Assessing Adaptive Irrigation Impacts on Water Scarcity in Nonstationary Environments-A Multi-Agent Reinforcement Learning Approach
WATER RESOURCES RESEARCH(2021)
摘要
One major challenge in water resource management is to balance the uncertain and nonstationary water demands and supplies caused by the changing anthropogenic and hydroclimate conditions. To address this issue, we developed a reinforcement learning agent-based modeling (RL-ABM) framework where agents (agriculture water users) are able to learn and adjust water demands based on their interactions with the water systems. The intelligent agents are created by a reinforcement learning algorithm adapted from the Q-learning algorithm. We illustrated this framework in a case study where the RL-ABM is two-way coupled with the Colorado River Simulation System (CRSS), a long-term planning model used for the administration of the Colorado River Basin, for assessing agriculture water uses impacts on water scarcity. Seventy-eight intelligent agents are simulated, which can be grouped into three categories based on their parameter values: the "aggressive" (swift actions; low regrets), the "forward-looking conservative" (mild actions; high regrets; fast learning), and the "myopic conservative" (mild actions; median regrets; slow learning). The ABM-CRSS results showed that the major reservoirs in the Upper Colorado Basin might experience more frequent water shortages due to the increasing water uses compared to the original CRSS results. If the drought continues, the case study also demonstrates that agents can learn and adjust their demands.
更多查看译文
关键词
coupled human-nature systems,agriculture water demands,machine learning,water resources,Colorado River Basin
AI 理解论文
溯源树
样例
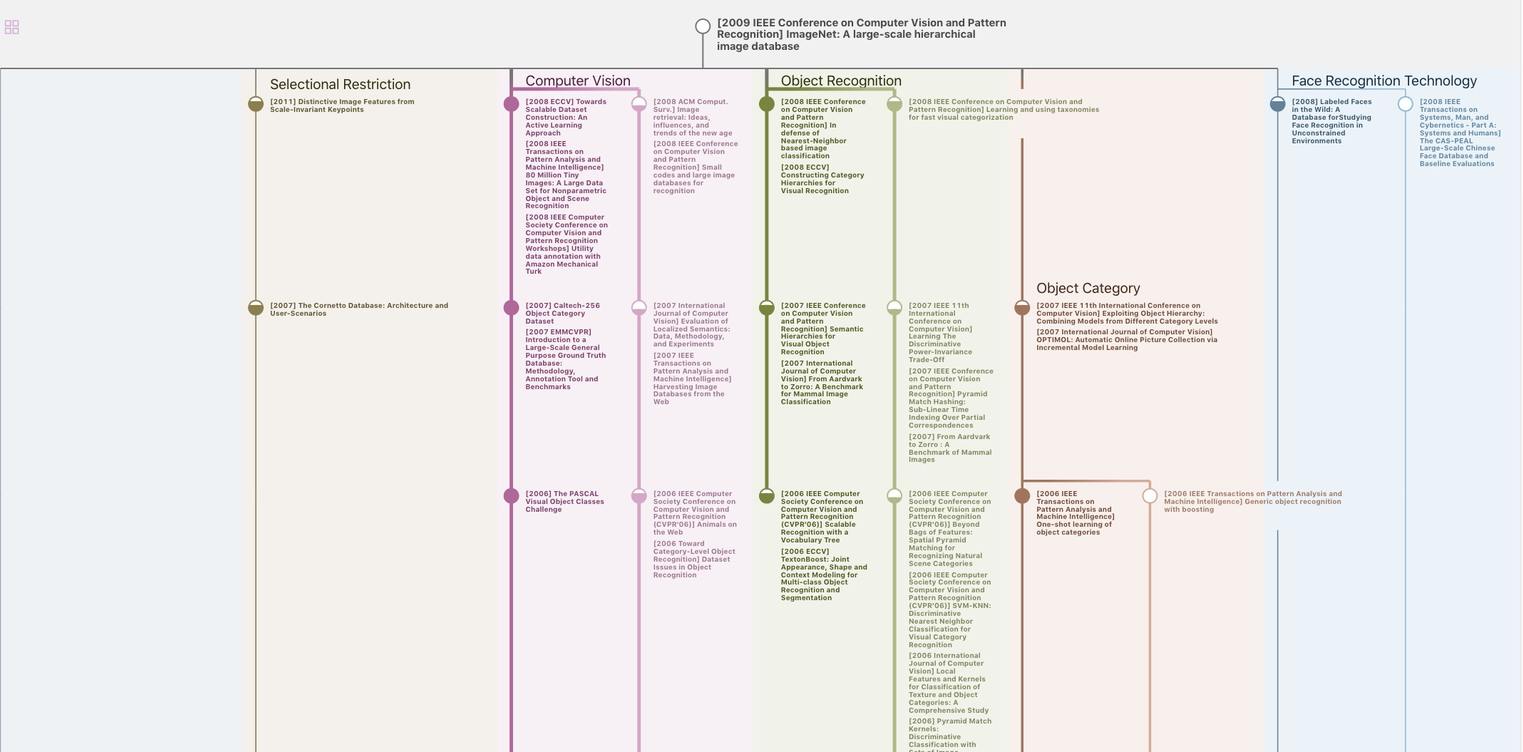
生成溯源树,研究论文发展脉络
Chat Paper
正在生成论文摘要