Comparing the Predictability of Sensor Modalities to Detect Stress from Wearable Sensor Data
2022 IEEE 19th Annual Consumer Communications & Networking Conference (CCNC)(2022)
摘要
Detecting stress from wearable sensor data enables those struggling with unhealthy stress coping mechanisms to better manage their stress. Previous studies have investigated how mechanisms for detecting stress from sensor data can be optimized, comparing alternative algorithms and approaches to find the best possible outcome. One strategy to make these mechanisms more accessible is to reduce the number of sensors that wearable devices must support. Reducing the number of sensors will enable wearable devices to be a smaller size, require less battery, and last longer, making use of these wearable devices more accessible. To progress towards this more convenient stress detection mechanism, we investigate how learning algorithms perform on singular modalities and compare the outcome with results from multiple modalities. We found that singular modalities performed comparably or better than combined modalities on two stress-detection datasets, suggesting that there is promise for detecting stress with fewer sensor requirements. From the four modalities we tested, acceleration, blood volume pulse, and electrodermal activity, we saw acceleration and electrodermal activity to stand out in a few cases, but all modalities showed potential. Our results are acquired from testing with random holdout and leave-one-subject-out validation, using several machine learning techniques. Our results can inspire work on optimizing stress detection with singular modalities to make the benefits of these detection mechanisms more convenient.
更多查看译文
关键词
stress detection,machine learning,wearable sensors
AI 理解论文
溯源树
样例
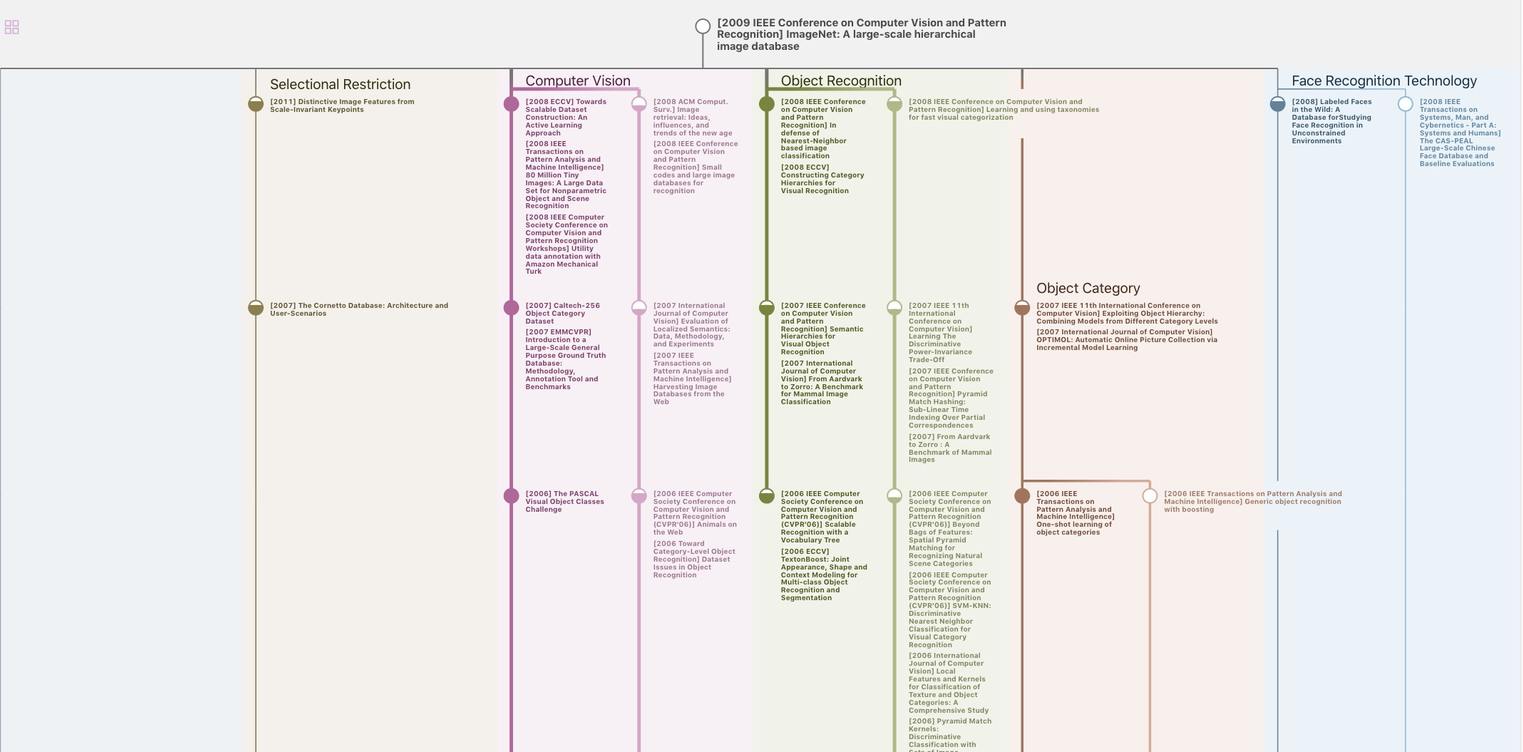
生成溯源树,研究论文发展脉络
Chat Paper
正在生成论文摘要