Confident Semantic Ranking Loss for Part Parsing.
ICME(2021)
摘要
Part parsing is taken as a dense prediction task, assigning each pixel a semantic part label. Some previous methods tried to model the human-known relationships among different parts (inter-part). However, these methods are hard to be used for multi-object part parsing since the given relationships are highly dependent on human priors which require the special model to learn. In addition, pixels in the same part (intra-part) are always assumed equally important. In fact, even they belong to the same part, some pixels are quite uncertain for their predictions while some are with high confidence, but theoretically they are representing the same feature. In this paper, we study the inequality and uncertainty of intra-part and inter-part pixels and propose the confident-semantic-ranking (CO-Rank) loss function, which maximizes the similarities of different groups of pixels to alleviate the uncertainty and models the pixel relationships among intra-/inter-parts. In addition, previous feature maps lost some of part-level relationships due to simply using the global max/average pooling, hence, we propose a new Global Object Pooling layer (GOP) to encode the abundant global information while preserving the geometry details. The experimental results show that our proposed method achieves new state-of-the-art performance on multi-class part parsing benchmark Pascal-Part dataset.
更多查看译文
关键词
Part parsing,uncertainty,loss function,global pooling
AI 理解论文
溯源树
样例
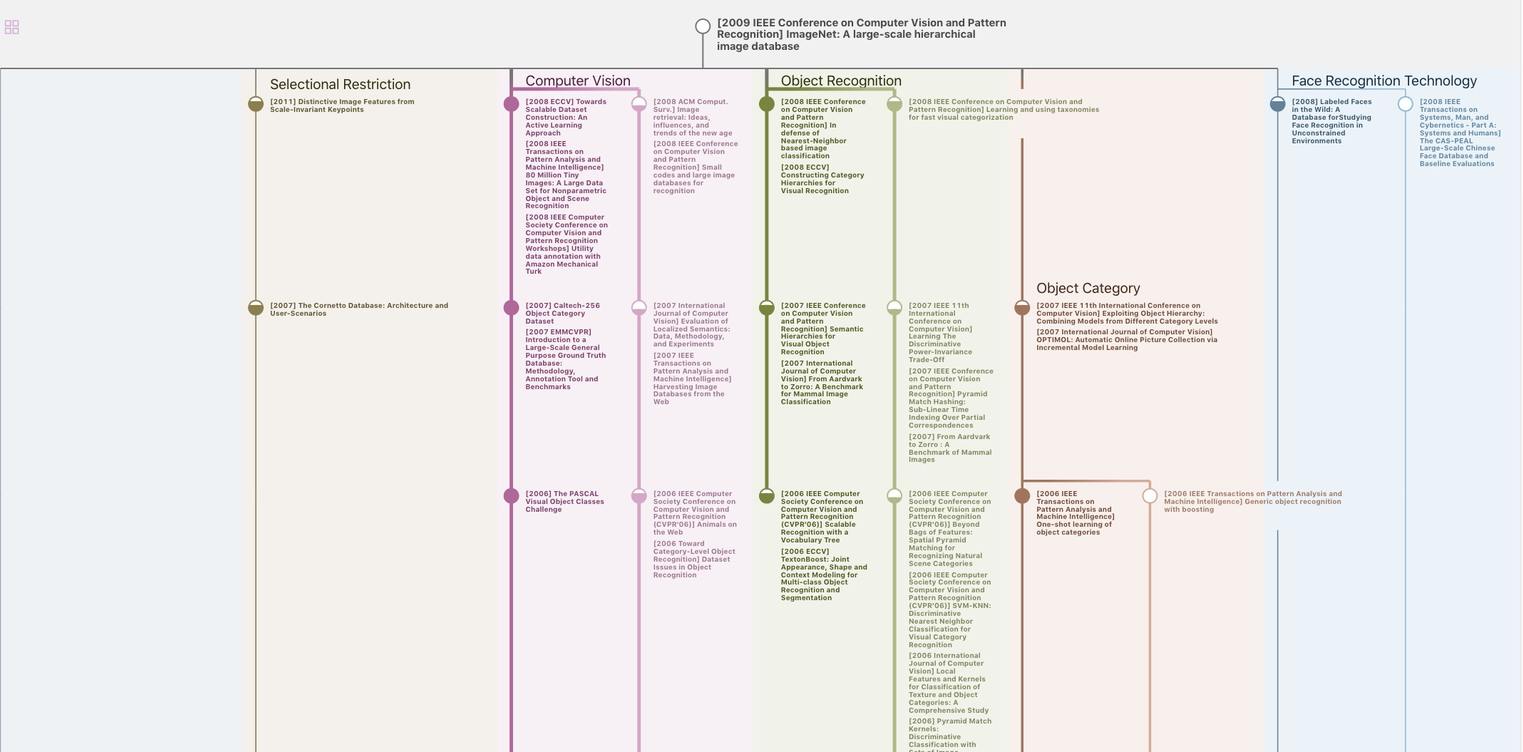
生成溯源树,研究论文发展脉络
Chat Paper
正在生成论文摘要