VAEPass: A lightweight passwords guessing model based on variational auto-encoder
COMPUTERS & SECURITY(2022)
摘要
Password guessing has attracted considerable attention in recent years. With the successful application of deep learning (DL) methods in natural language processing, password guessing models that leverage deep learning techniques by treating passwords as short texts, e.g., Recurrent Neural Network-based model and PassGAN model, have been confirmed to be more effective in terms of generalizability. However, the architectures of these existing DL-based password guessing models are typically extremely complex, which makes the process of training and password generation time-consuming. It is desirable to build a lightweight password guessing model that can reduce the time required for model training while maintaining the password guessing effect. In this study, we propose VAEPass, a lightweight password guessing model based on a Variational Auto-Encoder (VAE), which comprises of an encoder and a decoder established using Gated Convolutional Neural Network (GCNN). Furthermore, we improve the proposed VAEPass model to treat common character combinations summarized from training passwords as tokens and guess passwords, known as VAEPass(token), at a token-level. Experiments demonstrate that the matching rate of the proposed VAEPass(token) is 2.7%similar to 9.3% higher than that of PassGAN method in the one-site test. Moreover, compared with the state-of-the-art PassGAN model (i.e., a DL-based model), the parameters in VAEPass are approximately 32% of that in PassGAN and the training time required by VAEPass is approximately 11% of the time required by PassGAN. (C) 2021 Elsevier Ltd. All rights reserved.
更多查看译文
关键词
Password guessing attack, Information security, Variational auto-encoder, Gated convolutional neural network, Password authentication
AI 理解论文
溯源树
样例
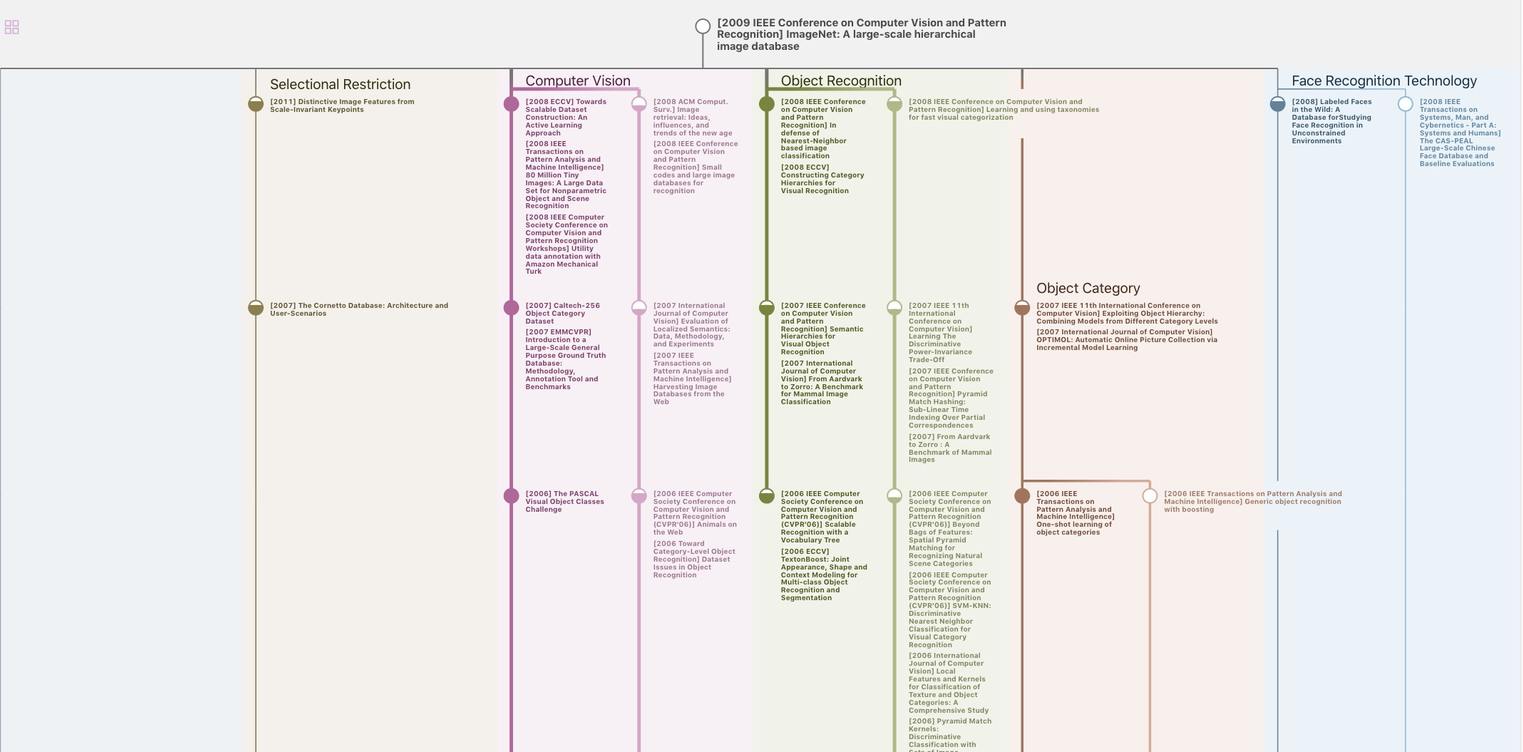
生成溯源树,研究论文发展脉络
Chat Paper
正在生成论文摘要