A Fuzzy-Based Support Vector Regression Framework for Crop Yield Prediction
Advances in intelligent systems and computing(2021)
摘要
This paper proposes a fuzzy-based support vector regression framework for crop yield prediction. To achieve its objectives, interval type-2 fuzzy logic (IT2-FL), principal component analysis (PCA) and support vector regression (SVR) algorithms are employed. The IT2-FL algorithm is used to predict the missing predictor parameter values in the original crop yield prediction dataset while PCA performs feature selection and dimensionality reduction, thereby eliminating redundant information (features) from the transformed dataset by IT2-FL. SVR, machine learning algorithm, is used for model training and testing of the reduced dataset. The performance of the SVR model is evaluated using mean square error (MSE) and root mean square error (RMSE) metrics. Results show that SVR is more accurate at predicting crop yield with an error of 0.002071 for MSE and 0.045513 for RMSE, providing robustness to outliers and minimizing generalization errors with accuracy of 99% for MSE and 95% for RMSE. This indicates that farmers can rely on predicted outcomes from the proposed framework to adopt practices that maximize crop production, improve crop yield, and sustain food sufficiency to the teeming population.
更多查看译文
关键词
Interval type-2 fuzzy logic, Support vector regression, Crop yield prediction, Food sufficiency
AI 理解论文
溯源树
样例
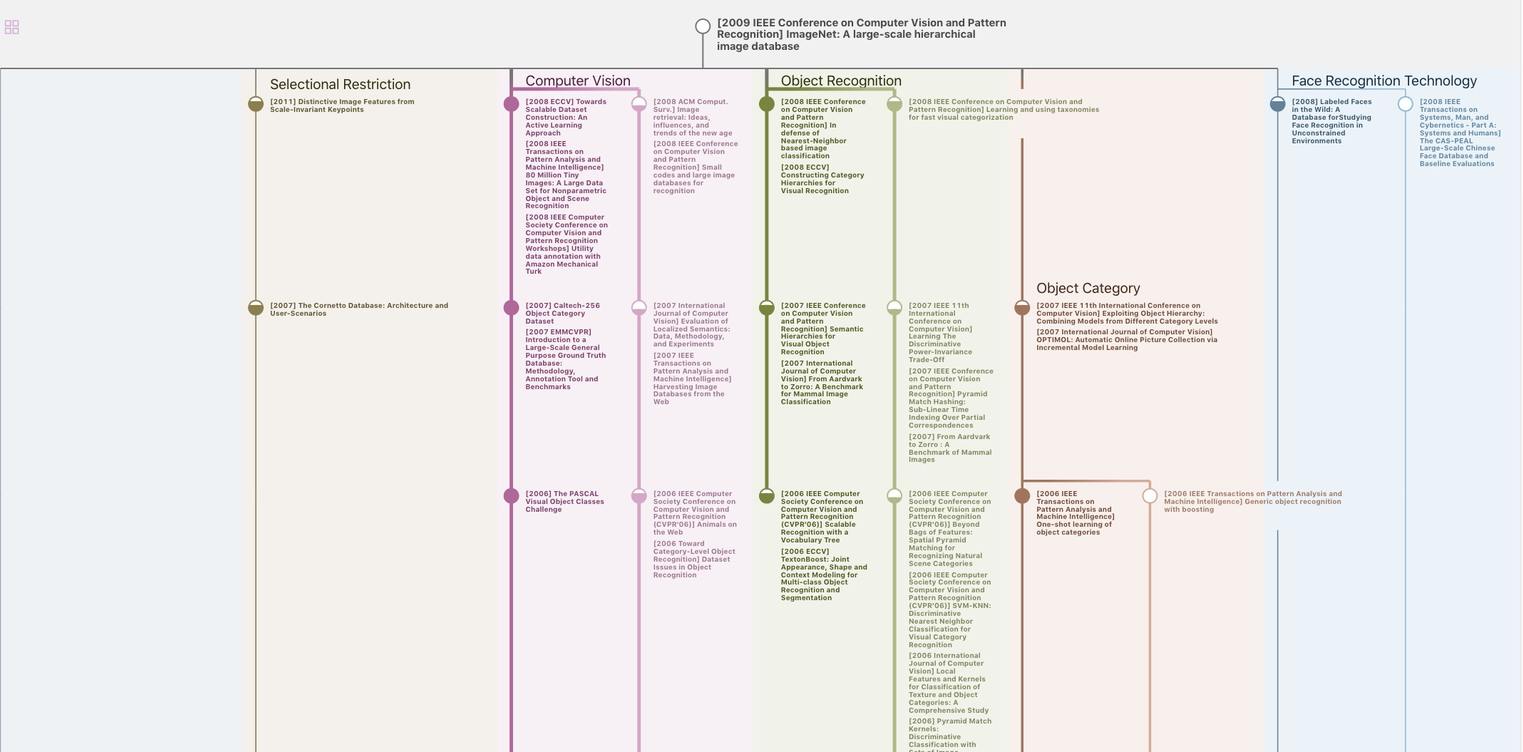
生成溯源树,研究论文发展脉络
Chat Paper
正在生成论文摘要