Learning Physics-Informed Neural Networks without Stacked Back-propagation
arxiv(2023)
摘要
Physics-Informed Neural Network (PINN) has become a commonly used machine learning approach to solve partial differential equations (PDE). But, facing high-dimensional secondorder PDE problems, PINN will suffer from severe scalability issues since its loss includes second-order derivatives, the computational cost of which will grow along with the dimension during stacked back-propagation. In this work, we develop a novel approach that can significantly accelerate the training of Physics-Informed Neural Networks. In particular, we parameterize the PDE solution by the Gaussian smoothed model and show that, derived from Stein's Identity, the second-order derivatives can be efficiently calculated without back-propagation. We further discuss the model capacity and provide variance reduction methods to address key limitations in the derivative estimation. Experimental results show that our proposed method can achieve competitive error compared to standard PINN training but is significantly faster. Our code is released at https://github.com/LithiumDA/PINN-without-Stacked-BP.
更多查看译文
关键词
neural networks,physics-informed,back-propagation
AI 理解论文
溯源树
样例
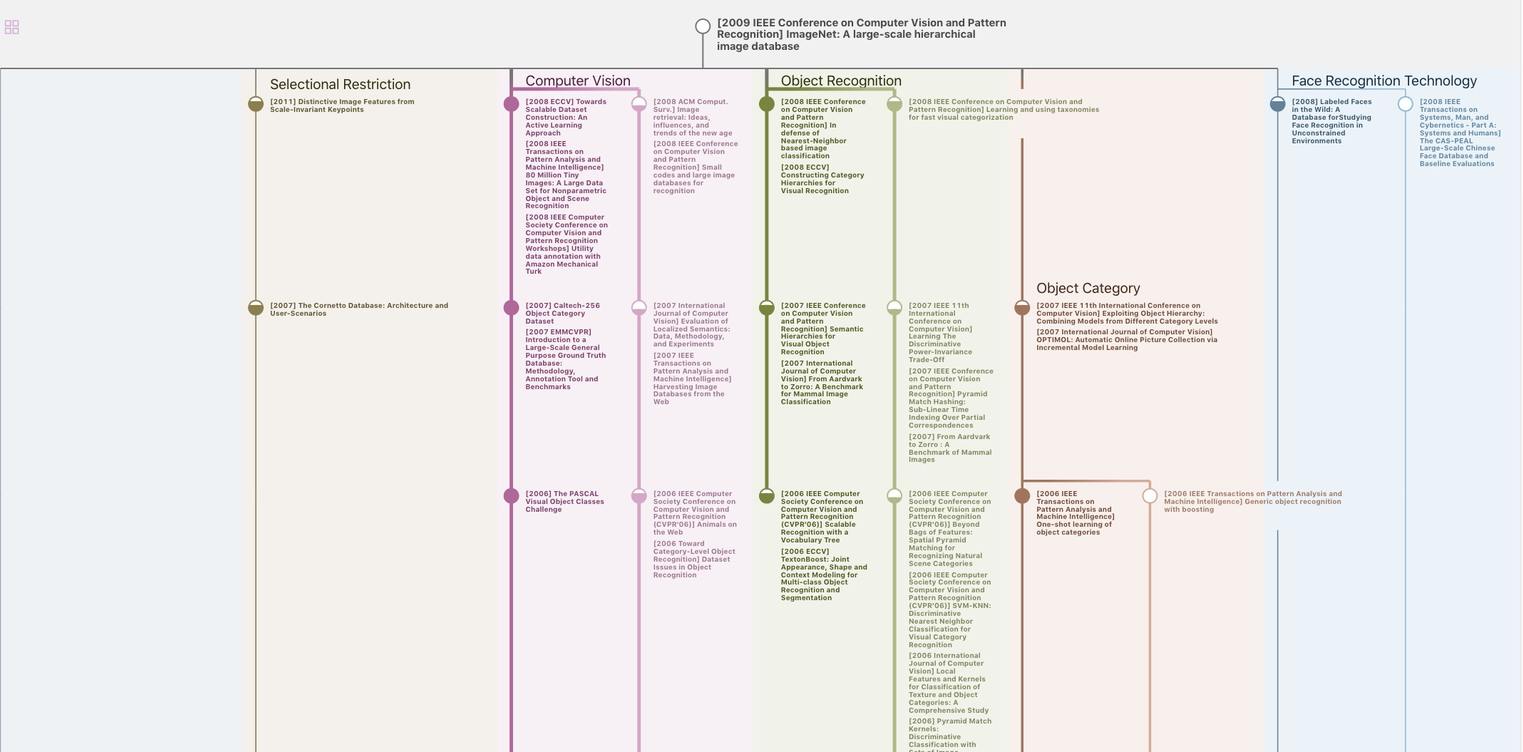
生成溯源树,研究论文发展脉络
Chat Paper
正在生成论文摘要