Decentralized learning of randomization-based neural networks with centralized equivalence
APPLIED SOFT COMPUTING(2022)
摘要
We consider a decentralized learning problem where training data samples are distributed over agents (processing nodes) of an underlying communication network topology without any central (master) node. Due to information privacy and security issues in a decentralized setup, nodes are not allowed to share their training data and only parameters of the neural network are allowed to be shared. This article investigates decentralized learning of randomization-based neural networks that provides centralized equivalent performance as if the full training data are available at a single node. We consider five randomization-based neural networks that use convex optimization for learning. Two of the five neural networks are shallow, and the others are deep. The use of convex optimization is the key to apply alternating-direction-method-of-multipliers with decentralized average consensus. This helps us to establish decentralized learning with centralized equivalence. For the underlying communication network topology, we use a doubly-stochastic network policy matrix and synchronous communications. Experiments with nine benchmark datasets show that the five neural networks provide good performance while requiring low computational and communication complexity for decentralized learning. The performance rankings of five neural networks using Friedman rank are also enclosed in the results, which are ELM < RVFL< dRVFL < edRVFL < SSFN. (c) 2021 The Author(s). Published by Elsevier B.V. This is an open access article under the CC BY-NC-ND license (http://creativecommons.org/licenses/by-nc-nd/4.0/).
更多查看译文
关键词
Randomized neural network, Distributed learning, Multi-layer feedforward neural network, Alternating direction method of multipliers
AI 理解论文
溯源树
样例
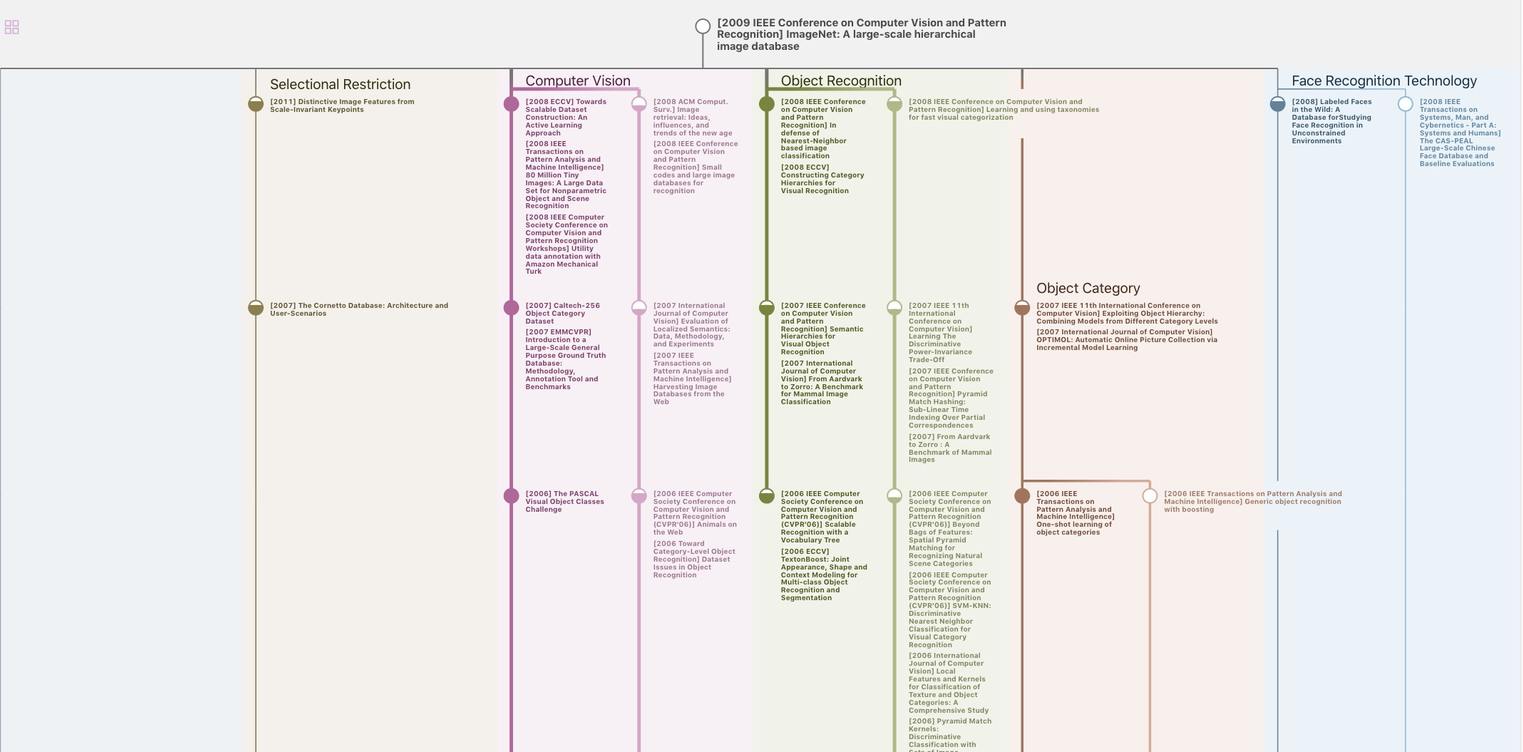
生成溯源树,研究论文发展脉络
Chat Paper
正在生成论文摘要