GIFT: Graph-guIded Feature Transfer for Cold-Start Video Click-Through Rate Prediction
Conference on Information and Knowledge Management(2022)
摘要
ABSTRACTShort video has witnessed rapid growth in the past few years in e-commerce platforms like Taobao. To ensure the freshness of the content, platforms need to release a large number of new videos every day, making conventional click-through rate (CTR) prediction methods suffer from the item cold-start problem. In this paper, we propose GIFT, an efficient Graph-guIded Feature Transfer system, to fully take advantages of the rich information of warmed-up videos to compensate for the cold-start ones. Specifically, we establish a heterogeneous graph that contains physical and semantic linkages to guide the feature transfer process from warmed-up video to cold-start videos.Specifically, we establish a heterogeneous graph that contains physical and semantic linkages to guide the feature transfer process. The physical linkages consist of the explicit relationships (e.g., produced by the same author, or showcasing the same product etc.), and the semantic linkages measure the proximity of multi-modal representations of two videos. We elaborately design the feature transfer function to make aware of different parts of transferred features (e.g., id representations and historical statistics) from different types of nodes and edges along the metapath on the graph. We conduct extensive experiments on a large real-world dataset, and the results show that our GIFT system outperforms SOTA methods significantly and brings a 6.82% lift on CTR in the homepage of Taobao App.
更多查看译文
关键词
Recommender system, Graph representation learning
AI 理解论文
溯源树
样例
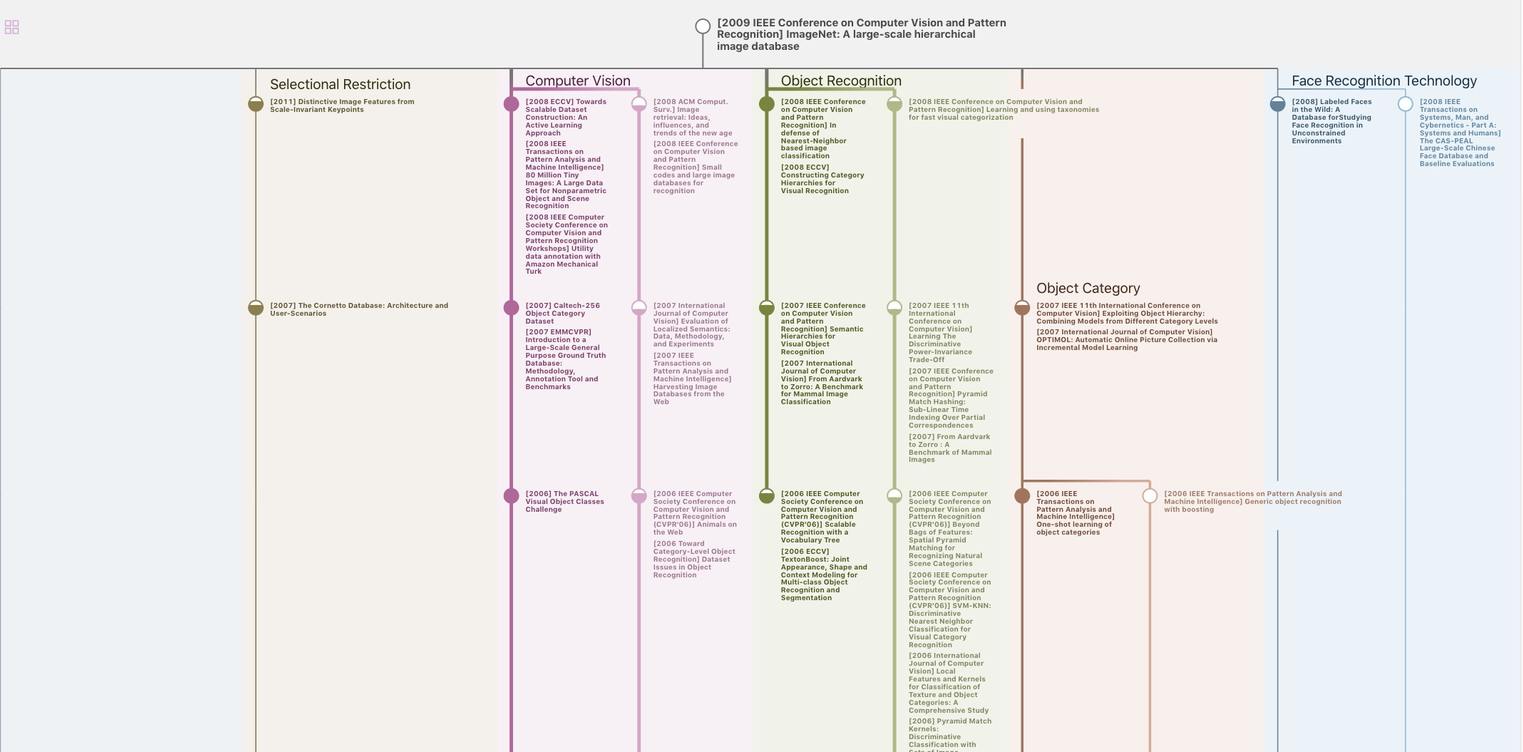
生成溯源树,研究论文发展脉络
Chat Paper
正在生成论文摘要