Effects of Data Augmentation on the Identification of Cough Sound Using Convolutional Neural Networks
crossref
摘要
Cough identification employing deep learning models has generated significant interest in fields such as telemedicine. However, it is challenging to have a considerable number of cough sounds to train such models, and consequently, it is necessary to apply techniques to generate more data. In this work, the effect of data augmentation on the identification of cough was studied using convolutional neural networks (CNN). For this, a system composed of Front-End and Back-End was implemented. In the Front-End, the original recordings and those generated by data augmentation by frequency shift and noise injection were processed and transformed into Mel spectrograms. On the Back-End, the spectrograms were analyzed and classified using a CNN. The results show that the transformations proposed to increase the amount of data help reducing the overfitting by 42.5% while bettering accuracy by a relative improvement of 1%. Therefore, applying data augmentation techniques to train CNNs for differentiating coughs from other sound events results in a simple but effective strategy.
更多查看译文
关键词
Data augmentation, Cough identification, Convolutional neural networks
AI 理解论文
溯源树
样例
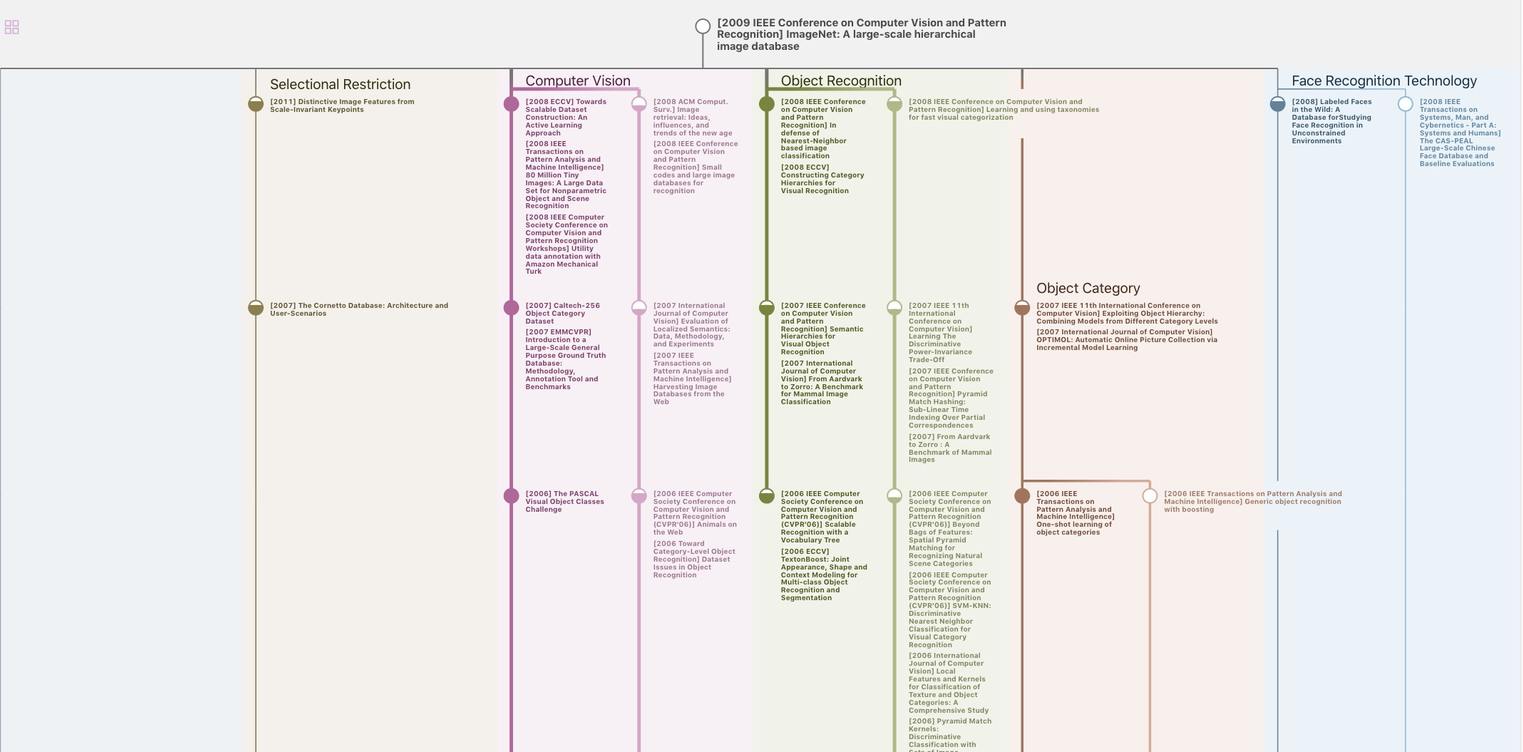
生成溯源树,研究论文发展脉络
Chat Paper
正在生成论文摘要