Detecting Multilayer Clouds From the Geostationary Advanced Himawari Imager Using Machine Learning Techniques
IEEE Transactions on Geoscience and Remote Sensing(2022)
摘要
This study develops a machine learning (ML)-based multilayer cloud detection algorithm for the passive Advanced Himawari Imager (AHI) aboard the geostationary Himawari-8 satellite. AHI measurements in the 0.64-, 1.6-, 2.3-, 3.9-, 7.3-, 8.6-, 11.2-, and 12.4-
$\mu \text{m}$
channels, their combinations, geolocations, and observational geometries are used as predictors, and collocated active CloudSat and CALIPSO data are used to accurately label multilayer cloud pixels as the reference/truth of the predictand. We develop an ML-based daytime model (ML-Day) that utilizes all the aforementioned predictors and an all-time one (ML-All) that excludes the solar channel-dependent variables. Among four ML algorithms, the random forest (RF) performs slightly better than the artificial neural network, K-nearest neighbor, and support vector machines. By comparing with the merged CloudSat and CALIPSO product, the ML-Day model correctly identifies ~89% single-layer clouds and ~70% multilayer clouds, outperforming the Moderate Resolution Imaging Spectroradiometer (MODIS) operational multilayer cloud product (~80% and ~40% given by Marchant
et al.
). The success rates of ML-All for single-layer and multilayer clouds also reach ~85% and ~64%, respectively. The misclassification of our algorithm is mostly caused by missing optically thin clouds, a drawback of most radiometers without the 1.38-
$\mu \text{m}$
channel. Furthermore, with multilayer cloud pixels well detected by our algorithm, the AHI operational cloud top height retrievals are found to be larger biased due to multilayer cloud occurrence and might be improved by considering cloud vertical structures.
更多查看译文
关键词
Himawari-8,machine learning (ML) techniques,multilayer cloud,passive radiometer
AI 理解论文
溯源树
样例
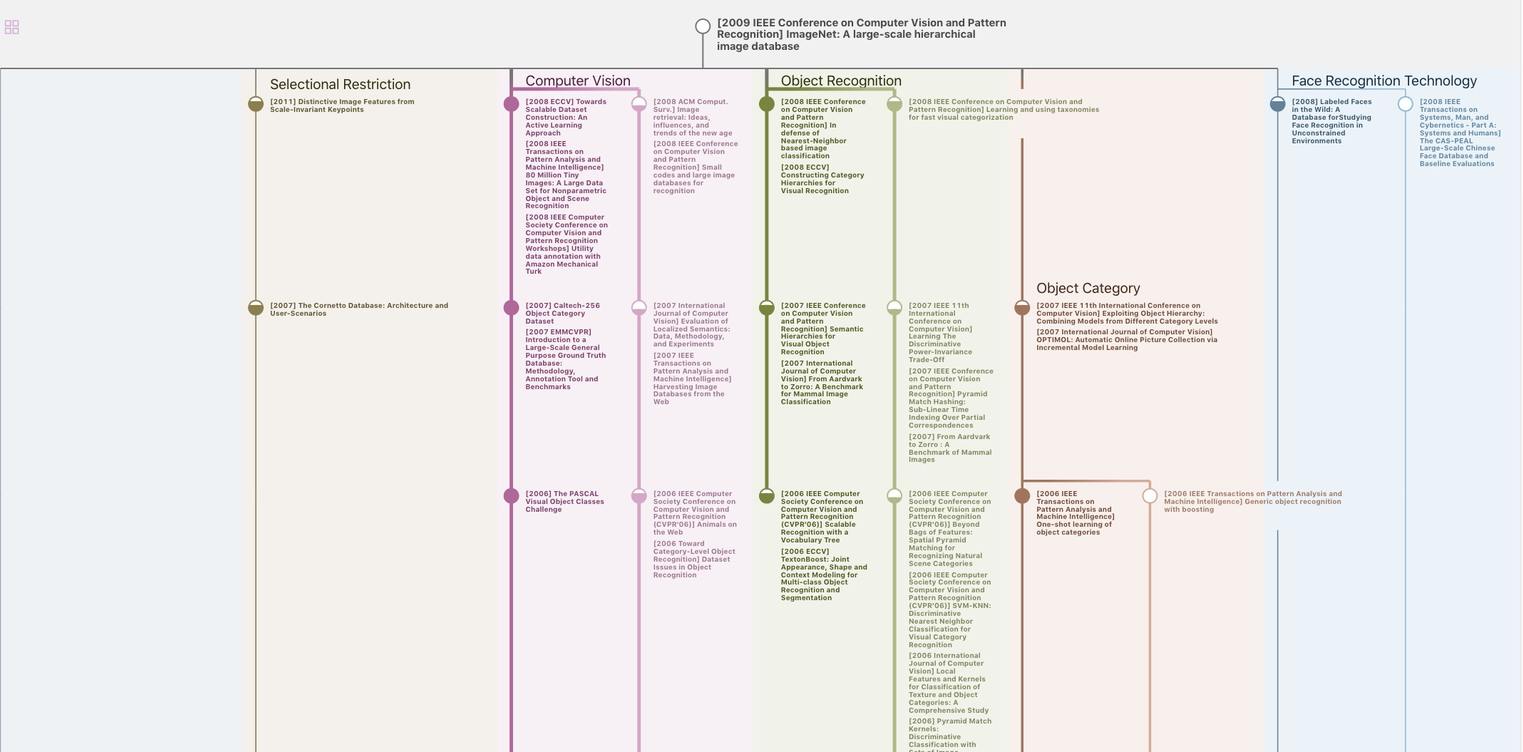
生成溯源树,研究论文发展脉络
Chat Paper
正在生成论文摘要