GNN-Retro: Retrosynthetic Planning with Graph Neural Networks.
AAAI Conference on Artificial Intelligence(2022)
摘要
Retrosynthetic planning plays an important role in the field of organic chemistry, which could generate a synthetic route for the target product. The synthetic route is a series of reactions which are started from the available molecules. The most challenging problem in the generation of the synthetic route is the large search space of the candidate reactions. Estimating the cost of candidate reactions has been proved effectively to prune the search space, which could achieve a higher accuracy with the same search iteration. And the estimation of one reaction is comprised of the estimations of all its reactants. So, how to estimate the cost of these reactants will directly influence the quality of results. To get a better performance, we propose a new framework, named GNN-Retro, for retrosynthetic planning problem by combining graph neural networks(GNN) and the latest search algorithm. The structure of GNN in our framework could incorporate the information of neighboring molecules, which will improve the estimation accuracy of our framework. The experiments on the USPTO dataset show that our framework could outperform the state-of-the-art methods with a large margin under the same settings.
更多查看译文
关键词
Data Mining & Knowledge Management (DMKM)
AI 理解论文
溯源树
样例
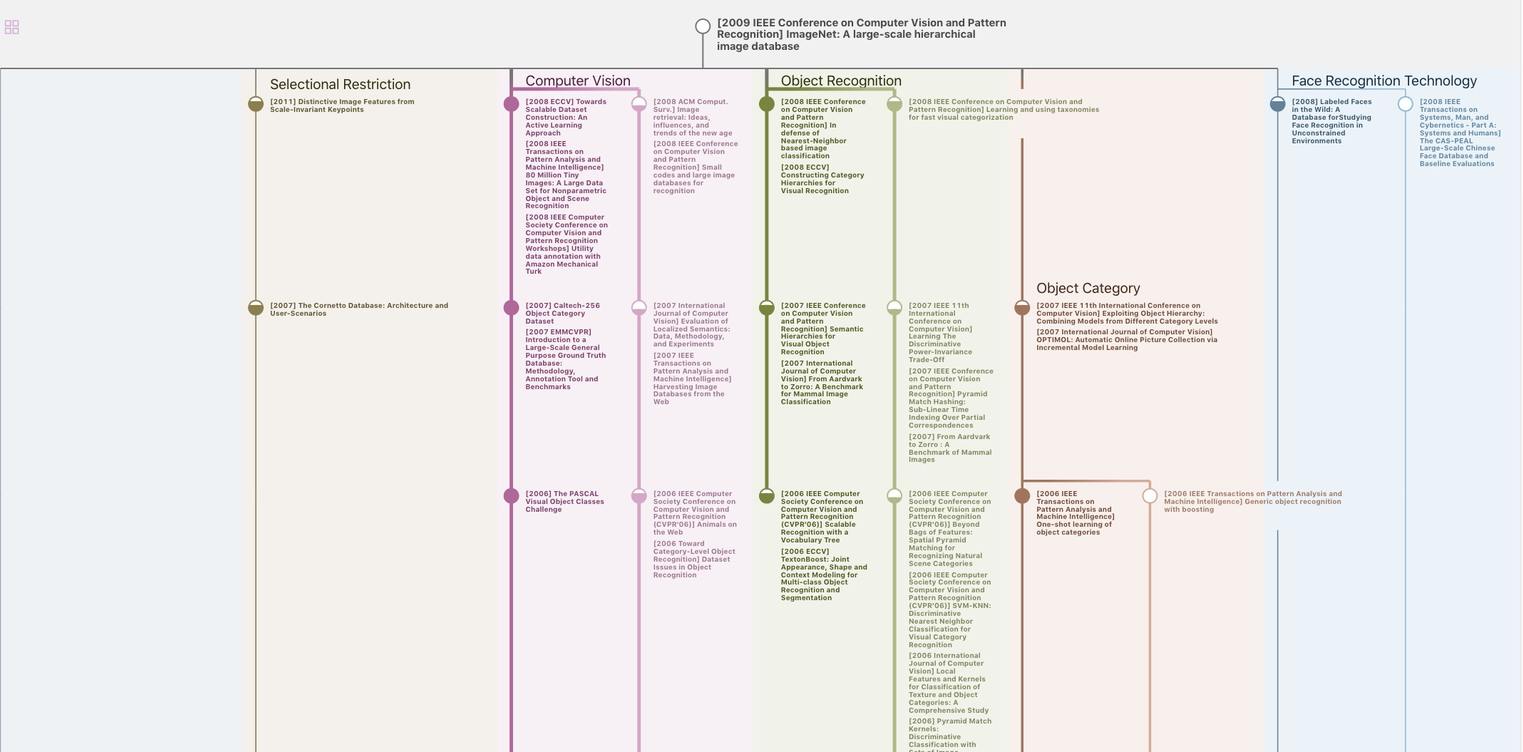
生成溯源树,研究论文发展脉络
Chat Paper
正在生成论文摘要