General-purpose, long-context autoregressive modeling with Perceiver AR.
International Conference on Machine Learning(2022)
摘要
Real-world data is high-dimensional: a book, image, or musical performance can easily contain hundreds of thousands of elements even after compression. However, the most commonly used autoregressive models, Transformers, are prohibitively expensive to scale to the number of inputs and layers needed to capture this long-range structure. We develop Perceiver AR, an autoregressive, modality-agnostic architecture which uses cross-attention to map long-range inputs to a small number of latents while also maintaining end-to-end causal masking. Perceiver AR can directly attend to over a hundred thousand tokens, enabling practical long-context density estimation without the need for hand-crafted sparsity patterns or memory mechanisms. When trained on images or music, Perceiver AR generates outputs with clear long-term coherence and structure. Our architecture also obtains state-of-the-art likelihood on long-sequence benchmarks, including 64x64 ImageNet images and PG-19 books.
更多查看译文
关键词
autoregressive modeling,ar,general-purpose,long-context
AI 理解论文
溯源树
样例
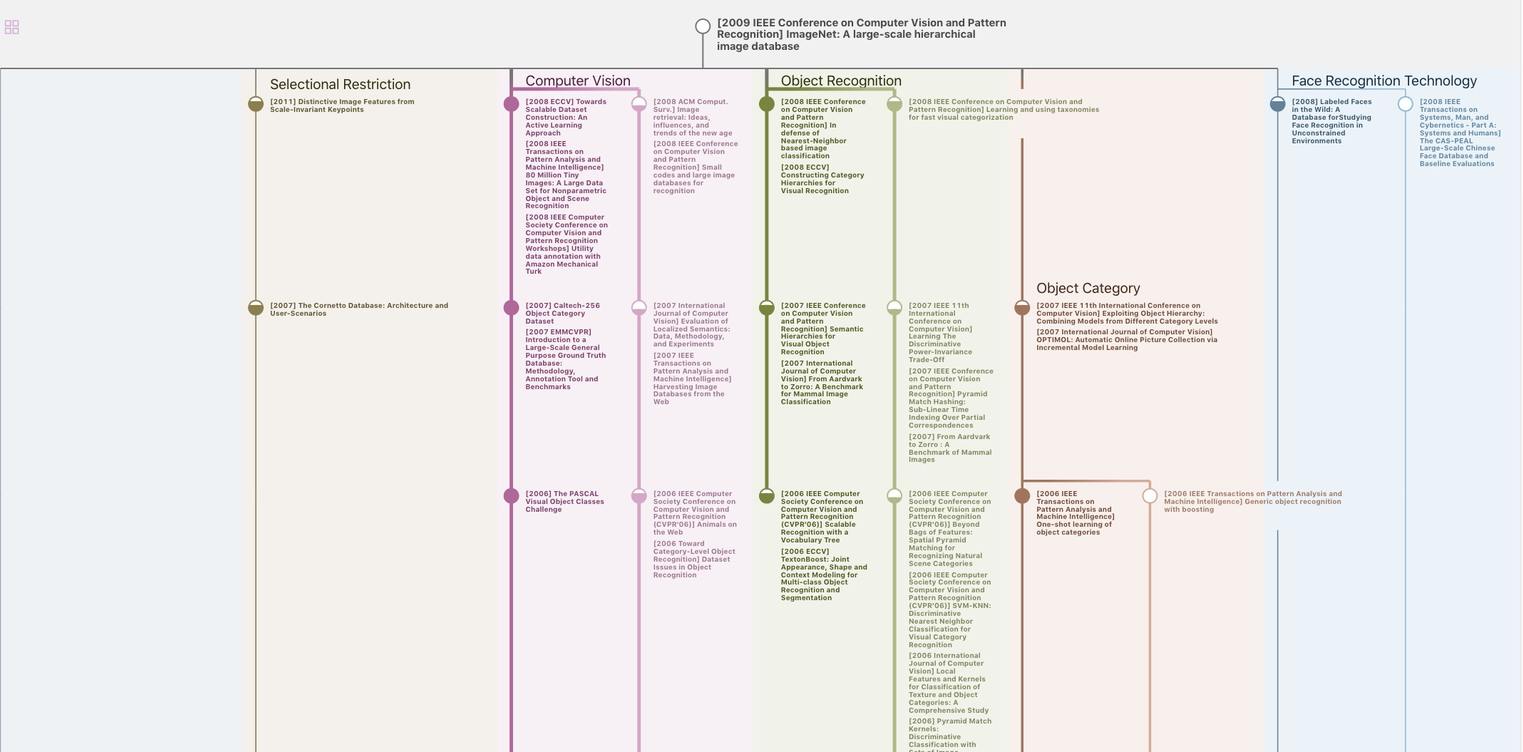
生成溯源树,研究论文发展脉络
Chat Paper
正在生成论文摘要