Placental Vessel Extraction with Shearlets, Laplacian Eigenmaps, and a Conditional Generative Adversarial Network
Association for Women in Mathematics SeriesUnderstanding Complex Biological Systems with Mathematics(2018)
摘要
The placenta is the key organ of maternal–fetal interactions, where nutrient, oxygen, and waste transfer take place. Differences in the morphology of the placental chorionic surface vascular network (PCSVN) have been associated with developmental disorders such as autism, hinting that the PCSVN could potentially serve as a biomarker for early diagnosis and treatment of autism. Studying PCSVN features in large cohorts requires a reliable and automated mechanism to extract the vascular networks. This paper presents two distinct methods for PCSVN enhancement and extraction. Our first algorithm, which builds upon a directional multiscale mathematical framework based on a combination of shearlets and Laplacian eigenmaps, is able to intensify the appearance of vessels with high success in high-contrast images such as those produced in CT scans. Our second algorithm, which applies a conditional generative adversarial neural network (cGAN), was trained to simulate a human-traced PCSVN given a digital photograph of the placental chorionic surface. This method surpasses any existing automated PCSVN extraction methods reported on digital photographs of placentas. We hypothesize that a suitable combination of the two methods could further improve PCSVN extraction results and should be studied in the future.
更多查看译文
关键词
placental vessel extraction,conditional generative adversarial network,laplacian eigenmaps,shearlets
AI 理解论文
溯源树
样例
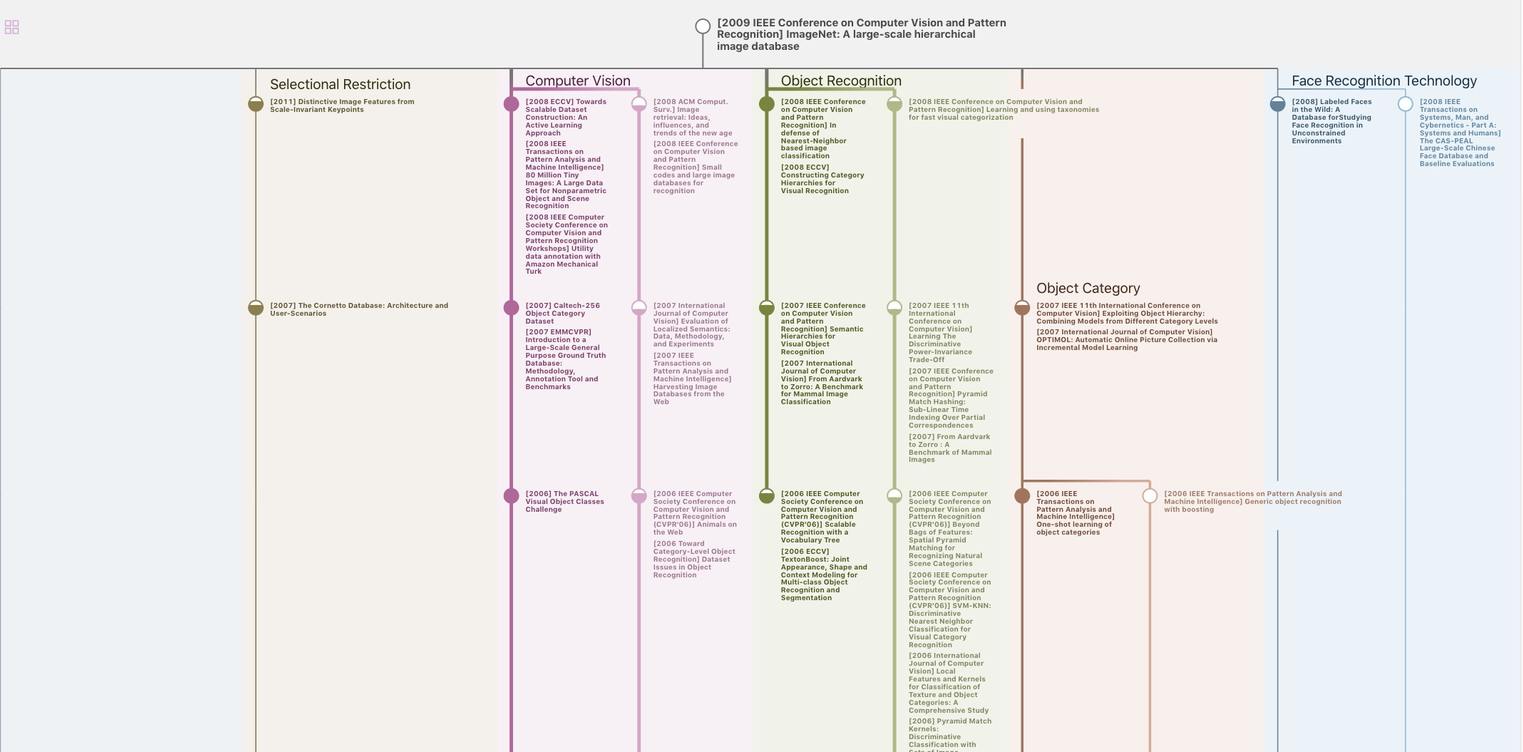
生成溯源树,研究论文发展脉络
Chat Paper
正在生成论文摘要