Predictive Models as Early Warning Systems: A Bayesian Classification Model to Identify At-Risk Students of Programming
SAI (2)(2021)
摘要
The pursuit of a deeper understanding of factors that influence student performance outcomes has long been of interest to the computing education community. Among these include the development of effective predictive models to predict student academic performance. Predictive models may serve as early warning systems to identify students at risk of failing or quitting early. This paper presents a class of machine learning predictive models based on Naive Bayes classification, to predict student performance in introductory programming. The models use formative assessment tasks and self-reported cognitive features such as prior programming knowledge and problem-solving skills. Our analysis revealed that the use of just three variables was a good fit for the models employed. The models that used in-class assessment and cognitive features as predictors returned best at-risk prediction accuracies, compared with models that used take-home assessment and cognitive features as predictors. The prediction accuracy in identifying at-risk students on unknown data for the course was 71% (overall prediction accuracy) in compliance with the area under the curve (ROC) score (0.66). Based on these results we present a generic predictive model and its potential application as an early warning system for early identification of students at risk.
更多查看译文
关键词
Early warning systems, Formative assessment tasks, Predictive data mining models, Problem Solving Skills
AI 理解论文
溯源树
样例
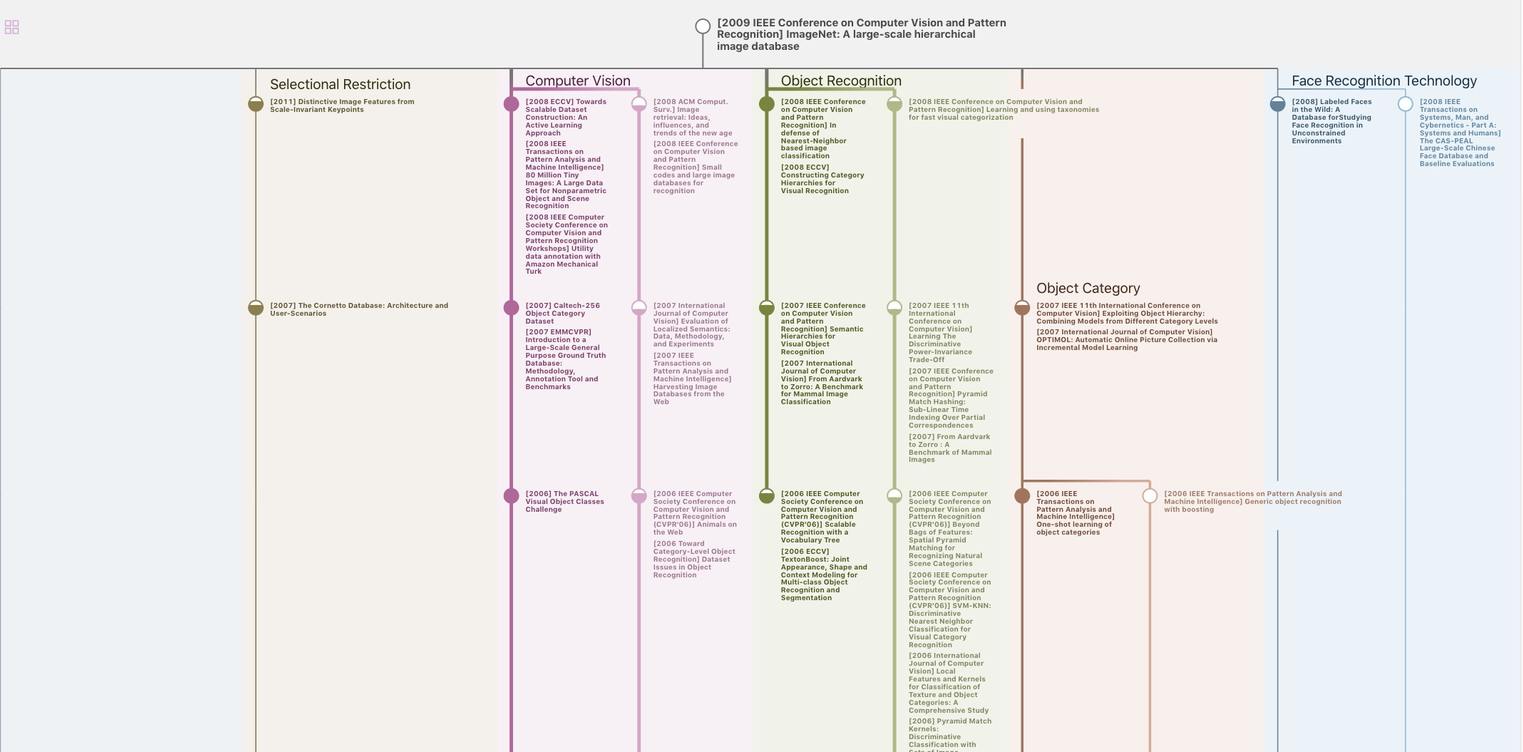
生成溯源树,研究论文发展脉络
Chat Paper
正在生成论文摘要