On Learning Contrastive Representations for Learning with Noisy Labels.
IEEE Conference on Computer Vision and Pattern Recognition(2022)
摘要
Deep neural networks are able to memorize noisy labels easily with a softmax cross-entropy (CE) loss. Previous studies attempted to address this issue focus on incorporating a noise-robust loss function to the CE loss. However, the memorization issue is alleviated but still remains due to the non-robust CE loss. To address this issue, we focus on learning robust contrastive representations of data on which the classifier is hard to memorize the label noise under the CE loss. We propose a novel contrastive regularization function to learn such representations over noisy data where label noise does not dominate the representation learning. By theoretically investigating the representations induced by the proposed regularization function, we reveal that the learned representations keep information related to true labels and discard information related to corrupted labels. Moreover, our theoretical results also indicate that the learned representations are robust to the label noise. The effectiveness of this method is demonstrated with experiments on benchmark datasets.
更多查看译文
关键词
Representation learning, Deep learning architectures and techniques, Machine learning, Transparency,fairness,accountability,privacy and ethics in vision
AI 理解论文
溯源树
样例
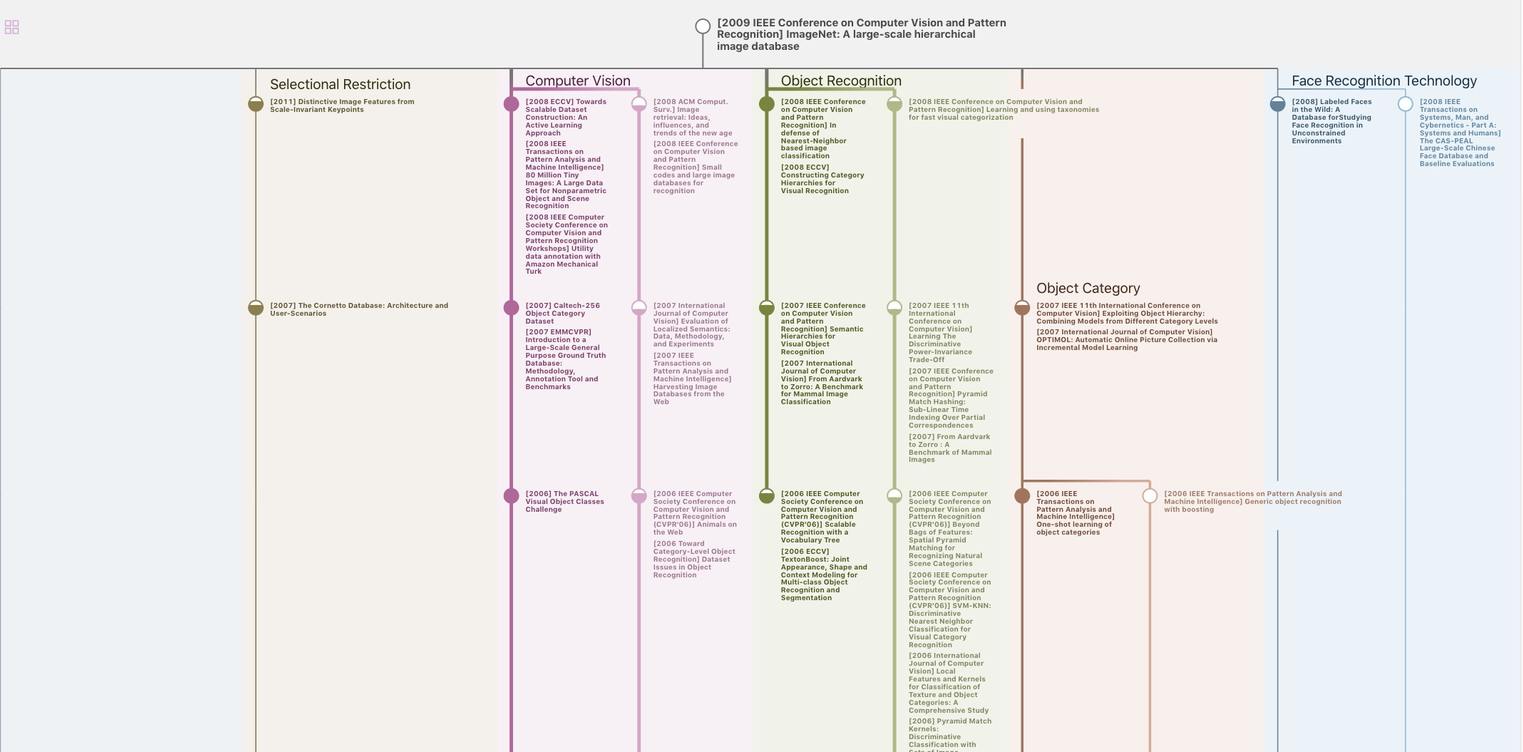
生成溯源树,研究论文发展脉络
Chat Paper
正在生成论文摘要