A Sparse Online Approach for Streaming Data Classification via Prototype-Based Kernel Models
Neural Processing Letters(2022)
摘要
Processing big data streams through machine learning algorithms has various challenges, such as little time to train the models, hardware memory constraints, and concept drift. In this paper, we show that prototype-based kernel classifiers designed by sparsification procedures, such as the approximate linear dependence (ALD) method, provides an adequate tradeoff between accuracy and size complexity of kernelized nearest neighbor classifiers. The proposed approach automatically selects relevant samples from the training data stream to form a sparse dictionary of prototypes, which are then used in kernelized distance metrics to classify arriving samples on the fly. Additionally, the proposed method is fully adaptive, in the sense that it updates and removes prototypes from the dictionary, enabling it to learn continuously in nonstationary environments. The results obtained from a comprehensive set of computer simulations involving artificial and real streaming data sets indicate that the proposed algorithm can build models with low complexity and competitive classification error rates compared to state of the art.
更多查看译文
关键词
Stream data classification,Kernel methods,Prototype-based models,Sparsification
AI 理解论文
溯源树
样例
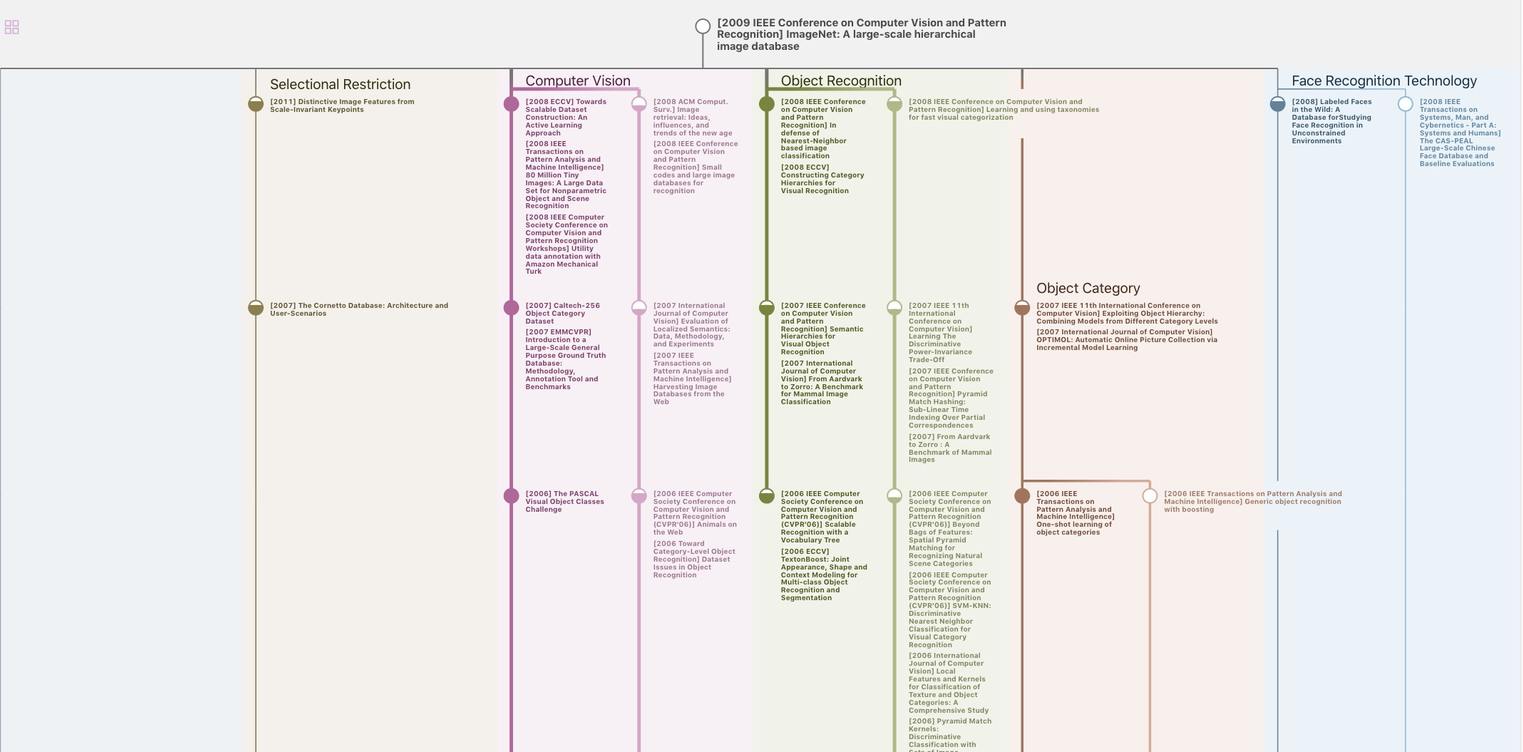
生成溯源树,研究论文发展脉络
Chat Paper
正在生成论文摘要