Attention Enhanced Reinforcement Learning for Multi agent Cooperation
IEEE transactions on neural networks and learning systems(2023)
摘要
In this article, a novel method, called attention enhanced reinforcement learning (AERL), is proposed to address issues including complex interaction, limited communication range, and time-varying communication topology for multi agent cooperation. AERL includes a communication enhanced network (CEN), a graph spatiotemporal long short-term memory network (GST-LSTM), and parameters sharing multi-pseudo critic proximal policy optimization (PS-MPC-PPO). Specifically, CEN based on graph attention mechanism is designed to enlarge the agents’ communication range and to deal with complex interaction among the agents. GST-LSTM, which replaces the standard fully connected (FC) operator in LSTM with graph attention operator, is designed to capture the temporal dependence while maintaining the spatial structure learned by CEN. PS-MPC-PPO, which extends proximal policy optimization (PPO) in multi agent systems with parameters’ sharing to scale to environments with a large number of agents in training, is designed with multi-pseudo critics to mitigate the bias problem in training and accelerate the convergence process. Simulation results for three groups of representative scenarios including formation control, group containment, and predator–prey games demonstrate the effectiveness and robustness of AERL.
更多查看译文
关键词
Training,Reinforcement learning,Games,Scalability,Task analysis,Standards,Optimization,Attention mechanism,deep reinforcement learning (DRL),graph convolutional networks,multi agent systems
AI 理解论文
溯源树
样例
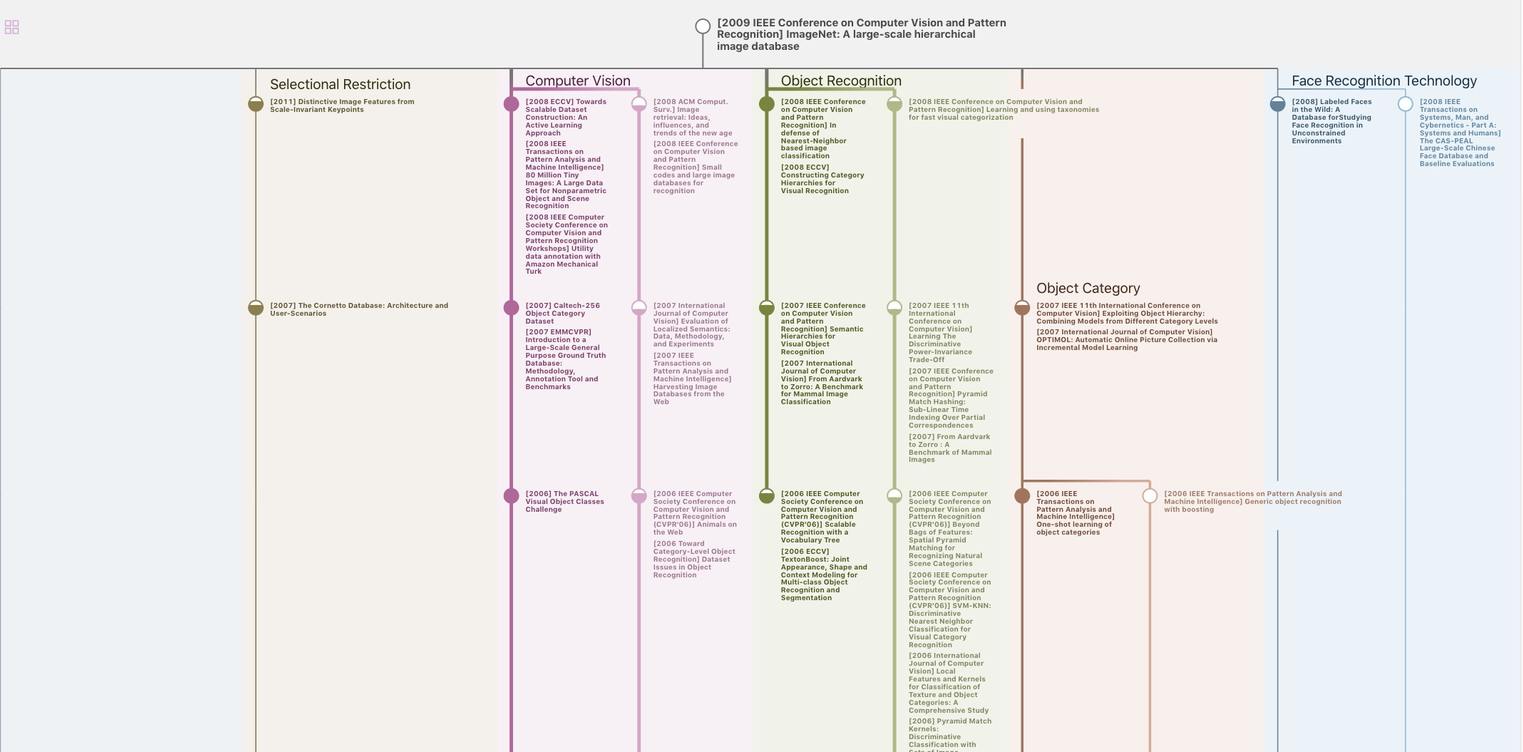
生成溯源树,研究论文发展脉络
Chat Paper
正在生成论文摘要