Dual Encoder-Based Dynamic-Channel Graph Convolutional Network With Edge Enhancement for Retinal Vessel Segmentation
IEEE Transactions on Medical Imaging(2022)
摘要
Retinal vessel segmentation with deep learning technology is a crucial auxiliary method for clinicians to diagnose fundus diseases. However, the deep learning approaches inevitably lose the edge information, which contains spatial features of vessels while performing down-sampling, leading to the limited segmentation performance of fine blood vessels. Furthermore, the existing methods ignore the dynamic topological correlations among feature maps in the deep learning framework, resulting in the inefficient capture of the channel characterization. To address these limitations, we propose a novel dual encoder-based dynamic-channel graph convolutional network with edge enhancement (DE-DCGCN-EE) for retinal vessel segmentation. Specifically, we first design an edge detection-based dual encoder to preserve the edge of vessels in down-sampling. Secondly, we investigate a dynamic-channel graph convolutional network to map the image channels to the topological space and synthesize the features of each channel on the topological map, which solves the limitation of insufficient channel information utilization. Finally, we study an edge enhancement block, aiming to fuse the edge and spatial features in the dual encoder, which is beneficial to improve the accuracy of fine blood vessel segmentation. Competitive experimental results on five retinal image datasets validate the efficacy of the proposed DE-DCGCN-EE, which achieves more remarkable segmentation results against the other state-of-the-art methods, indicating its potential clinical application.
更多查看译文
关键词
Algorithms,Fundus Oculi,Image Processing, Computer-Assisted,Neural Networks, Computer,Retinal Vessels
AI 理解论文
溯源树
样例
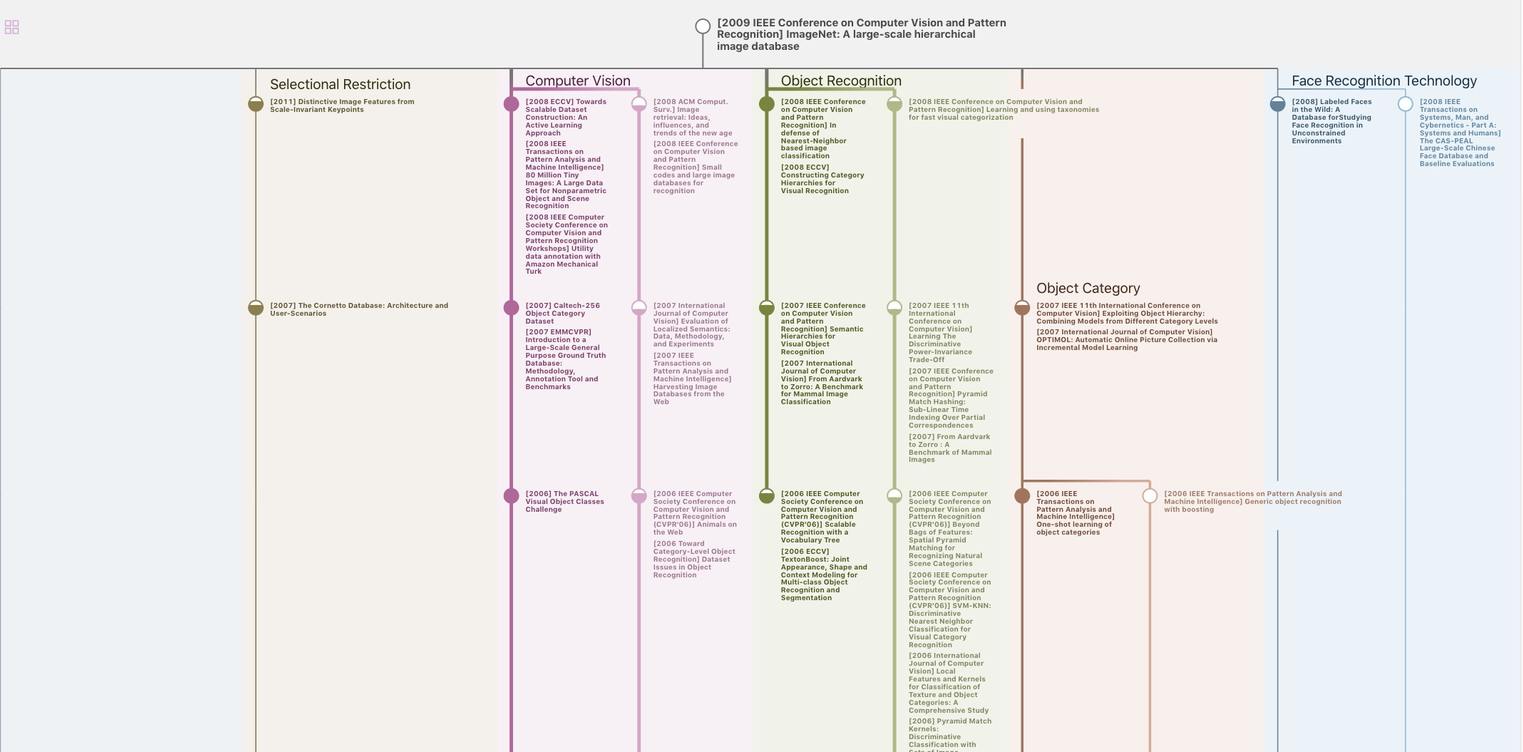
生成溯源树,研究论文发展脉络
Chat Paper
正在生成论文摘要