Enhanced Innovized Progress Operator for Evolutionary Multi- and Many-Objective Optimization
IEEE Transactions on Evolutionary Computation(2022)
摘要
Innovization is a task of learning common relationships among some or all of the Pareto-optimal (PO) solutions in multi- and many-objective optimization problems. A recent study has shown that a chronological sequence of nondominated solutions obtained along the successive generations of an optimizer possesses salient patterns that can be learnt using a Machine Learning (ML) model, and can help the offspring solutions progress in useful directions. This article enhances each constitutive module of the above approach, including novel interventions on management of the convergence-diversity tradeoff while mapping the solutions from the previous and current generation; use of a computationally more efficient ML method, namely, Random Forest (RF); and changing the manner and extent to which the learnt ML model is utilized toward advancement of the offspring. The proposed modules constitute what is called the enhanced innovized progress (IP2) operator. To investigate the search efficacy provided by the IP2 operator, it is integrated with multi-and many-objective optimization algorithms, such as NSGA-II, NSGA-III, MOEA/D, and MaOEA-IGD, and tested on a range of two- to ten-objective test problems, and five real-world problems. Since the IP2 operator utilizes the history of gradual and progressive improvements in solutions over generations, without requiring any additional solution evaluations, it opens up a new direction for ML-assisted evolutionary optimization.
更多查看译文
关键词
Innovization,Innovized Progress (IP),learning-assisted optimization,machine learning (ML),multiobjective optimization,online Innovization
AI 理解论文
溯源树
样例
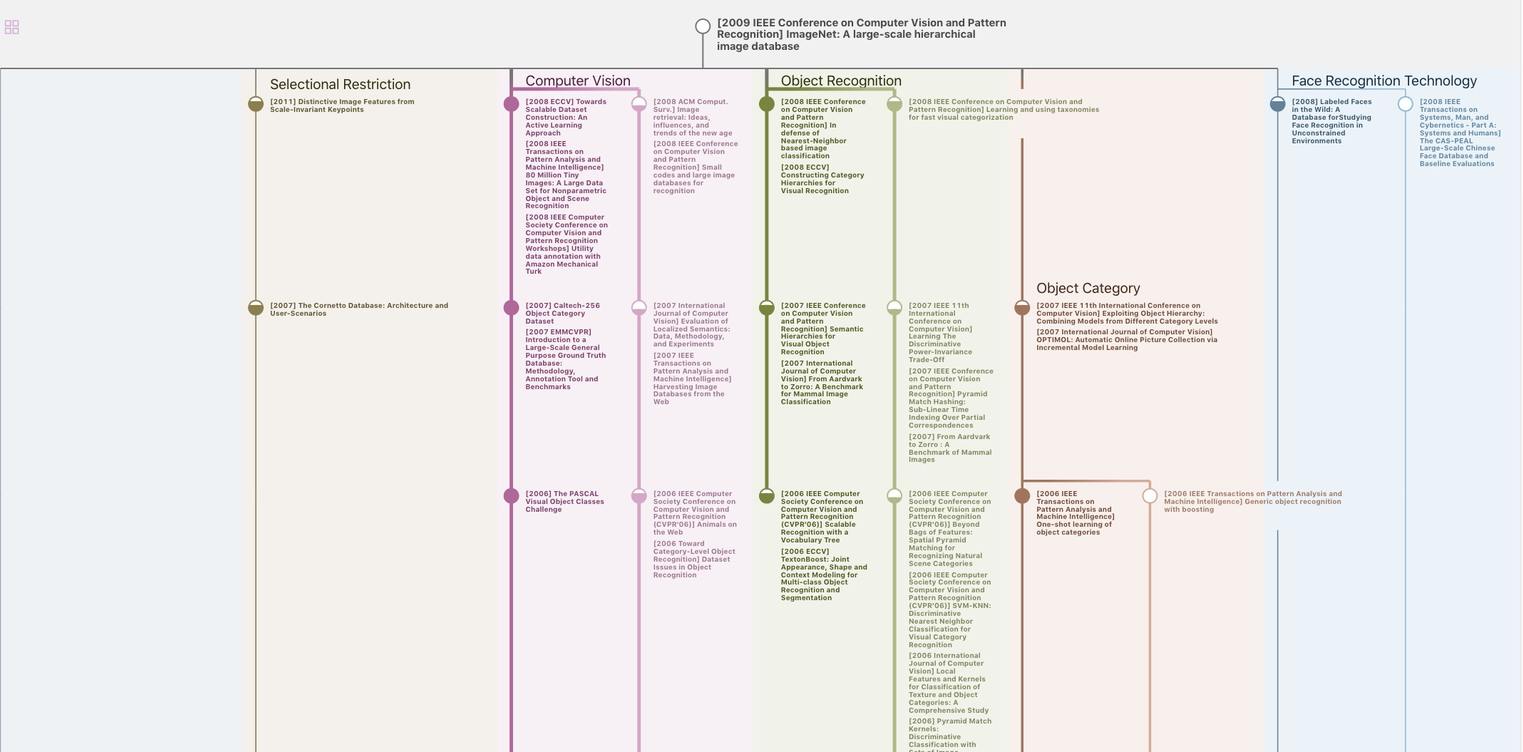
生成溯源树,研究论文发展脉络
Chat Paper
正在生成论文摘要