Data-driven Clustering and Feature-based Retail Electricity Pricing with Smart Meters
Social Science Research Network(2020)
摘要
We consider an electric utility company that serves retail electricity customers over a discrete-time horizon. In each period, the company observes the customers' consumption as well as high-dimensional features on customer characteristics and exogenous factors. These features exhibit three types of heterogeneity—over time, customers, or both. Based on the consumption and feature observations, the company can dynamically adjust the retail electricity price at the customer level. The consumption depends on the features: there is an underlying structure of clusters in the feature space, and the relationship between consumption and features is different in each cluster. Initially, the company knows neither the underlying cluster structure nor the corresponding consumption models. We measure the company's performance by its average regret, i.e., the profit loss per period per customer, relative to a clairvoyant who knows the underlying cluster structure and the consumption model in each cluster. We design a data-driven policy of joint spectral clustering and feature-based pricing, and show that its average regret converges to zero at the fastest achievable rate. We conduct case studies based on real-life smart meter data from Texas and simulation experiments. Relative to the company policy, our policy increases company profits by 146% over a three-month period.
更多查看译文
AI 理解论文
溯源树
样例
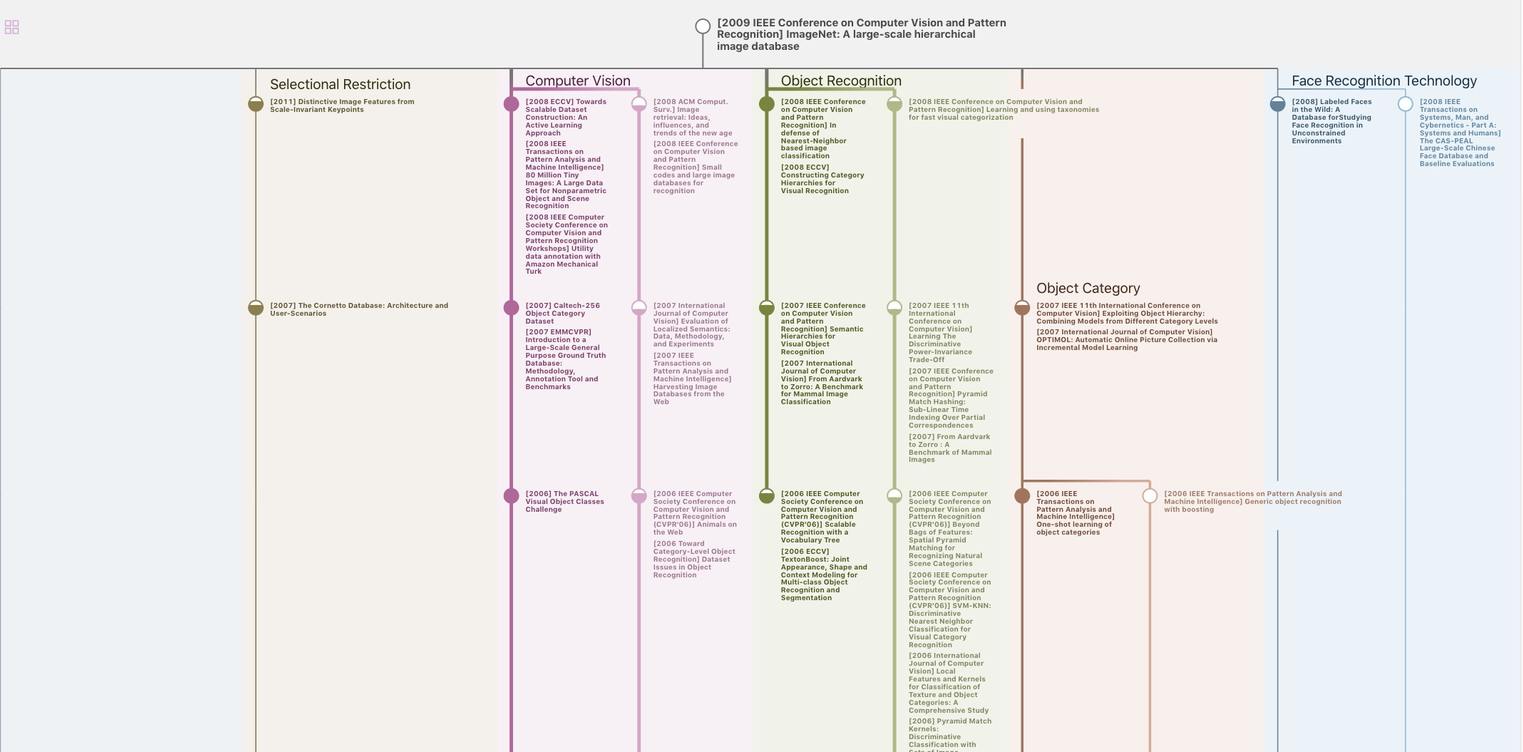
生成溯源树,研究论文发展脉络
Chat Paper
正在生成论文摘要