Entropy-Driven Stochastic Policy for Fast Federated Learning in Beyond 5G Edge-RAN
2021 IEEE GLOBAL COMMUNICATIONS CONFERENCE (GLOBECOM)(2021)
摘要
Scalability and sustainability are the corner stones to unleash the potential of beyond fifth-generation (B5G) ultra-dense networks that are expected to handle massive and heterogeneous services. This implies that the transport of the underlying raw monitoring data should be minimized across the network, and urges to bring the analysis functions closer to the data collection points. While federated learning (FL) is an efficient tool to implement such a decentralized strategy, real networks are generally characterized by time- and space-varying users distributions, traffic profiles, and channel conditions. This makes the data collected across different points non independent and identically distributed (non-IID), which is challenging for FL tasks. To cope with this issue, we first introduce a new a priori metric that we call dataset entropy, whose role is to capture the distribution, the quantity of information, the unbalanced structure and the "non-IIDness" of a dataset independently of the models. This entropy is calculated using a clustering scheme based on a similarity matrix defined over both the features and the supervised output spaces, and is targeting classification as well as regression tasks. The FL aggregation server then uses the reported dataset entropies to devise i) an entropy-based federated averaging scheme, and ii) a stochastic participant selection policy to significantly stabilize the training, minimize the convergence time, and reduce the corresponding computation cost. Numerical results are provided to illustrate all these advantages.
更多查看译文
关键词
convergence time,entropy-driven stochastic policy,fast federated learning,corner stones,ultra-dense networks,massive services,heterogeneous services,underlying raw monitoring data,data collection points,decentralized strategy,space-varying users distributions,traffic profiles,channel conditions,FL tasks,dataset entropy,unbalanced structure,clustering scheme,supervised output spaces,regression tasks,FL aggregation server,stochastic participant selection policy,beyond 5G edge-RAN
AI 理解论文
溯源树
样例
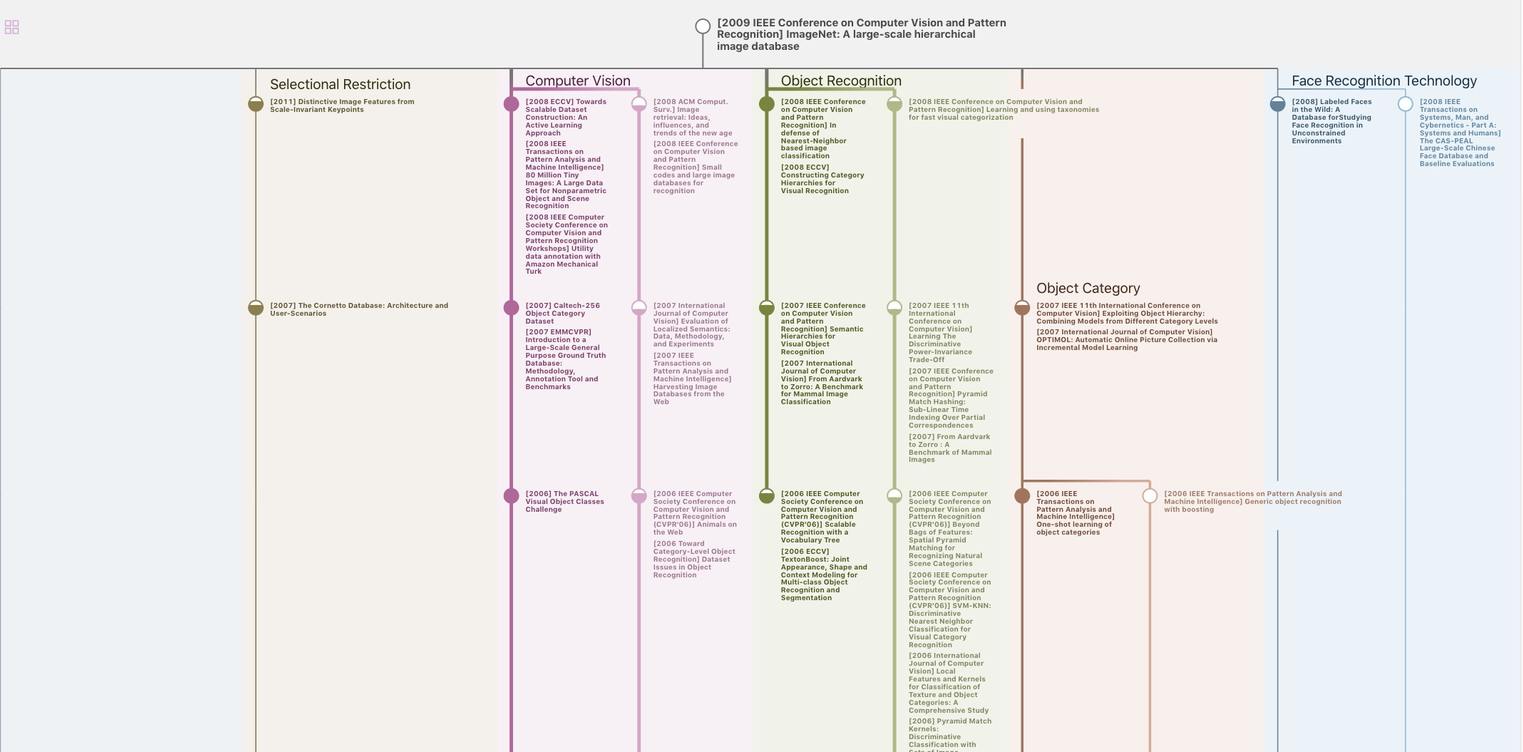
生成溯源树,研究论文发展脉络
Chat Paper
正在生成论文摘要