Making Predictions for Sequentially Available Choices: A Semiparametric Gaussian Copula Approach (SGC)
Social Science Research Network(2021)
摘要
Today’s consumers often encounter choices that are available sequentially and that expire quickly, such as when using an online dating app or when shopping for sales of online streaming services and daily deals. In such situations, consumers make decisions while facing uncertainty about future alternatives. Predictions of consumer behavior in these situations must overcome two main challenges: (1) imbalanced data, which arise when observations are disproportionately distributed across different decision categories, and (2) difficulties in combining the numerous variables from different distributions, which are needed for effective prediction. We develop a semiparametric Gaussian Copula (SGC) approach that predicts consumer decisions for sequentially available choices. The SGC model is grounded in the search literature, and predictions are made using a copula-based approach. Specifically, we leverage the means of semiparametric Gaussian copulas to generate a complex joint distribution encompassing all variables. Consumer choice predictions are then derived based on each individual consumer’s likelihood of making that choice and calculated using the conditional posterior of the joint distribution. As our model is semiparametric in nature and its predictions are based on choice likelihood, it has strong predictive performance when applied to imbalanced data. We apply our model to a proprietary dataset that comprises customers’ clickstream data from a daily deal platform. The results demonstrate the superior performance of our model by comparing it with other prediction models widely used in similar scenarios. Our model can advance companies’ efforts by targeting the right customers with the right product at the right time.
更多查看译文
AI 理解论文
溯源树
样例
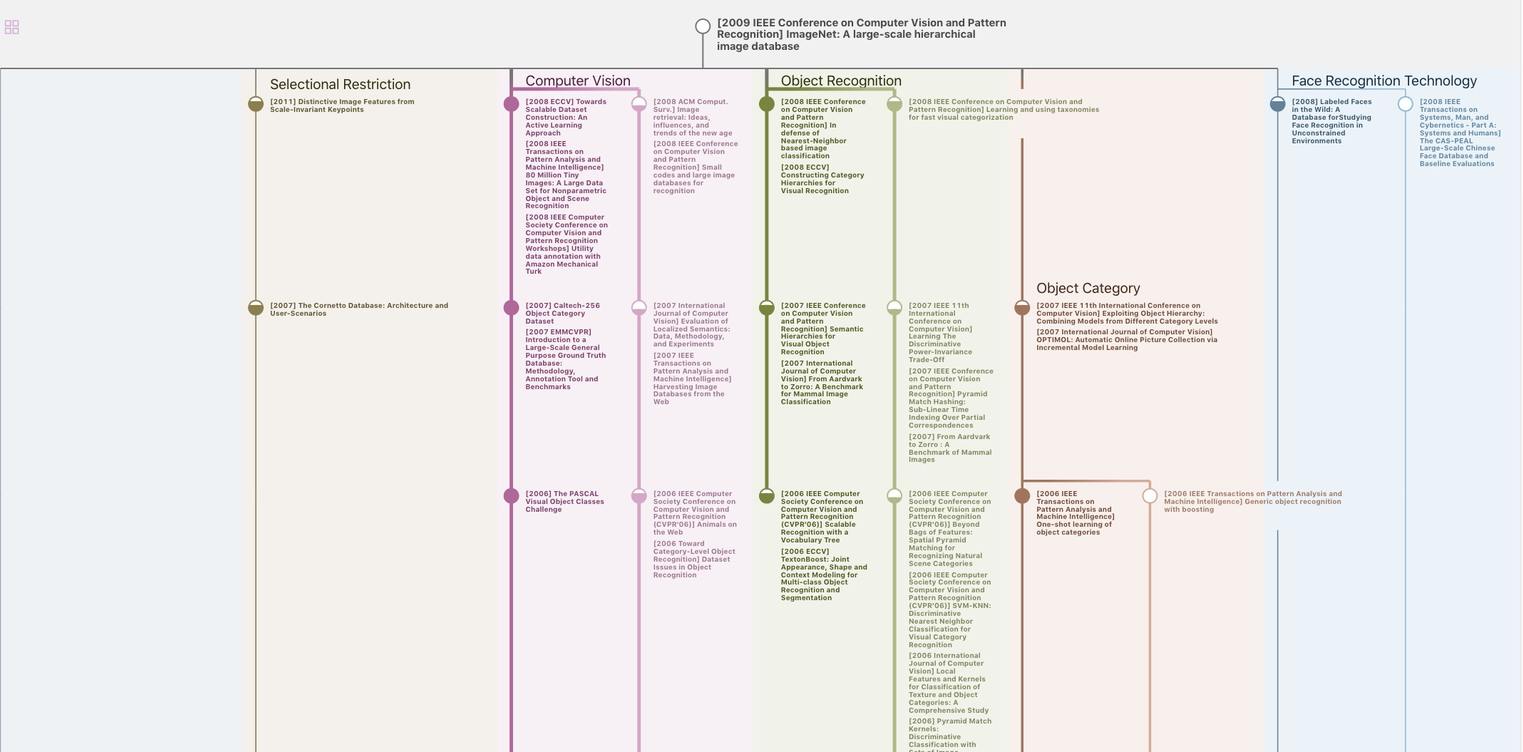
生成溯源树,研究论文发展脉络
Chat Paper
正在生成论文摘要