Spherical DNNs and Their Applications in 360 $^\circ$ Images and Videos
IEEE Transactions on Pattern Analysis and Machine Intelligence(2021)
摘要
Spherical images or videos, as typical non-Euclidean data, are usually stored in the form of 2D panoramas obtained through an equirectangular projection, which is neither equal area nor conformal. The distortion caused by the projection limits the performance of vanilla Deep Neural Networks (DNNs) designed for traditional Euclidean data. In this paper, we design a novel Spherical Deep Neural Network (DNN) to deal with the distortion caused by the equirectangular projection. Specifically, we customize a set of components, including a spherical convolution, a spherical pooling, a spherical ConvLSTM cell and a spherical MSE loss, as the replacements of their counterparts in vanilla DNNs for spherical data. The core idea is to change the identical behavior of the conventional operations in vanilla DNNs across different feature patches so that they will be adjusted to the distortion caused by the variance of sampling rate among different feature patches. We demonstrate the effectiveness of our Spherical DNNs for saliency detection and gaze estimation in 360° videos. To facilitate the study of the 360 video saliency detection, we further construct a large-scale 360° video saliency detection dataset. Comprehensive experiments validate the effectiveness of our proposed Spherical DNNs for spherical handwritten digit classification and sport classification, saliency detection and gaze tracking in 360° videos.
更多查看译文
AI 理解论文
溯源树
样例
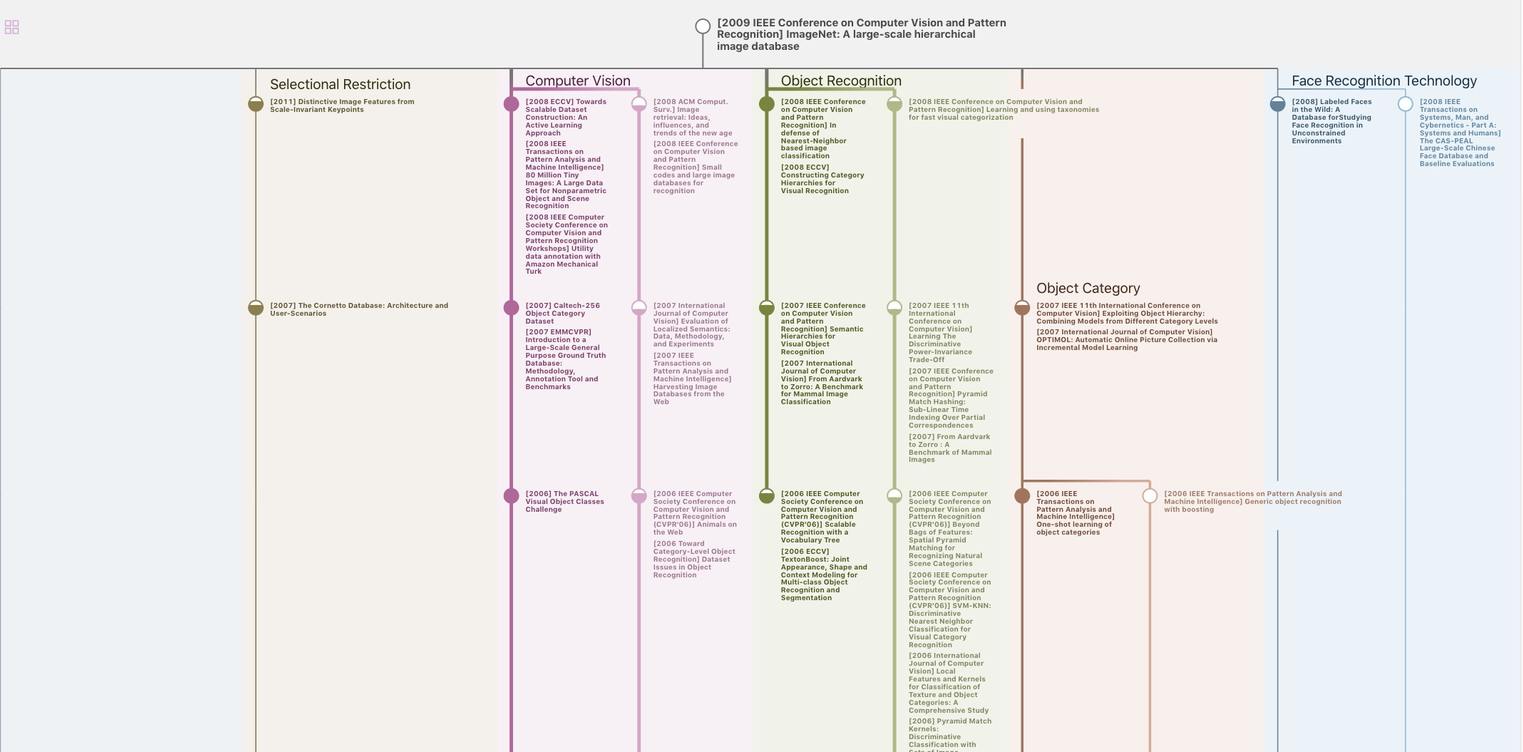
生成溯源树,研究论文发展脉络
Chat Paper
正在生成论文摘要