Parameter Training Methods for Convolutional Neural Networks With Adaptive Adjustment Method Based on Borges Difference
IEEE Transactions on Signal Processing(2022)
摘要
This paper proposes a momentum algorithm based on Borges difference and an adaptive momentum (Adam) algorithm based on Borges difference to update parameters, which can adjust the momentum information more flexibly. The Borges difference is proposed from the definition of Borges derivative to be combined with the gradient algorithm in convolutional neural networks. The proposed momentum algorithm based on Borges difference and Adam algorithm based on Borges difference can be adjusted more flexibly in order to speed up the convergence. The parameter optimization algorithm with the Borges difference presents a better performance compared with the integer-order momentum algorithm and integer-order Adam algorithm, with the proposed nonlinear adjustment method for the parameter tuning of convolutional neural networks. By analyzing experimental results of Fashion-MNIST dataset and CIFAR-10 dataset, the optimization algorithms based on Borges difference proposed in this paper gain better effects on the optimization model compared with the corresponding ones based on the integer-order difference, and can speed up the convergence speed and recognition accuracy of the image recognition.
更多查看译文
关键词
Fractal difference,convolution neural networks,momentum,Adam algorithm,nonlinear adaptive tuning method
AI 理解论文
溯源树
样例
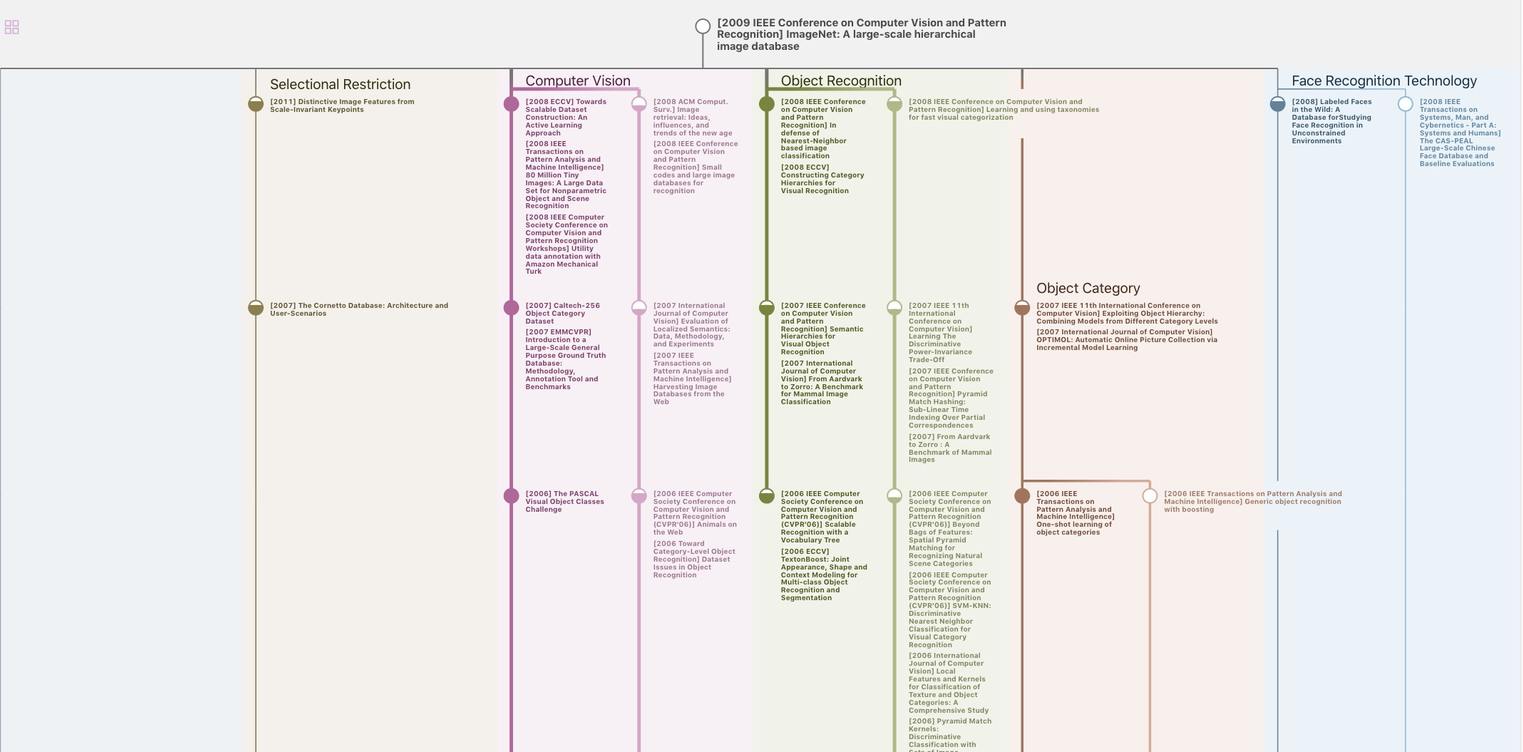
生成溯源树,研究论文发展脉络
Chat Paper
正在生成论文摘要