A Diagnostic Study Of Visual Question Answering With Analogical Reasoning
ICIP(2021)
摘要
The deep learning community has made rapid progress in low-level visual perception tasks such as object localization, detection and segmentation. However, for tasks such as Visual Question Answering (VQA) and visual language grounding that require high-level reasoning abilities, huge gaps still exist between artificial systems and human intelligence. In this work, we perform a diagnostic study on recent popular VQA in terms of analogical reasoning. We term it as Analogical VQA, where a system needs to reason on a group of images to find analogical relations among them in order to correctly answer a natural language question. To study the task in depth, we propose an initial diagnostic synthetic dataset CLEVR-Analogy, which tests a range of analogical reasoning abilities (e.g. reasoning on object attributes, spatial relationships, existence, and arithmetic analogies). We benchmark various recent state-of-the-art methods on our dataset and compare the results against human performance, and discover that existing systems fall shorts when facing analogical reasoning involving spatial relationships. The dataset and code will be publicly available to facilitate future research.
更多查看译文
关键词
analogical reasoning,visual reasoning,Visual Question Answering (VQA),synthetic dataset,benchmark
AI 理解论文
溯源树
样例
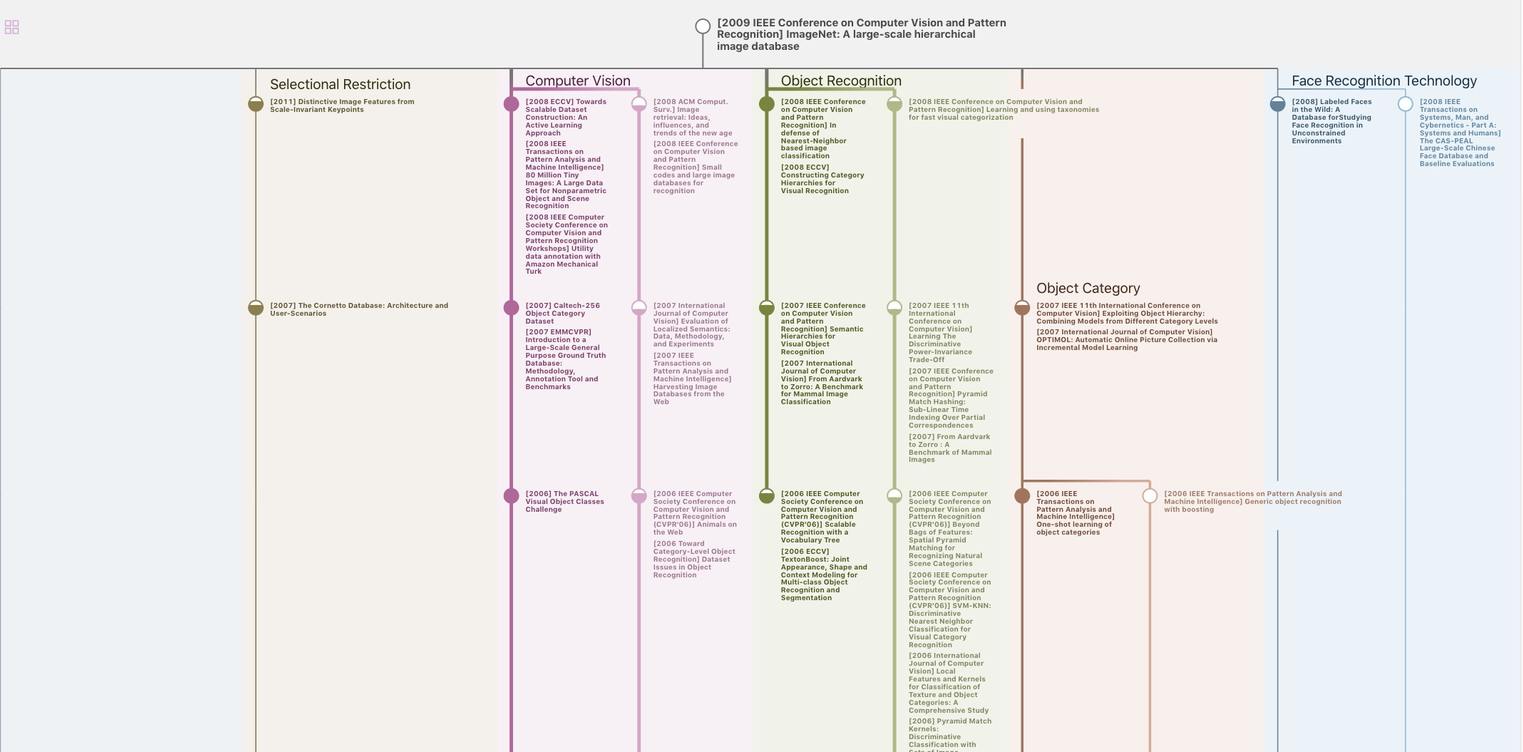
生成溯源树,研究论文发展脉络
Chat Paper
正在生成论文摘要