Taris: An online speech recognition framework with sequence to sequence neural networks for both audio-only and audio-visual speech
COMPUTER SPEECH AND LANGUAGE(2022)
摘要
It is widely accepted that the visual modality of speech provides complementary information to the speech recognition task, and many models have been introduced in order to make good use of the visual channel. This article develops Taris, a fully differentiable neural network model capable of decoding both audio-only and audio-visual speech in real time. We achieve this by connecting our previously proposed models AV Align and Taris, which are both end-to -end differentiable approaches to audio-visual speech integration and online speech recognition respectively. We evaluate AV Taris under the same conditions as AV Align and Taris on one of the largest publicly available audio-visual speech datasets, LRS2. Our results show that AV Taris is superior to the audio-only variant of Taris, demonstrating the utility of the visual modality to speech recognition within the real time decoding framework defined by Taris. Compared to an equivalent Transformer-based AV Align model that takes advantage of full sentences without meeting the real-time requirement, we report an absolute degradation of approximately 3% with AV Taris. As opposed to the more popular alternative for online speech recognition, namely the RNN Transducer, Taris offers a greatly simplified fully differentiable training pipeline. We speculate that AV Taris has the potential to popularise the adoption of Audio-Visual Speech Recognition (AVSR) technology and overcome the inherent limitations of the audio modality in less optimal listening conditions.1
更多查看译文
关键词
Online speech recognition,Audio-visual speech integration,Learning to count words,Multimodal speech processing,Speech recognition
AI 理解论文
溯源树
样例
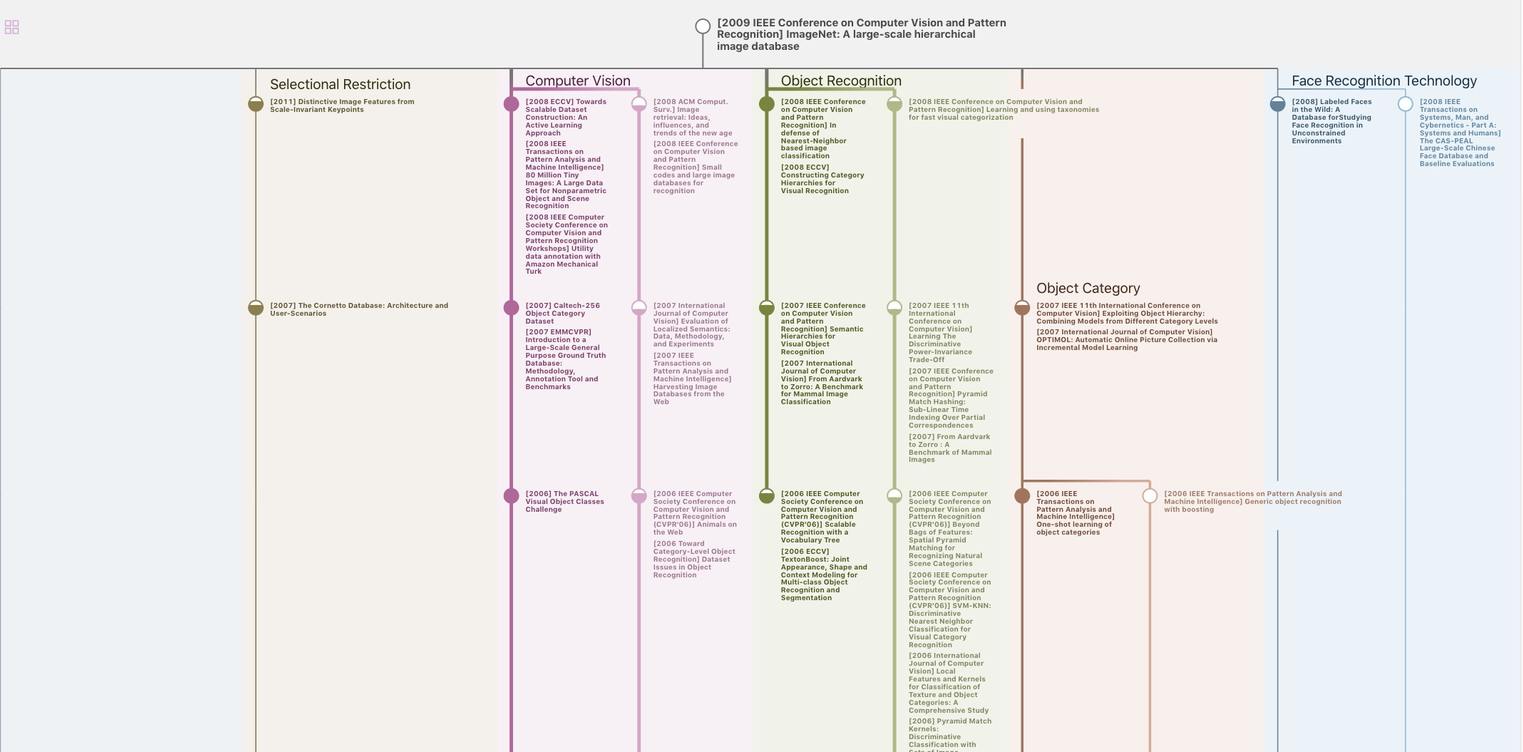
生成溯源树,研究论文发展脉络
Chat Paper
正在生成论文摘要