Objects Detection from High-Resolution Remote Sensing Imagery Using Training-Optimized YOLOv3 Network
Laser & Optoelectronics Progress(2021)
摘要
The traditional YOLOv3 model uses ImageNet and COCO datasets for training, in which the scene target characteristics are significantly different from those in test datasets, and leads to low detection accuracy of complex scene targets in high-resolution remote-sensing images. This paper optimizes the training process of the traditional YOLOv3 network using the idea of transfer learning. During the training of the YOLOv3 network, the model is pre-trained by generating an augmented dataset similar to the target domain. The training-optimized method improves the accuracy of the object boundary of target prediction. Also, the parameters of the pre-training model are fine-tuned using a training dataset from the target domain, thus, completing the whole training process of the network. The experiment on the detection of three types of object, including aircraft, playground, overpass, was carried out based on a subset of RSOD & DIOR dataset for remote sensing image object detection. The results show that the proposed YOLOv3 model effectively improves the detection accuracy of the three types of targets in complex urban scenes. The mean average precision of object detection using our model improved by 2 or more, compared with the traditional YOLOv3 model.
更多查看译文
关键词
remote sensing, object detection, high-resolution remote-sensing image, YOLOv3 network, transfer learning
AI 理解论文
溯源树
样例
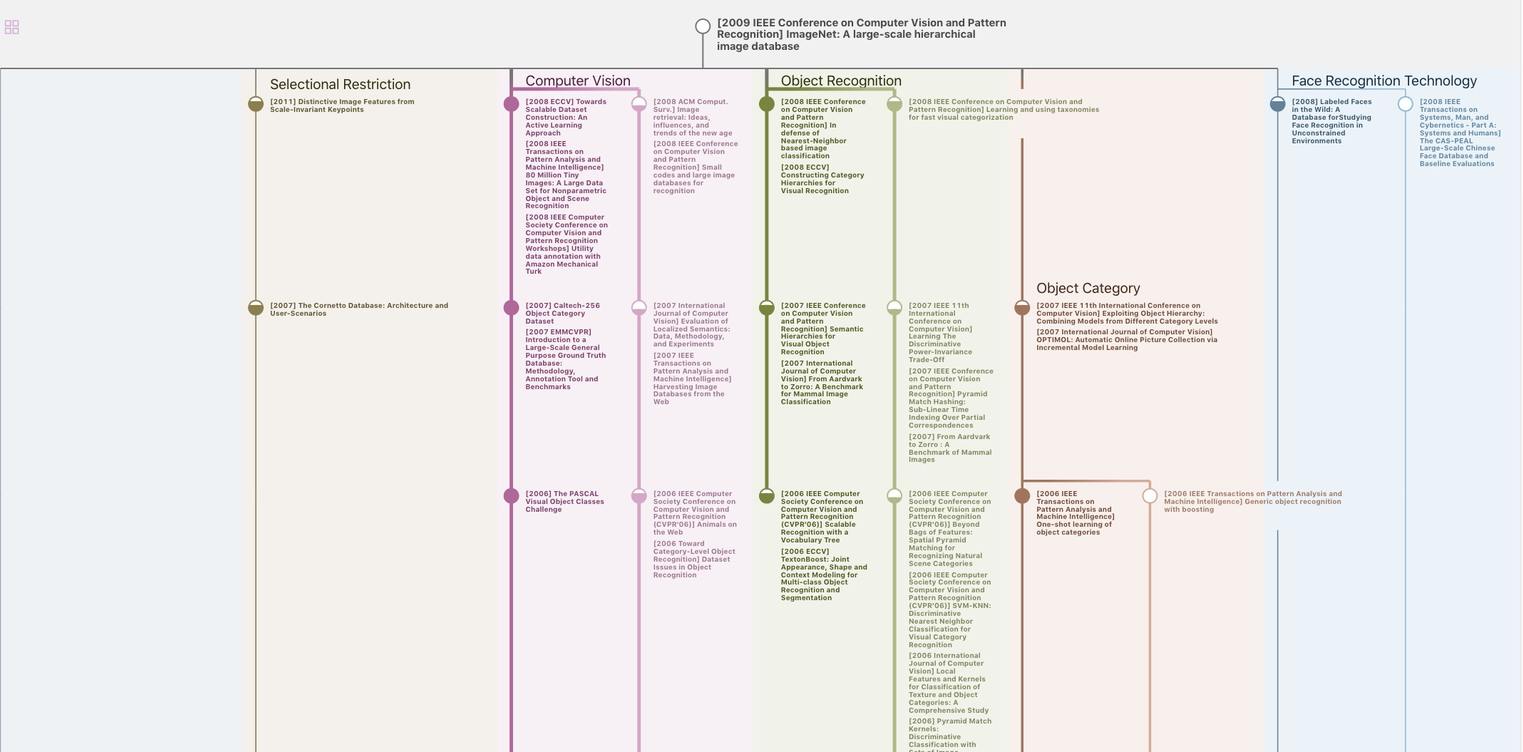
生成溯源树,研究论文发展脉络
Chat Paper
正在生成论文摘要