Strategy of hybrid optimization algorithms for source parameters estimation of hazardous gas in field cases.
GIS(2019)
摘要
ABSTRACTHazardous gas in chemical parks is an important factor affecting air quality. Its emission and leak accidents have gradually attracted people's attention. The emission and leak accidents are essentially air contaminant dispersion (ADS) and inverse traceability issues. Many ADS modeling methods have been developed by researchers. However, traditional ADS models are difficult to meet the requirement of both accuracy and efficiency. Due to the powerful fitting ability and fast calculation speed, artificial neural network (ANN) is used by more and more people for gas dispersion prediction. Of course, ANN training requires a large amount of data to ensure that the neural network can be applied to different scenarios. The Project Prairie Grass dataset provides us with plenty of actual experimental data to train neural network. The inverse traceability issue, which also known as source parameters estimation problem, has been solved by heuristic optimization algorithms in recent years. However, single optimization algorithm often has obvious advantages and disadvantages, and cannot balance local optimization and global optimization. Therefore, this paper explores the hybrid strategy between different optimization algorithms, and then combines single particle swarm optimization (PSO) algorithm with genetic algorithm (GA) and simulated annealing algorithm (SA) under the guidance of hybrid strategy. Finally, comparing single PSO and two hybrid optimization algorithms under unified standards, the results proves the superiority of hybrid optimization algorithms.
更多查看译文
AI 理解论文
溯源树
样例
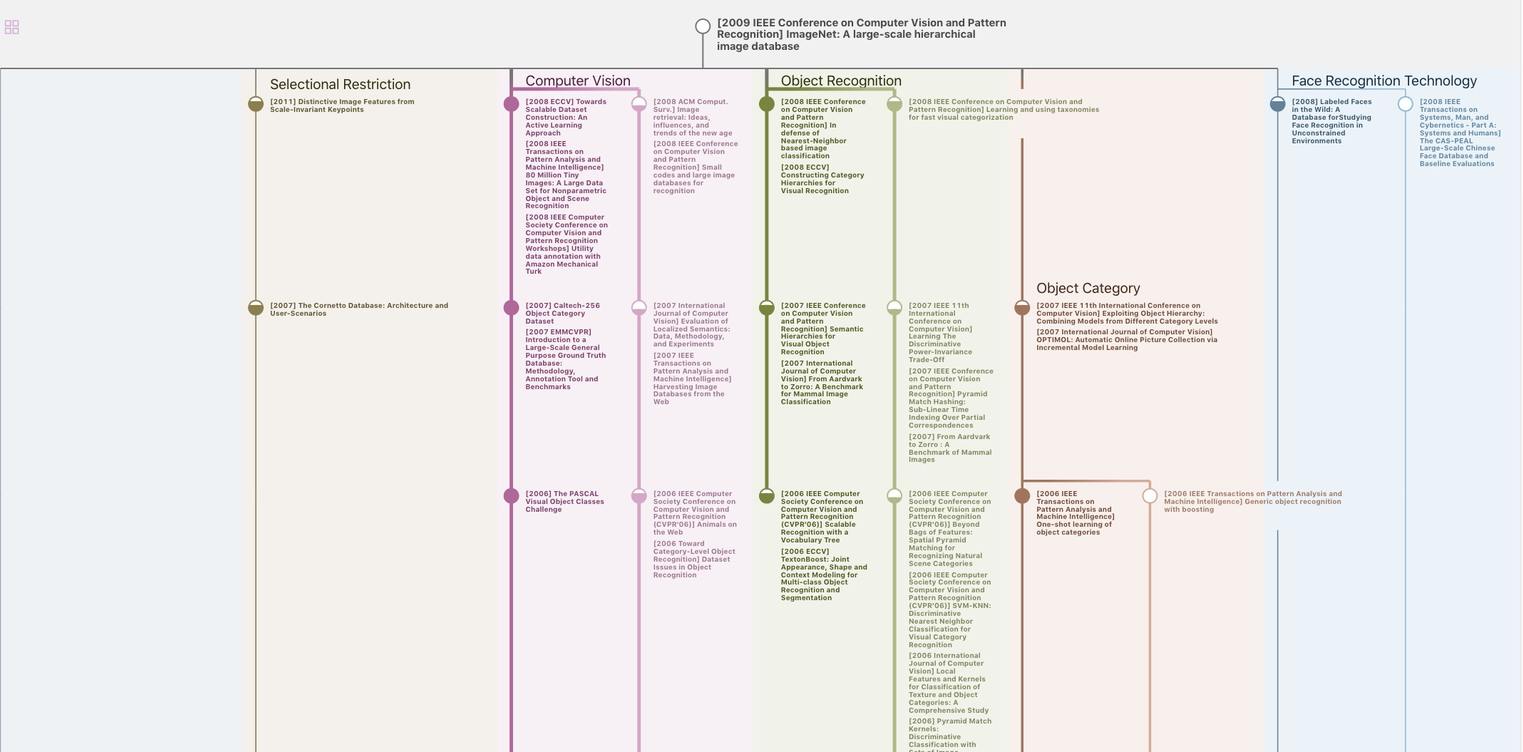
生成溯源树,研究论文发展脉络
Chat Paper
正在生成论文摘要