Improving Deep Learning-Based Cloud Detection for Satellite Images With Attention Mechanism
IEEE Geoscience and Remote Sensing Letters(2022)
摘要
Clouds in satellite images limit the ability of imagery to extract the ground information, which makes it difficult to the following image analysis tasks. Hence, cloud detection is a changeling but fundamental task in the preprocessing of satellite image processing. In this letter, an encoder-decoder neural network architecture, cloud detection with ACON and attention mechanism (CAA)-UNet, is proposed for cloud detection. CAA-UNet is based on U-Net architecture and incorporates the attention mechanism. The asymmetric encoder and decoder blocks are proposed to discover more discriminative features. Then, a modified attention gate is integrated into each skip connection to highlight salient features. These make our model distinguish between the cloud and noncloud more accurately. Furthermore, the recent new and effective activate function, ActivateOrNot (ACON), is introduced into our model, which allows each neuron to adaptively activate or not, and improves the performance remarkably. Finally, the experiment results on two cloud datasets, Landsat-8 and a high-resolution cloud (HRC) cover validation dataset, show that CAA-UNet outperforms the state-of-the-art methods, especially in comprehensive indicators: Jaccard index, F-1 score, and overall accuracy.
更多查看译文
关键词
Activation function,attention mechanism,cloud detection,deep learning (DL),satellite images
AI 理解论文
溯源树
样例
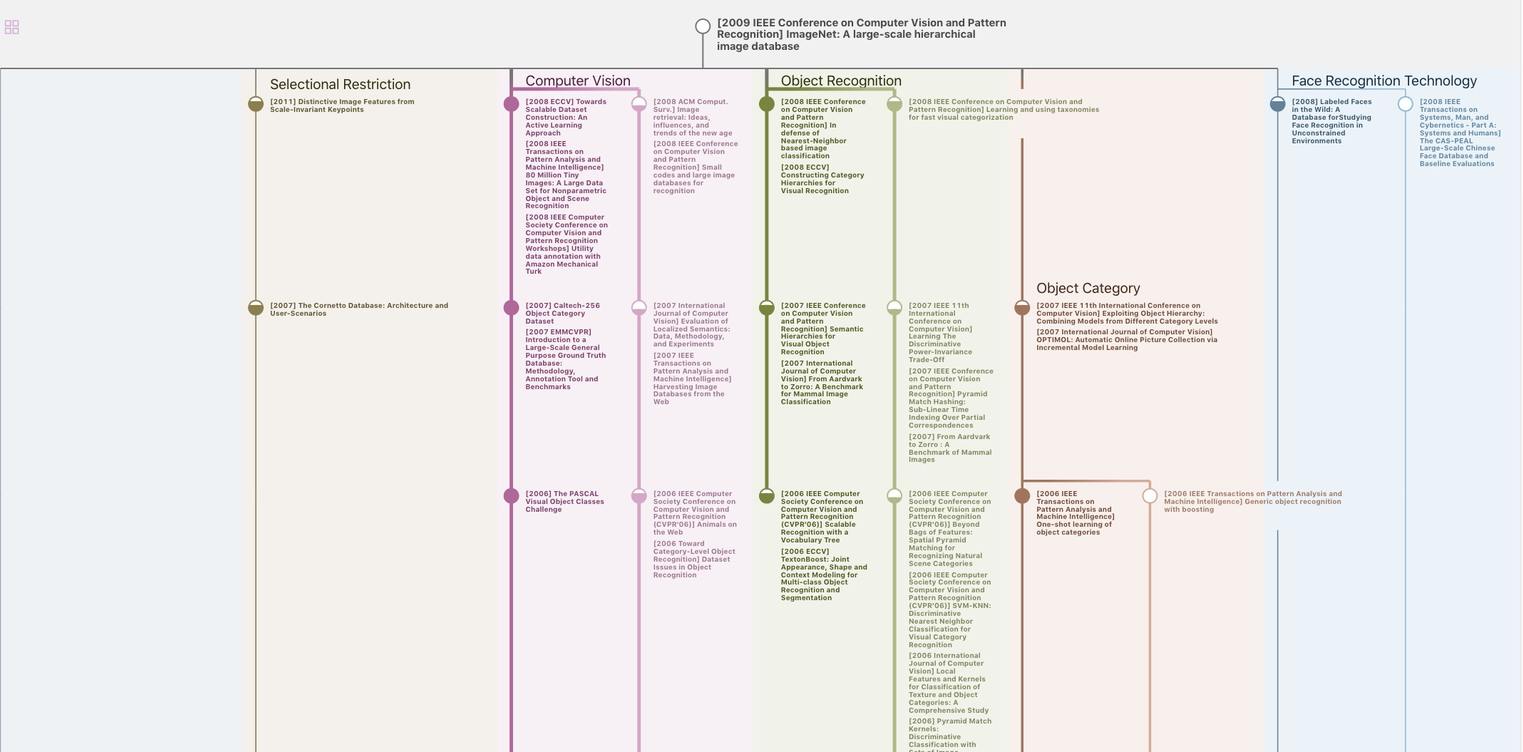
生成溯源树,研究论文发展脉络
Chat Paper
正在生成论文摘要