Fore-Net: Efficient inlier estimation network for large-scale indoor scenario
ISPRS Journal of Photogrammetry and Remote Sensing(2022)
摘要
Point clouds scanned in the real-world environment, especially for cluttered indoor spaces, are often corrupted by extraneous points from sensor noise or missing points because of occlusion or partially overlapped situations. These problems reduce the performance of subsequent tasks, especially for point cloud registration. This paper proposes a learning-based network, Fore-Net, to efficiently filter out outlier points and retain only inlier points, which can promote the performance of subsequent algorithms by optimizing the quality of source data. We designed a dual space attention module in Fore-Net that can enrich the network's learning by aggregating information from both feature and cartesian spaces and realizing information exchange between point cloud pairs. In addition, the paper proposes two automatic labeling strategies that enable Fore-Net to be trained and tested to detect overlapping regions and denoise the point clouds using existing datasets without hand labeling. We comprehensively evaluated Fore-Net using two large-scale indoor datasets (the 3Dmatch and S3DIS datasets) and one synthetic dataset (the ModelNet40 dataset). The results showed that Fore-Net was more accurate and robust than the state-of-the-art approaches, and Fore-Net could generalize well in different types of indoor scenes. We further validated that by using Fore-Net as a pre-processing step, the performance of registration algorithms, whether classical methods or learning-based methods, could be promoted in the low-overlapping scenario; Fore-Net could also be used as an effective tool to denoise the point clouds of indoor scenes and objects. The results demonstrate Fore-Net's efficiency and compatibility, which will make it attractive for tasks relying on high-quality source data.
更多查看译文
关键词
Inlier estimation,Low-overlapping point cloud registration,Indoor scenario,Point cloud denoising
AI 理解论文
溯源树
样例
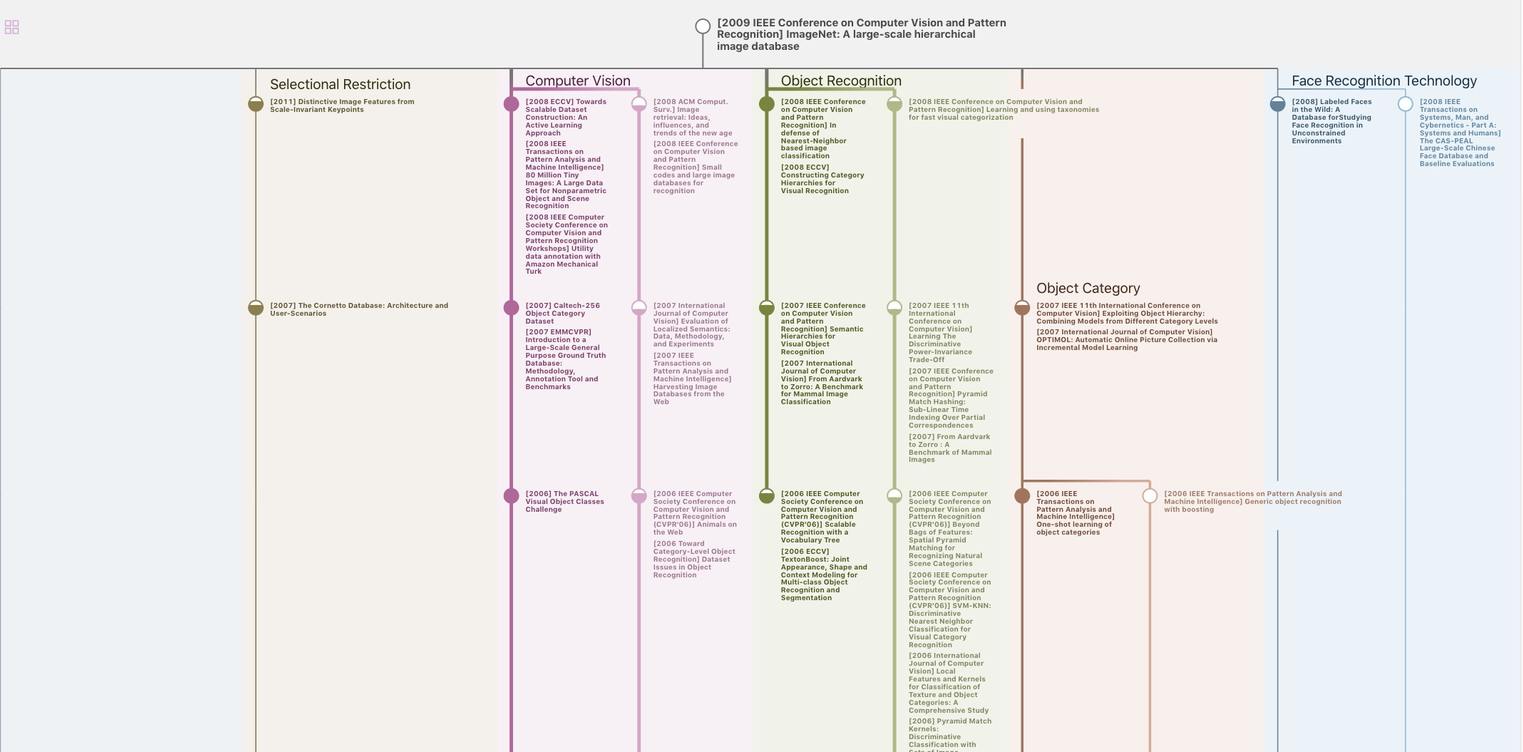
生成溯源树,研究论文发展脉络
Chat Paper
正在生成论文摘要