87. Development of a machine learning algorithm for neck motion classification in patients after anterior cervical discectomy and fusion (ACDF)
The Spine Journal(2021)
摘要
BACKGROUND CONTEXT Loss of cervical range of motion (ROM) is a common complication after anterior cervical discectomy and fusion (ACDF). Recovery of cervical ROM is a goal for postoperative ACDF patients and is achieved through prescribed at-home neck exercises and physical therapy. Currently, cervical ROM is assessed periodically via physical examination by trained health care professionals. These methods, however, are incapable of continuous measurement of neck motion in a patient's naturalist environment. A skin-mounted, wireless, and wearable sensor that offers a noninvasive way to continuously monitor neck motion as well as heart rate, respiratory rate, energy expenditure, swallow count, and body orientation would hold significant clinical utility by tracking recovery, ensuring adherence to physical therapy, and providing warning of potentially deleterious excessive motion during the essential postoperative period. PURPOSE Develop and validate in healthy normal subjects a machine learning algorithm to classify 8 neck motions: right rotation, left rotation, right lateral bending, left lateral bending, flexion, extension, retraction, protraction Validate the algorithm in postoperative ACDF patients Evaluate clinician and patient usability of the sensor. METHODS On 11 healthy subjects (6 females, 5 males), the sensor was placed at the suprasternal notch, centered between the sternocleidomastoid tendons. Ten four-second samples of each of the 8 motions were collected from each subject (880 total neck motion samples). Each sample consisted of the output from the sensor's 3-axis accelerometer and gyroscope: x, y, and z linear accelerations and angular velocities. A convolutional neural network (CNN) model was developed using Python to perform 8-way classification using a step-wise classification scheme. A leave-one-out training/testing scheme was used in which a model was trained on 10 subjects and then tested on the remaining subject, which was iterated across all 11 subjects. Additionally, a random mixing training/testing scheme was used, which involved random mixing of all 880 samples, training on 80% of the mixed samples, and testing on the remaining 20%. Ongoing activities include direct measurement of neck motion in the ACDF population. RESULTS Each motion produced a characteristic pattern of linear accelerations and angular velocities, which provided the features that were used for classification. The leave-one-out training/testing scheme yielded a mean accuracy of 84% (+/-12%), ranging from 57 to 94%. Ten trials of the random mixing training/testing scheme yielded a mean accuracy of 91% (+/- 1.0%). Flexion vs retraction and extension vs protraction were the most difficult signals to distinguish. CONCLUSIONS Rotation, lateral bending, flexion, extension, retraction, and protraction produce unique motion patterns that can be measured with a wearable sensor containing a 3-axis accelerometer and gyroscope placed at the suprasternal notch. Rotation, lateral bending, flexion, and extension produced the most unique motion signatures. Linear accelerations and angular velocities, measured by accelerometer and gyroscope, respectively, can be strategically selected to perform a step-wise 8-way classification of these neck motions with relatively high accuracy. Validation of the algorithm in the surgical patient population, which is ongoing, could demonstrate the utility of wearable sensors in the ACDF patient population and significantly improve their quality of postoperative care. FDA DEVICE/DRUG STATUS This abstract does not discuss or include any applicable devices or drugs.
更多查看译文
AI 理解论文
溯源树
样例
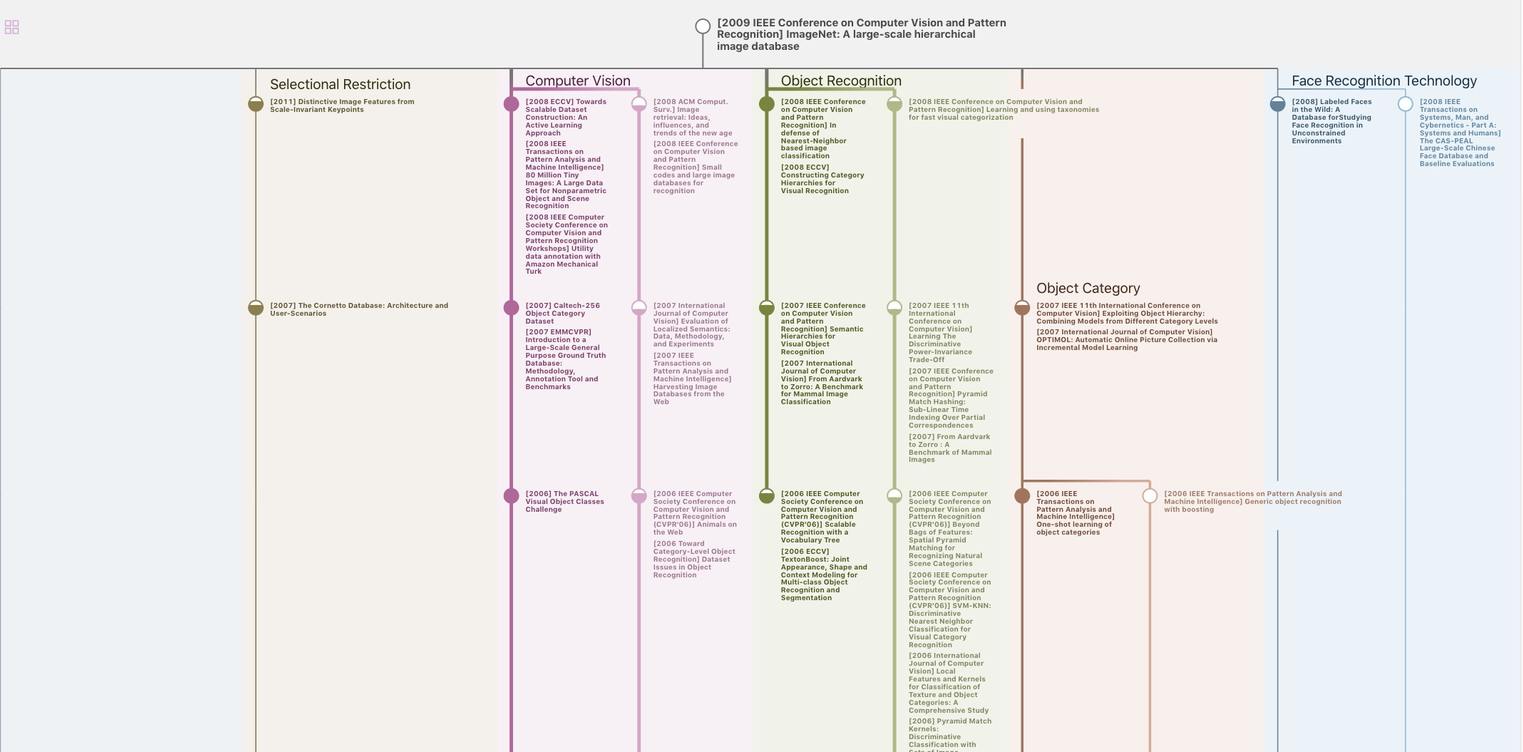
生成溯源树,研究论文发展脉络
Chat Paper
正在生成论文摘要