Can a Reinforcement Learning Trading Agent Beat Zero Intelligence Plus at Its Own Game?
Studies in computational intelligence(2021)
摘要
We develop a simple trading agent that extends Cliff’s Zero Intelligence Plus (ZIP) strategy for trading in continuous double auctions. The ZIP strategy makes trading decisions using a deterministic hand-crafted and fixed mapping between states of the market corresponding to properties of recent order-flow and actions on the agent’s limit price (raise price, lower price, do nothing). In contrast, we situate the ZIP decision rules within a larger policy space by associating probabilities with state–action pair, and allow the agent to learn the optimal policy by adjusting these probabilities using Q-learning. Because we use the same state and action space as ZIP, the resulting policies have virtually identical computational overhead to the original strategy, but with the advantage that we can retrain our strategy to find optimal policies in different trading environments. Using empirical methods, we found that our reinforcement learning agents are sometimes able to outperform the deterministic ZIP agent in at least one scenario.
更多查看译文
关键词
Zero-intelligence traders,Automated trading,Reinforcement learning
AI 理解论文
溯源树
样例
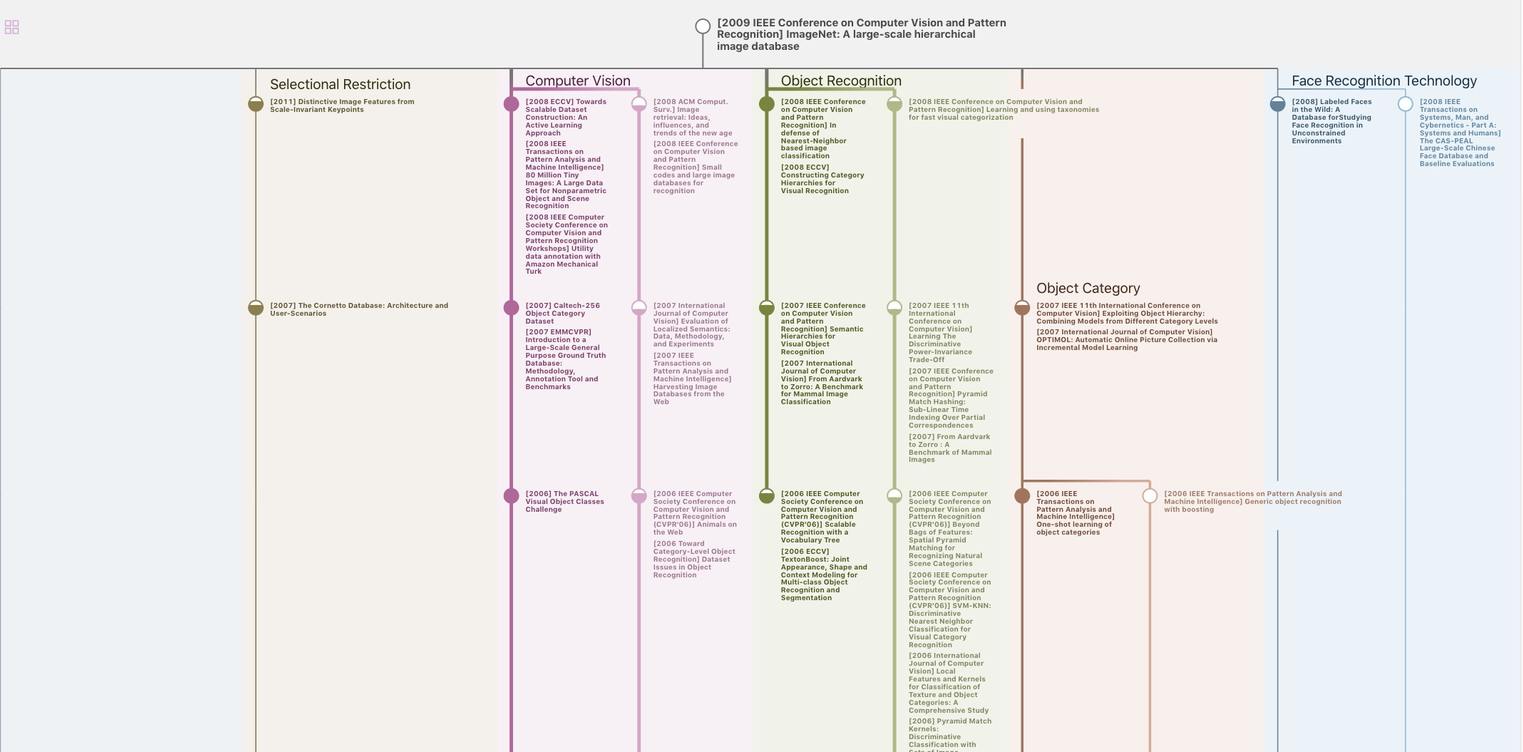
生成溯源树,研究论文发展脉络
Chat Paper
正在生成论文摘要