Design of Resolution-Tunable Neural Network-Based Integrated Reconstructive Spectrometer
IEEE Sensors Journal(2022)
摘要
Optical Spectroscopy is one of the most ubiquitous techniques found in academic research and industrial processes. Reconstructive spectroscopy is a new paradigm of spectroscopy where the reconstruction problem is modelled as an ill-posed inverse problem. Hence, we employ certain algorithms to solve such ill-posed inverse problems. This spectrometer paradigm helps miniaturize such optical spectrometers, which otherwise would have used bulky and expensive dispersive optical setup. In this work, we present a design of an on-chip reconstructive spectrometer for visible light using a spectrally selective silicon photodetector array. The spectral reconstruction is carried out using a set of two neural networks. Using different neural networks (one for broadband and another for narrowband) with the same hardware setup, we show the spectral reconstruction of broadband sources in the wavelength range of 400 nm–900 nm with a resolution of 28 nm and a laser source in the wavelength range of 780 nm–785 nm with a resolution of 0.6 nm. Hence, we demonstrate that a higher resolution can be achieved when the reconstructive neural network is trained on a smaller dynamic range.
更多查看译文
关键词
Computational spectroscopy,neural network,on-chip spectroscopy,photonic crystal,reconstructive spectroscopy
AI 理解论文
溯源树
样例
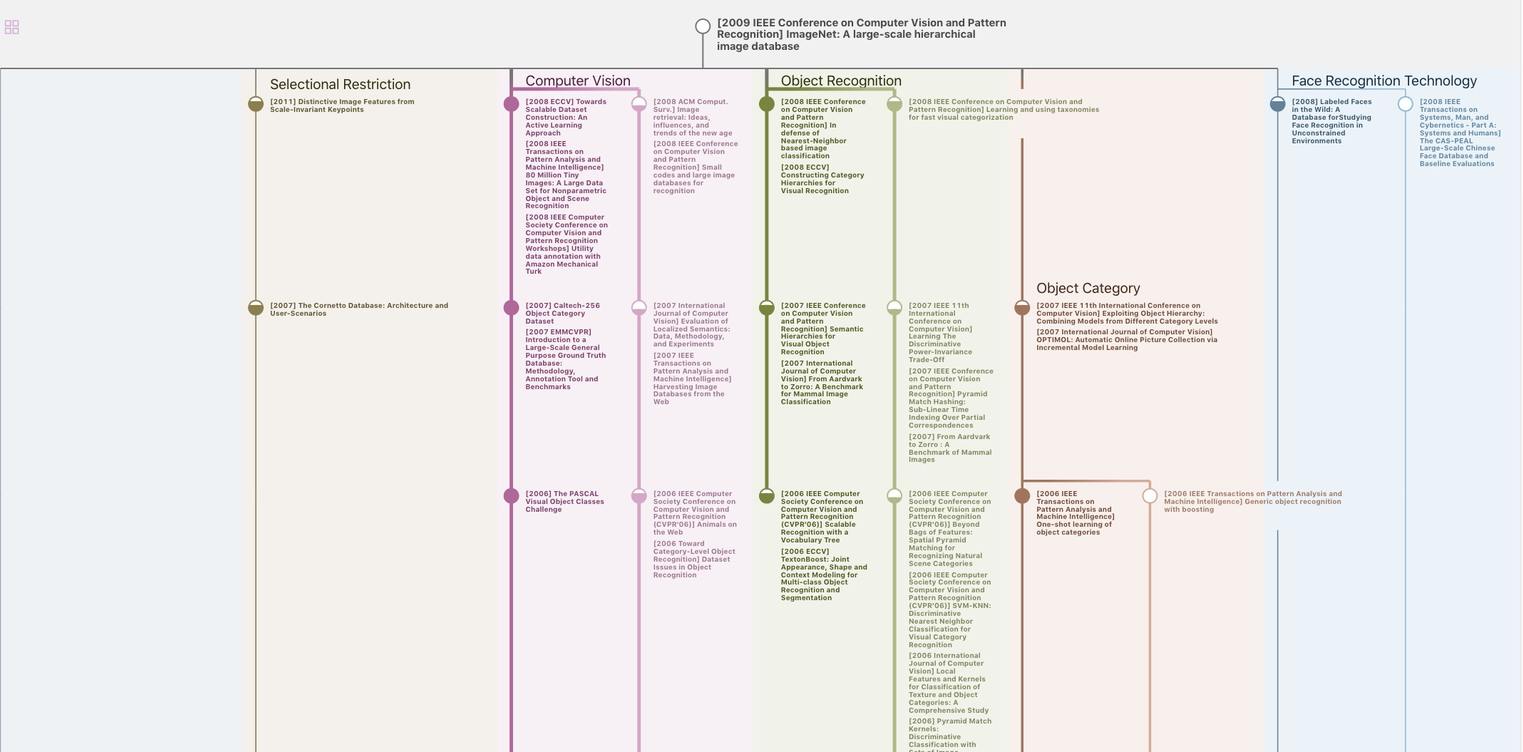
生成溯源树,研究论文发展脉络
Chat Paper
正在生成论文摘要