Feature Selection Based on Evolutionary Algorithms for Affective Computing and Stress Recognition
Engineering proceedings(2021)
摘要
In the area of affective computing, machine learning is used to recognize patterns in datasets based on extracted features. Feature selection is used to select the most relevant features from the large number of extracted features. Conventional feature selection methods are associated with a high computational cost depending on the classifier used. This paper presents a feature selection approach based on evolutionary algorithms using techniques inspired by natural evolution to optimize the computational process. Our method is implemented using an Optimize Selection operator from RapidMiner and is integrated within our previously developed workflow for affective computing and stress recognition from biosignals. The performance is evaluated based on the random forests classifier and a cross validation using our uulmMAC database for machine learning applications. Our proposed approach is faster than the forward selection method at similar recognition rates and does not stop at a local optimum, allowing a promising feature selection alternative in the field of affective computing.
更多查看译文
AI 理解论文
溯源树
样例
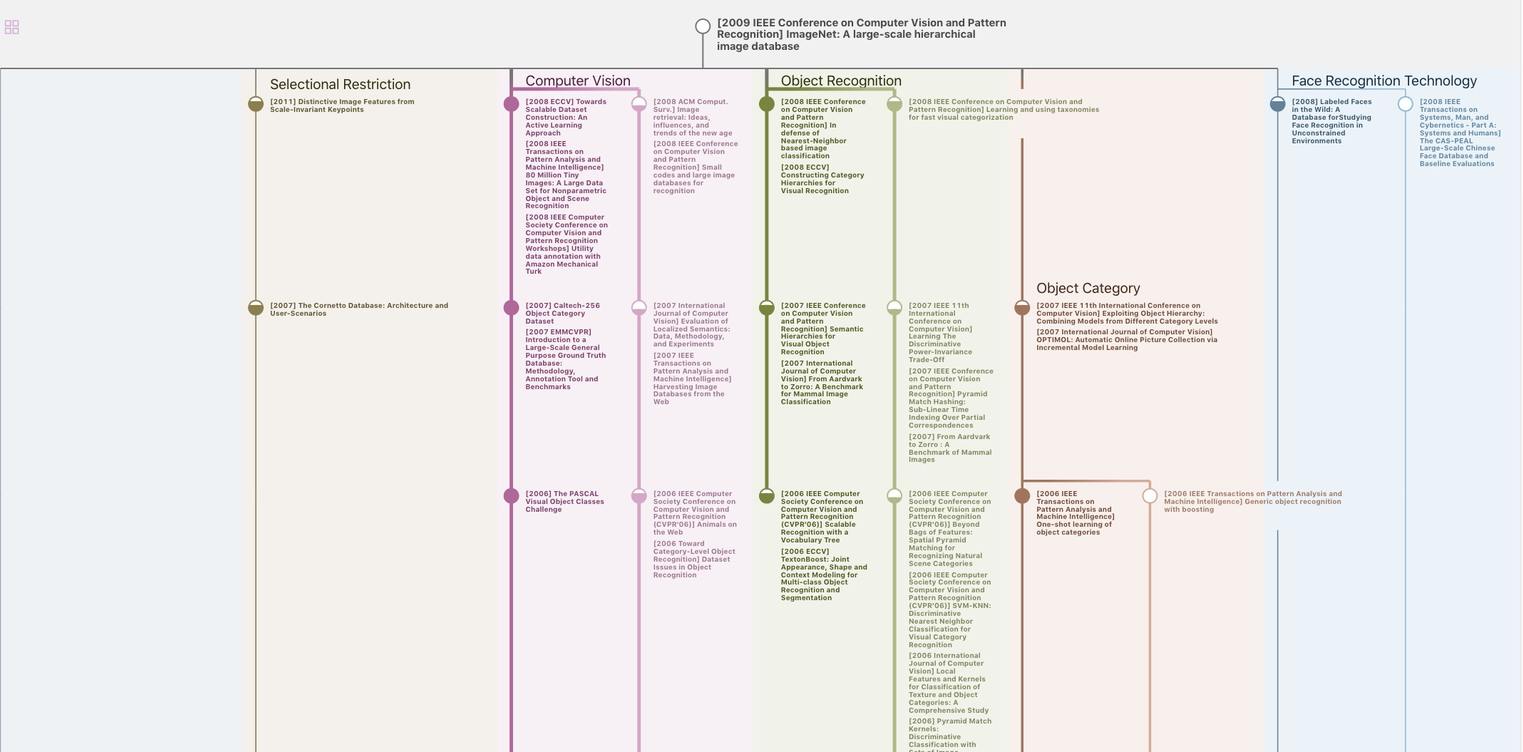
生成溯源树,研究论文发展脉络
Chat Paper
正在生成论文摘要