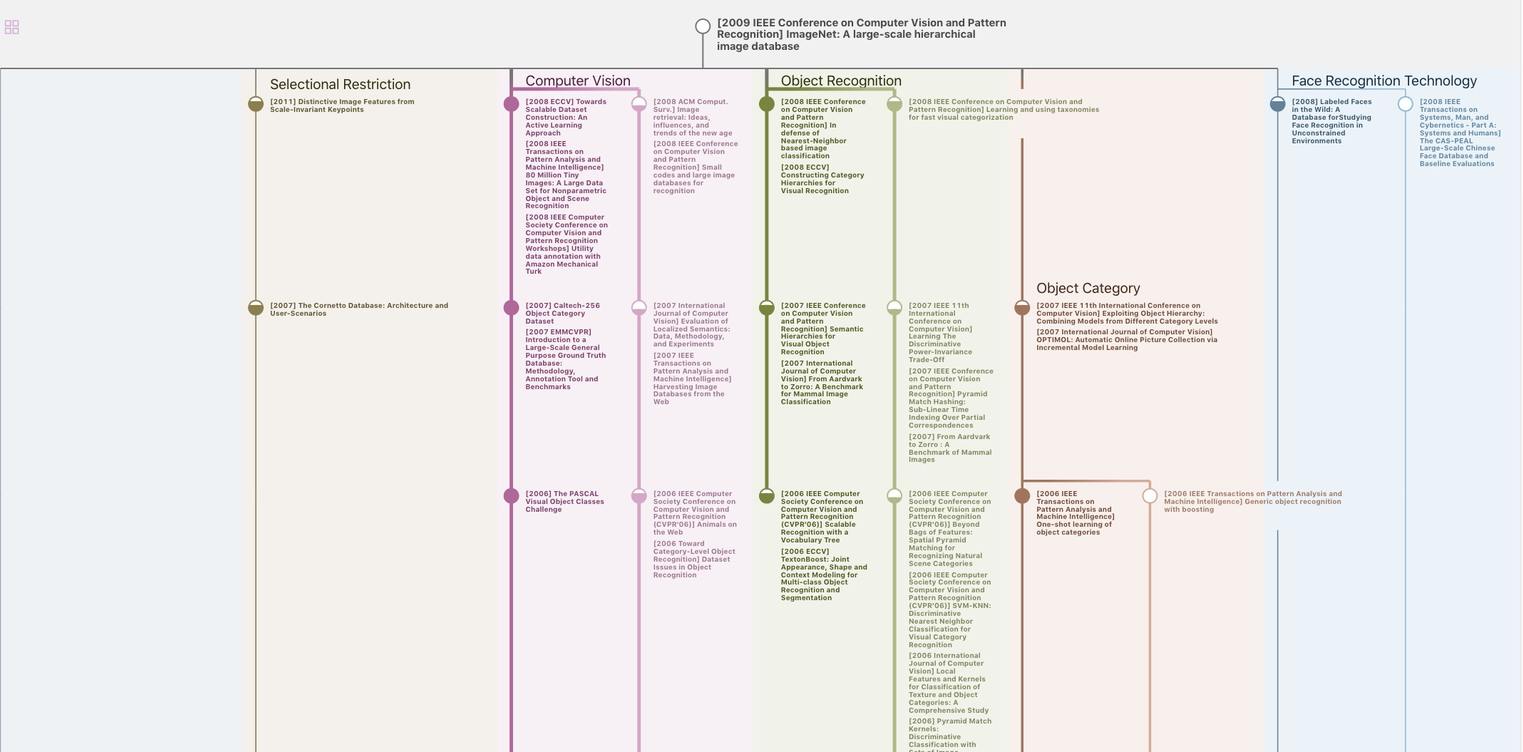
生成溯源树,研究论文发展脉络
Chat Paper
正在生成论文摘要