Contextual Proposal Network for Action Localization
2022 IEEE/CVF Winter Conference on Applications of Computer Vision (WACV)(2022)
摘要
This paper investigates the problem of Temporal Action Proposal (TAP) generation, which aims to provide a set of high-quality video segments that potentially contain actions events locating in long untrimmed videos. Based on the goal to distill available contextual information, we introduce a Contextual Proposal Network (CPN) composing of two context-aware mechanisms. The first mechanism, i.e., feature enhancing, integrates the inception-like module with long-range attention to capture the multi-scale temporal contexts for yielding a robust video segment representation. The second mechanism, i.e., boundary scoring, employs the bi-directional recurrent neural networks (RNN) to capture bi-directional temporal contexts that explicitly model actionness, background, and confidence of proposals. While generating and scoring proposals, such bi-directional temporal contexts are helpful to retrieve high-quality proposals of low false positives for covering the video action instances. We conduct experiments on two challenging datasets of ActivityNet-1.3 and THUMOS-14 to demonstrate the effectiveness of the proposed Contextual Proposal Network (CPN). In particular, our method respectively surpasses state-of-the-art TAP methods by 1.54% AUC on ActivityNet-1.3 test split and by 0.61% AR@200 on THUMOS-14 dataset.
更多查看译文
关键词
Action and Behavior Recognition Motion Processing, Multimedia Applications
AI 理解论文
溯源树
样例
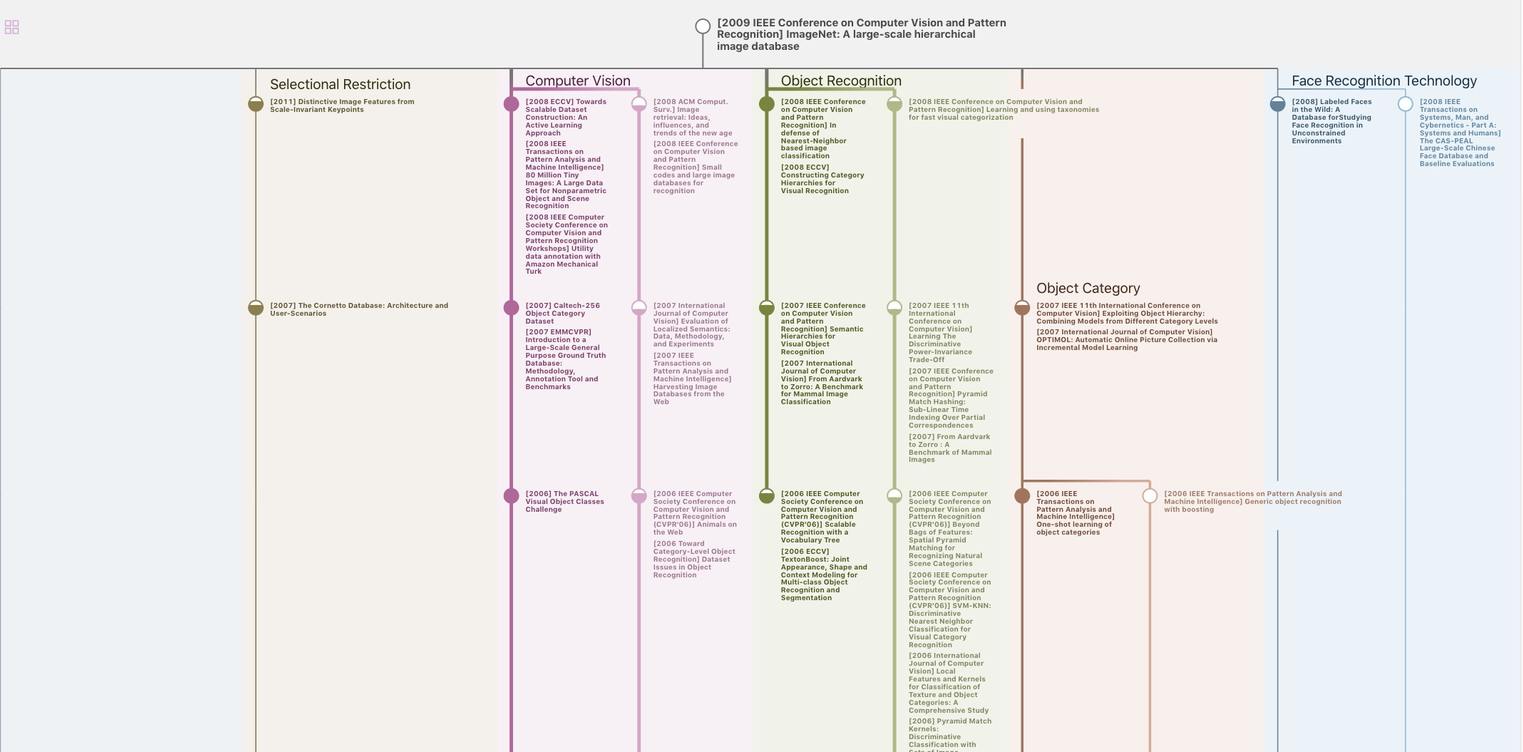
生成溯源树,研究论文发展脉络
Chat Paper
正在生成论文摘要