A fast robotic arm gravity compensation updating approach for industrial application using sparse selection and reconstruction
Robotics and Autonomous Systems(2022)
摘要
With the increasing complexity of robot tasks in the industrial field, sometimes the manipulator needs to change the payload or replace end-effectors frequently in a period of time, which will cause the change of gravity model. This paper presents a fast gravity compensation updating method based on sparse selection and reconstruction. Instead of using the classic model, feature sets in the formulation of dynamic motion are exploited and the gravity model learning is transformed into a sparse problem. Then, the Alternating Direction Multiplier Method (ADMM) is used to accelerate the process of solving the sparse optimization problem and reconstructing the effective features of the gravity model from the original signal. The merits of our method are that it does not depend on any kinematic and dynamic parameters, and there is no need to redesign the specific excitation trajectory in the gravity model updating. Thus, the updating process avoids heavy work of calibration and simplifies the labor complexity considerably from the conventional analytical methods. The results of various payload experiments on a real 7-DOF manipulator show that the proposed method can update the gravity compensation model efficiently and accurately.
更多查看译文
关键词
Industrial robot arm,Gravity compensation,Feature selection,Model reconstruction,ADMM
AI 理解论文
溯源树
样例
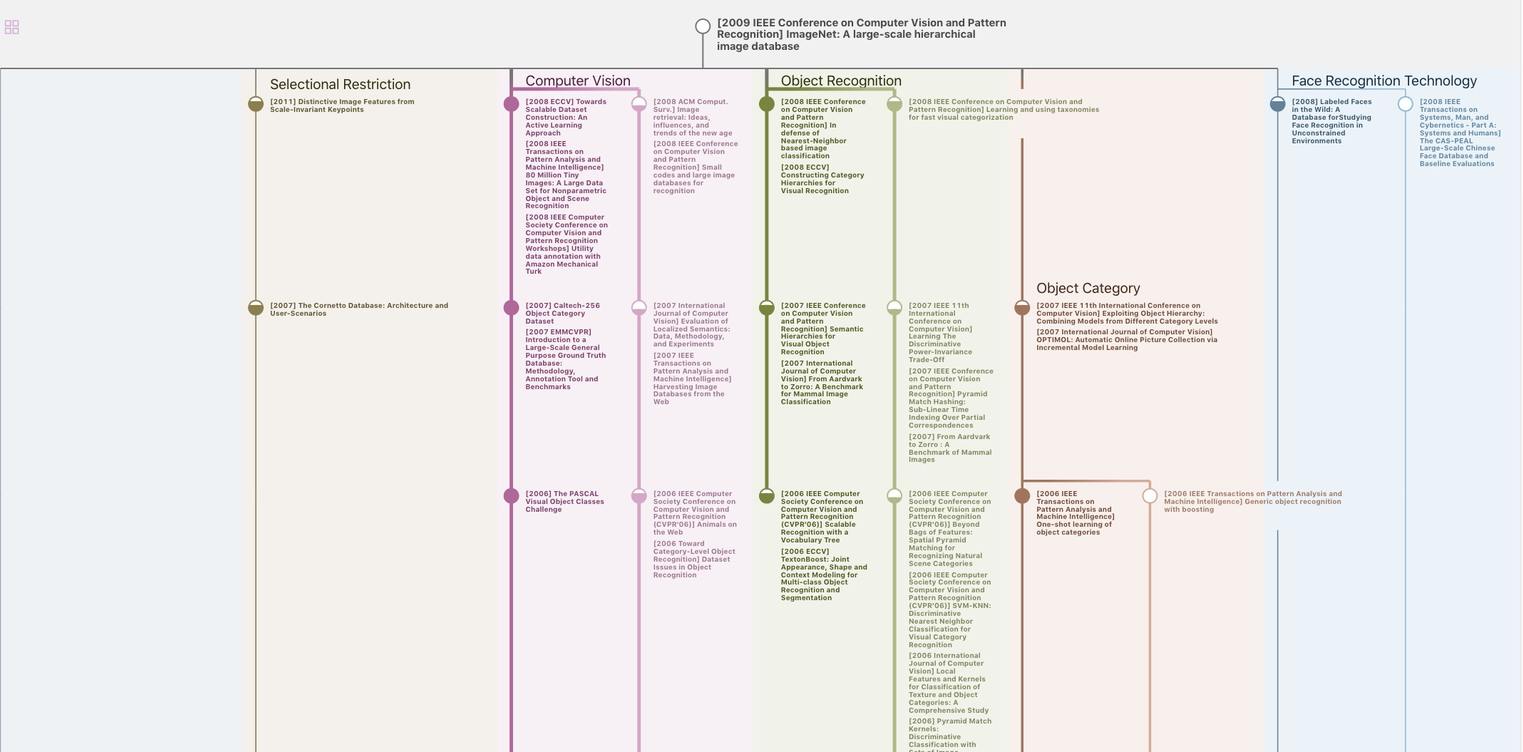
生成溯源树,研究论文发展脉络
Chat Paper
正在生成论文摘要