Assessing the Impacts of Integrating Snowpack Error Distribution in the Management of a Hydropower Reservoir Using Bayesian Stochastic Dynamic Programming (BSDP)
Proceedings of the 6th International Workshop on Hydro Scheduling in Competitive Electricity Markets(2019)
摘要
A hydropower system is presented in which long-term hydrological forecasts must be performed. The system is strongly snowmelt-dominated and the duration of the spring flood can last upwards of 5 months. The risk of flooding is very high when the snowpack is above long-term average values. This work analyzes the impacts of estimating and integrating the snowpack error distribution in a hydrological forecasting framework when optimized by a Bayesian Stochastic Dynamic Programming (BSDP) reservoir management optimization algorithm. The methodology follows two main steps. In the first step, the hydrological model is run on the historical dataset. The resulting hydrograph and hydrologic states are then compared to those of a synthetic "perfect" model simulation. An error distribution is defined between both series that can be used in the BSDP framework. Second, the first step is repeated with a classical SDP approach instead of BSDP to quantify the impacts of using the error distribution on hydropower generation. Results show that BSDP outperforms the classical SDP and that the snowpack error estimation plays a significant role in improving the reservoir management policy.
更多查看译文
关键词
Bayesian, Stochastic, Dynamic, Programming, Snow water equivalent, Hydrological forecasting
AI 理解论文
溯源树
样例
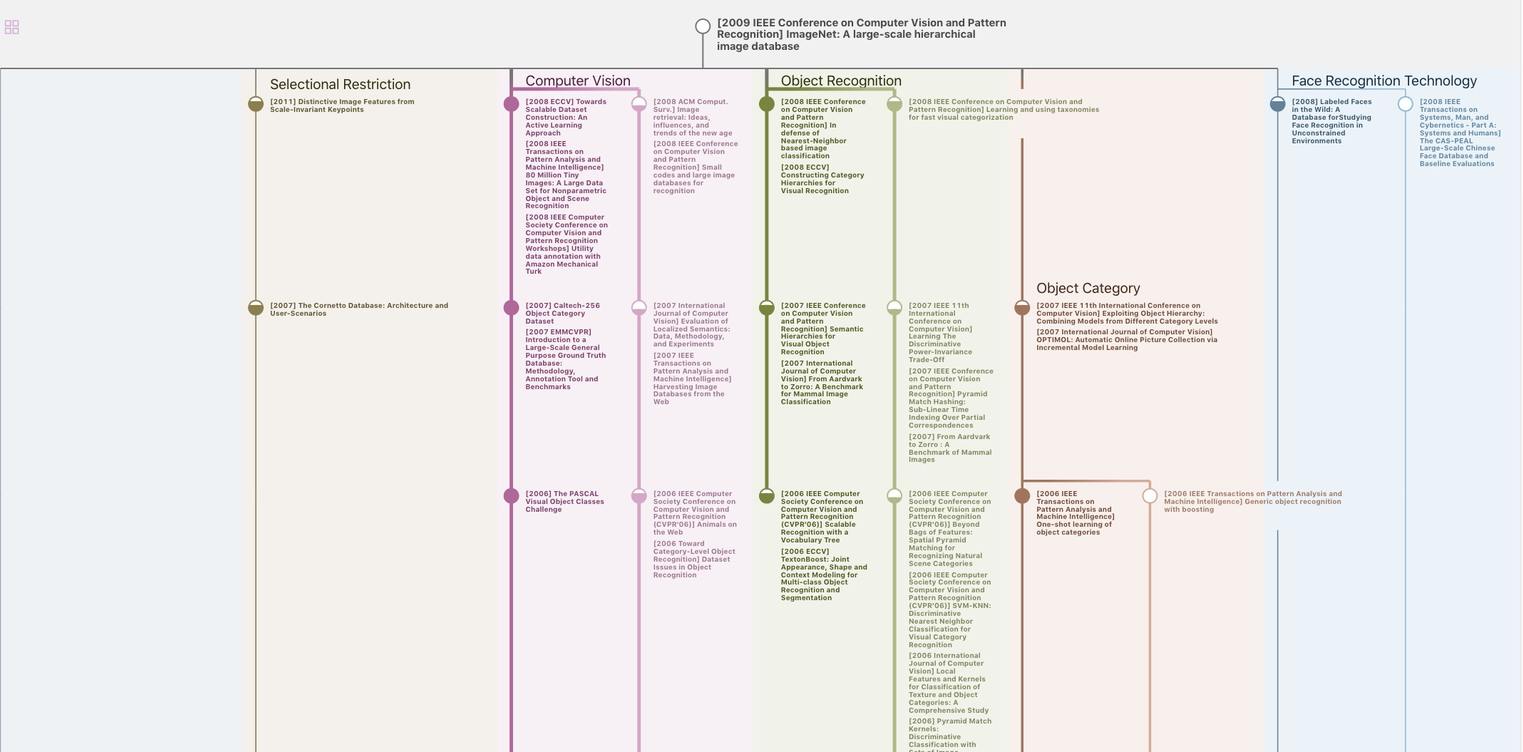
生成溯源树,研究论文发展脉络
Chat Paper
正在生成论文摘要