COVID-19 Surveillance Data and Models: Review and Analysis, Part 1
Social Science Research Network(2020)
摘要
BACKGROUND. Reliable COVID-19 data are critical for understanding the disease and spread of the pandemic, for decision-making, for developing and implementing public health measures, and for tracking the effectiveness of interventions. Currently, however, there is a confusing plethora of publicly available COVID-19 surveillance data resources. Relevant websites are frequently poorly designed making it extraordinarily time-consuming and frustrating to find and extract the relevant information.
METHODS. A systemic search of government, official agency, and non-government sources of COVID-19 surveillance and related data, computer code, and forecasting models was conducted.
RESULTS. A comprehensive compendium was built of COVID-19 surveillance data and models having worldwide national coverage, and some sources of particular interest having sub-national coverage. Hyperlinks are provided to download data or computer code from each of the resources. For each resource, a concise description of the data and metadata, including identification of the data sources used to compile the data is provided. The compendium is provided in the supplementary material, organized in nine tables: (1) COVID-19 surveillance datasets and sources; (2) Databases or catalogues of COVID-19 surveillance data; (3) Resources that provide a corpus of COVID-19 related text; (4) Resources that track COVID-19 government responses; (5) R code potentially useful for analysis of COVID-19 data; (6) COVID-19 related data analysis platforms; (7) COVID-19 models; (8) Useful visualizations of COVID-19 data that go beyond the usual ‘dashboards’; and, (9) Commercial sites that showcase their product with a COVID-19 use case. Selected examples of data resources and models are provided in two additional tables in the body of the text.
CONCLUSION. There is no single source of truth for COVID-19 surveillance data. Government and non-government data were found to be fragmented and difficult to find and use. There is a need to implement the principles of Open Science and FAIRER (Findable, Accessible, Interoperable, Reusable, Ethical, and Reproducible) data. There is an urgent need to develop a common standard for reporting communicable disease surveillance data without which Open Science and FAIRER data will be difficult to achieve.
更多查看译文
AI 理解论文
溯源树
样例
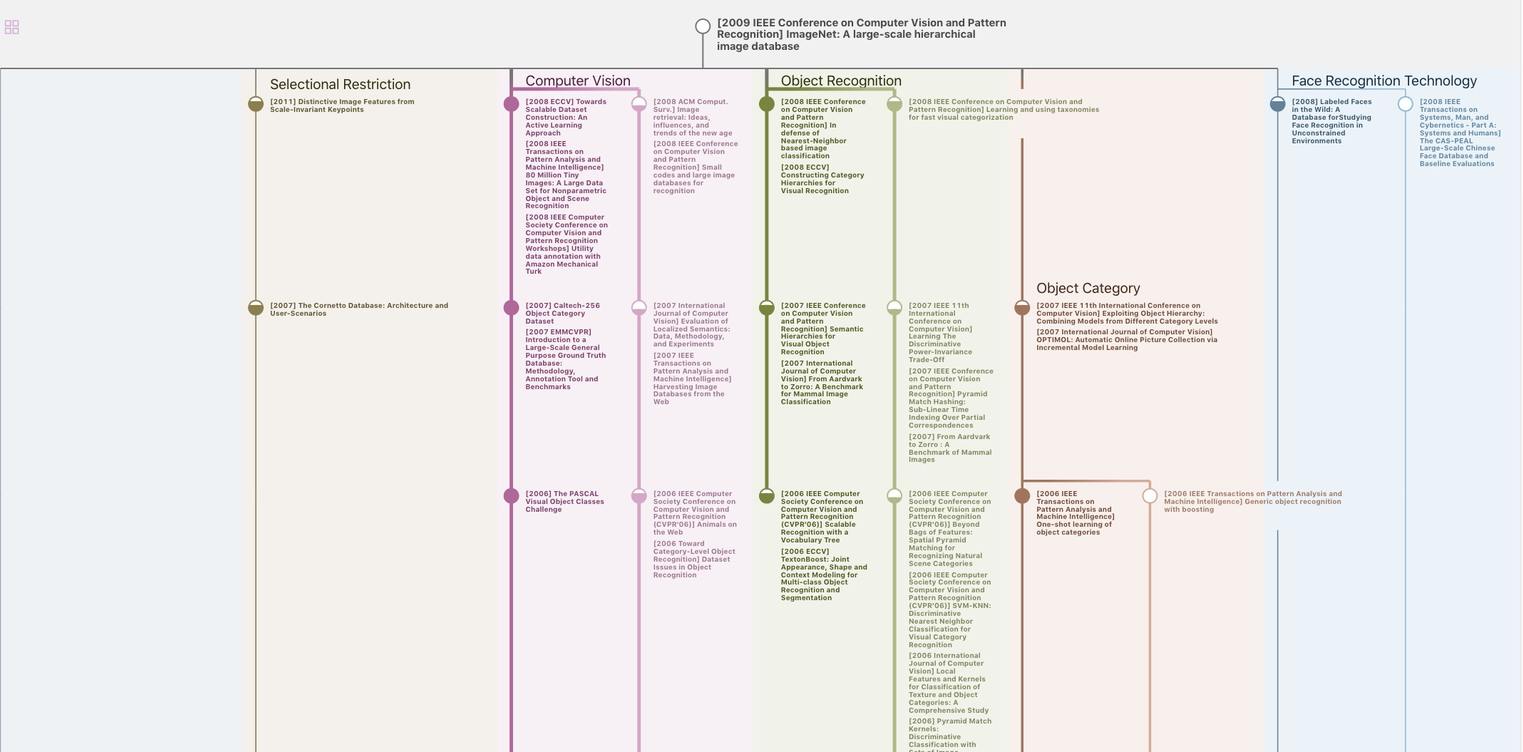
生成溯源树,研究论文发展脉络
Chat Paper
正在生成论文摘要