Region Attentive Action Unit Intensity Estimation With Uncertainty Weighted Multi-Task Learning
IEEE Transactions on Affective Computing(2023)
摘要
Facial action units (AUs) refer to a comprehensive set of atomic facial muscle movements. Recent works have focused on exploring complementary information by learning the relationships among AUs. Most existing approaches process AU co-occurrence and enhance AU recognition by learning the dependencies among AUs from labels, however, the complementary information among features of different AUs are ignored. Moreover, ground truth annotations suffer from a large intra-class variance and their associated intensity levels may vary depending on the annotators' experience. In this paper, we propose the Region Attentive AU intensity estimation method with Uncertainty Weighted Multi-task Learning (RA-UWML). A RoI-Net is first used to extract features from the pre-defined facial patches where the AUs locate. Then, we use the co-occurrence of AUs using both within patch and between patches representation learning. Within a given patch, we propose sharing representation learning in a multi-task manner. To achieve complementarity and avoid redundancy between different image patches, we propose to use a multi-head self-attention mechanism to adaptively and attentively encode each patch specific representation. Moreover, the AU intensity is represented as a Gaussian distribution, instead of a single value, where the mean value indicates the most likely AU intensity and the variance indicates the uncertainty of the estimated AU intensity. The estimated variances are leveraged to automatically weight the loss of each AU in the multitask learning model. In extensive experiments on the Disfa, Fera2015 and Feafa benchmarks, it is shown that the proposed AU intensity estimation model achieves better results compared to the state-of-the-art models.
更多查看译文
关键词
Action unit intensity,relation learning,multi-head self-attention,multi-task learning,data uncertainty
AI 理解论文
溯源树
样例
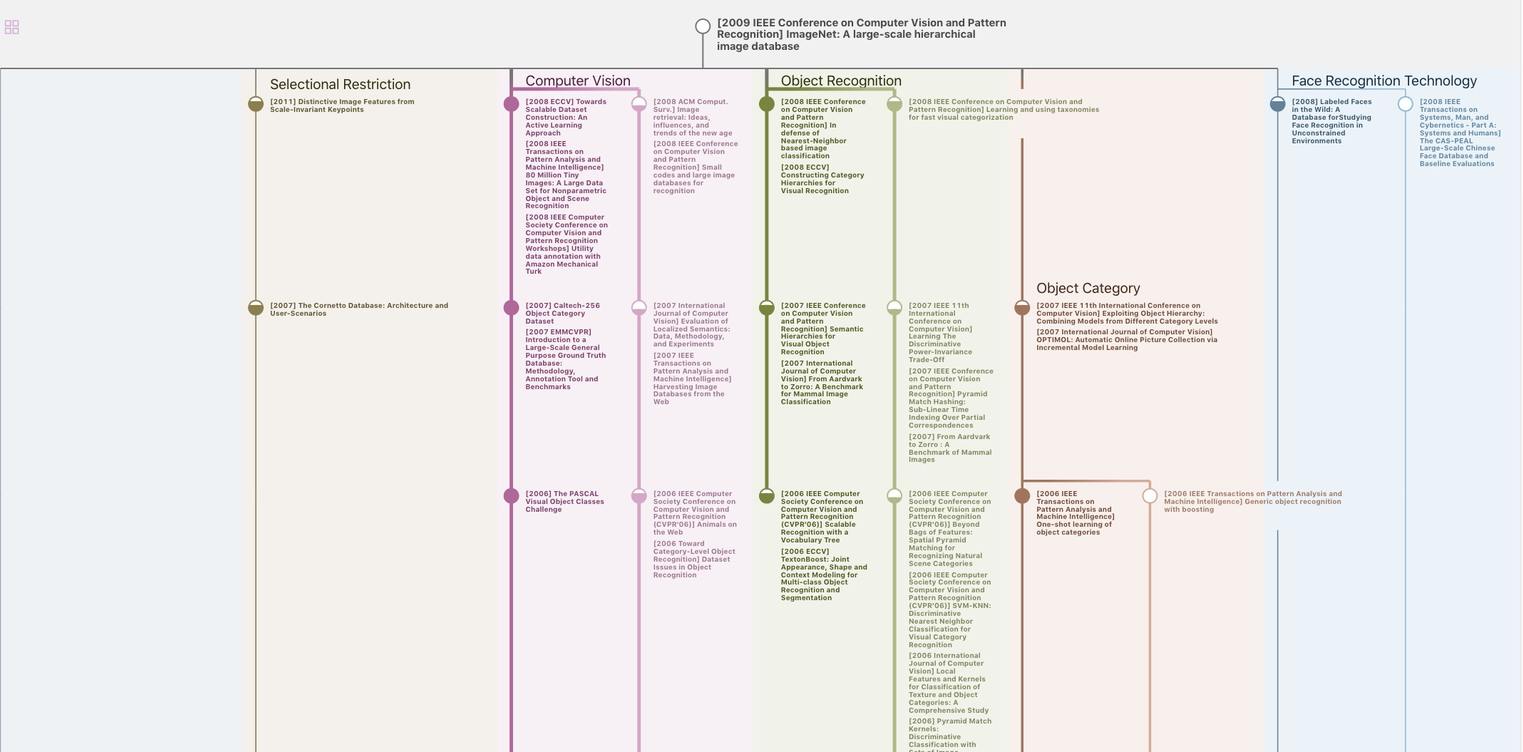
生成溯源树,研究论文发展脉络
Chat Paper
正在生成论文摘要