MARS: Learning Modality-Agnostic Representation for Scalable Cross-Media Retrieval
IEEE Transactions on Circuits and Systems for Video Technology(2022)
摘要
Cross-media retrieval (CMR) offers a flexible retrieval experience across multiple modalities. Existing CMR approaches are constrained by the assumption that the paired modalities are available in training, and they leverage the data of all modalities to obtain a common representation. However, as dealing with the data from new modality, the previous all modalities need to be re-trained, compromising the flexibility and practicality of CMR. In this paper, we propose an approach termed learning Modality-Agnostic Representation for Scalable cross-media retrieval (MARS), which allows each modality to be trained independently. To be specific, MARS treats the label information as a distinct modality, and introduces a label parsing module LabNet to generate semantic representation for correlating different modalities. Meanwhile, MARS constructs the modality-specific representation module DataNet to obtain the modality-shared representation and modality-exclusive representation equipped with unbiased semantic classification. Technically, for the first modality, we jointly train the LabNet and its DataNet to preserve the semantic similarity between the Label-derived representation and the modality-shared representation. For new modalities, MARS employs the well-learned LabNet to extract the representation in labels, and then such representation is served as the privilege to guide the associated DataNet training via the same objective. Furthermore, we assign the same classifier to the representation module of all modalities for better semantic alignment. With the above schema, the obtained modality-shared representation is considered to be modality-agnostic. Extensive experiments on several benchmark multi-modality datasets demonstrate that the proposed MARS achieves better results than existing methods.
更多查看译文
关键词
Multi-modality learning,cross-media retrieval,modality scalability,similarity retrieval
AI 理解论文
溯源树
样例
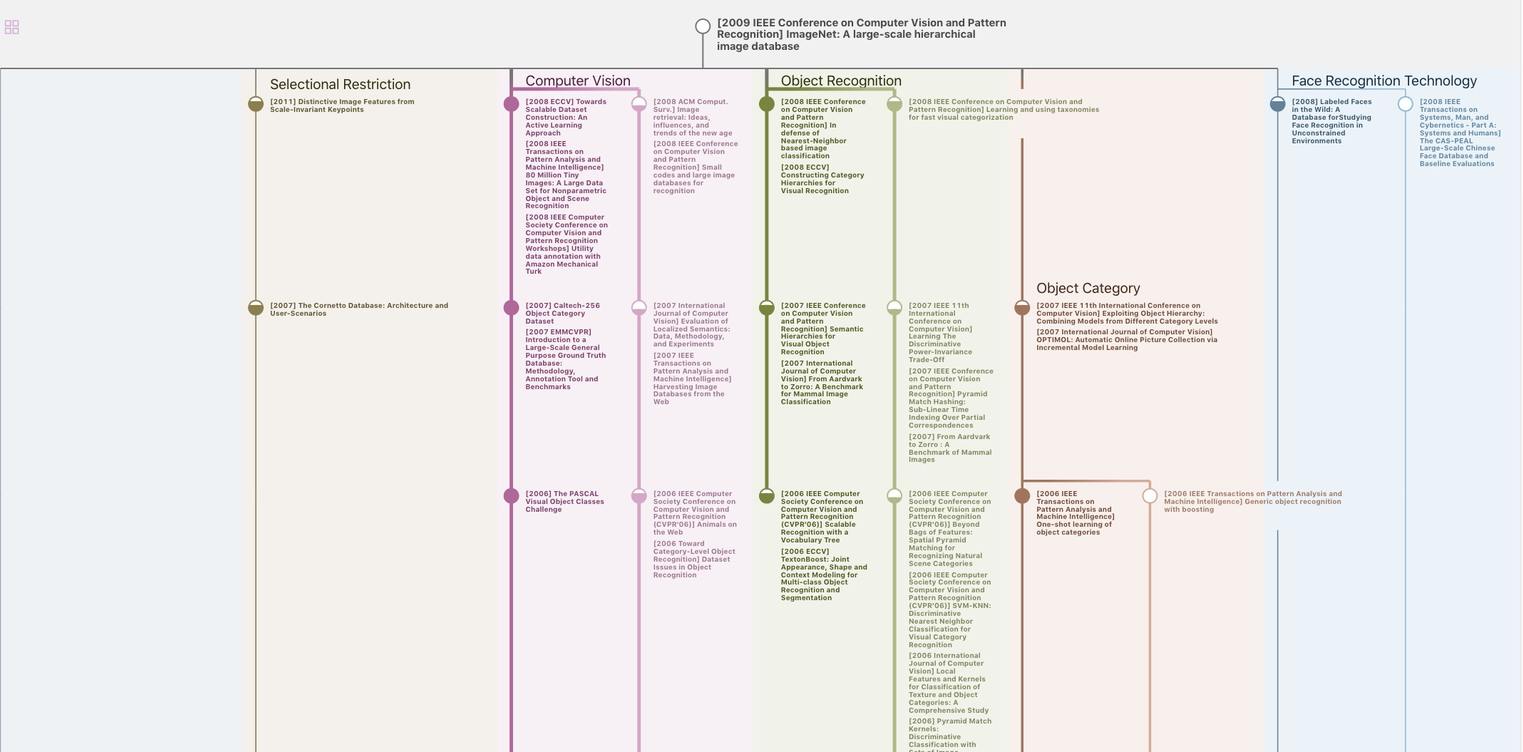
生成溯源树,研究论文发展脉络
Chat Paper
正在生成论文摘要