Enhanced IMU Sensors Fusion based on Adaptive Least Square Windowing Technique
2021 International Telecommunications Conference (ITC-Egypt)(2021)
摘要
Many applications in our daily life need accurate orientation and motion information. The Inertial Measurement Unit (IMU) is a perfect sensor for that purpose, because of its small size, low power consumption, and affordable price. These low-cost IMUs suffer from several sources of noise, that cause large divergence over time during the absence of an absolute positioning system. In addition to its poor performance, low-cost IMUs do not have reliable noise metrics data information in their vendor specification. Multiple IMUs can be utilized to enhance the overall system performance through fusion. As gyroscope errors have the main contribution in deciding the navigation system performance, this paper proposes two adaptive weighting least square approaches. During fusion, the weights of the LS filter are changed online according to two windowing methodologies, these windowing approaches estimate the bias, noise density, and scale factor level for each sensor measurements inside this window of time, which is then used to estimate the variance accompanied by each sensor that will be used as a weight inside the LS algorithm, these weights are adaptively changing with each period. The second methodology is also based on two different size window techniques, with cascaded LS filter topology. MATLAB navigation toolbox is utilized to generate two gyroscope IMUs row data with different noise configurations like noise density, random walk, and bias instability rates…etc. to imitate real-life raw measurements. These raw measurements are then used to validate and evaluate the two proposed algorithms, by comparing their RMSE to a standard LS method RMSE. The simulation was repeated 100 times and the RMSE of the proposed methodologies showed enhancement in the estimated measurements by more than 20% when compared to the standard LS, under the condition that the noise figures of the sensors are completely random.
更多查看译文
关键词
Least Square,Gyroscopes,IMU,Multiple-IMUs,Adaptive Least Square
AI 理解论文
溯源树
样例
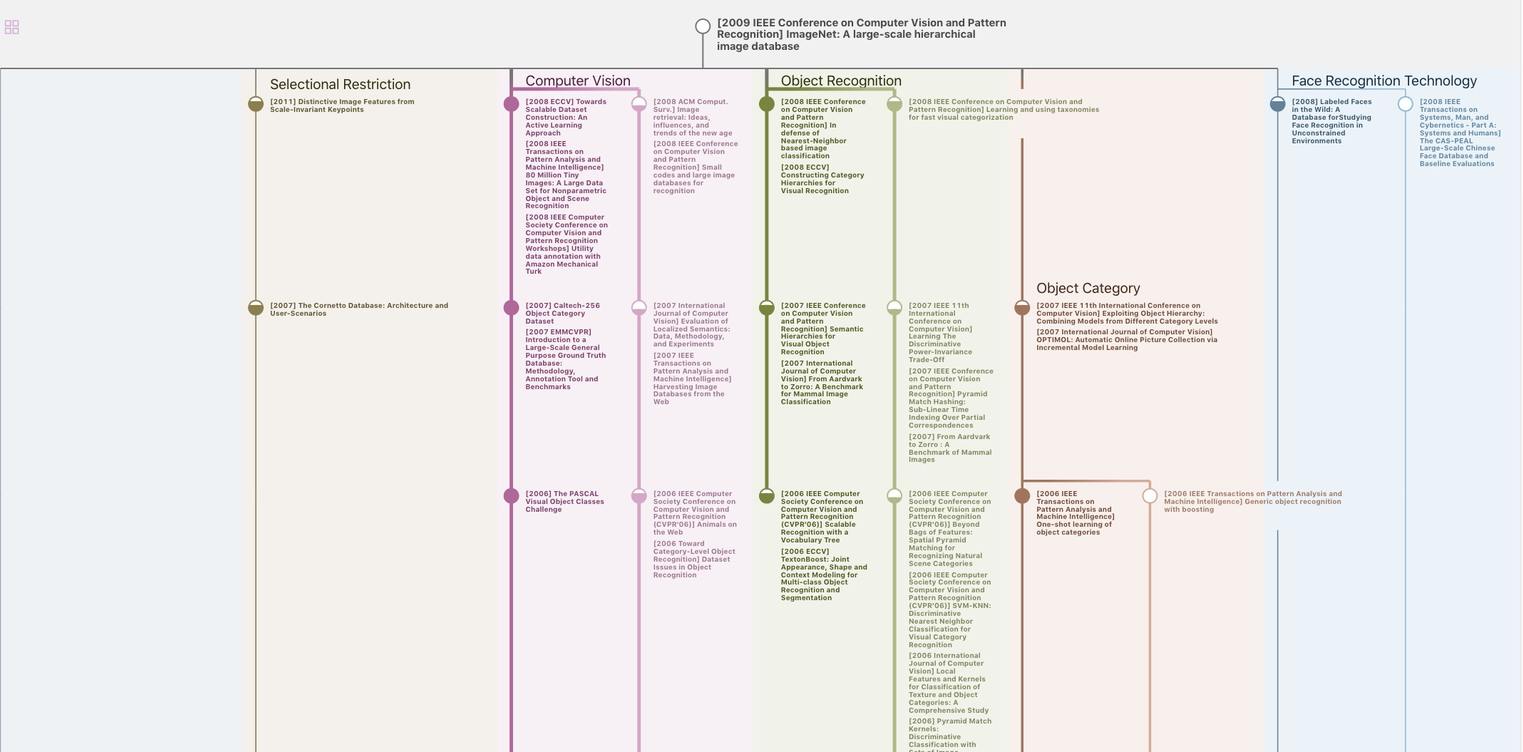
生成溯源树,研究论文发展脉络
Chat Paper
正在生成论文摘要