Incomplete Region Estimation and Restoration of 3D Point Cloud Human Face Datasets
SENSORS(2022)
摘要
Owing to imperfect scans, occlusions, low reflectance of the scanned surface, and packet loss, there may be several incomplete regions in the 3D point cloud dataset. These missing regions can degrade the performance of recognition, classification, segmentation, or upsampling methods in point cloud datasets. In this study, we propose a new approach to estimate the incomplete regions of 3D point cloud human face datasets using the masking method. First, we perform some preprocessing on the input point cloud, such as rotation in the left and right angles. Then, we project the preprocessed point cloud onto a 2D surface and generate masks. Finally, we interpolate the 2D projection and the mask to produce the estimated point cloud. We also designed a deep learning model to restore the estimated point cloud to improve its quality. We use chamfer distance (CD) and hausdorff distance (HD) to evaluate the proposed method on our own human face and large-scale facial model (LSFM) datasets. The proposed method achieves an average CD and HD results of 1.30 and 21.46 for our own and 1.35 and 9.08 for the LSFM datasets, respectively. The proposed method shows better results than the existing methods.
更多查看译文
关键词
3D point cloud, incomplete region, deep learning, estimation, and restoration
AI 理解论文
溯源树
样例
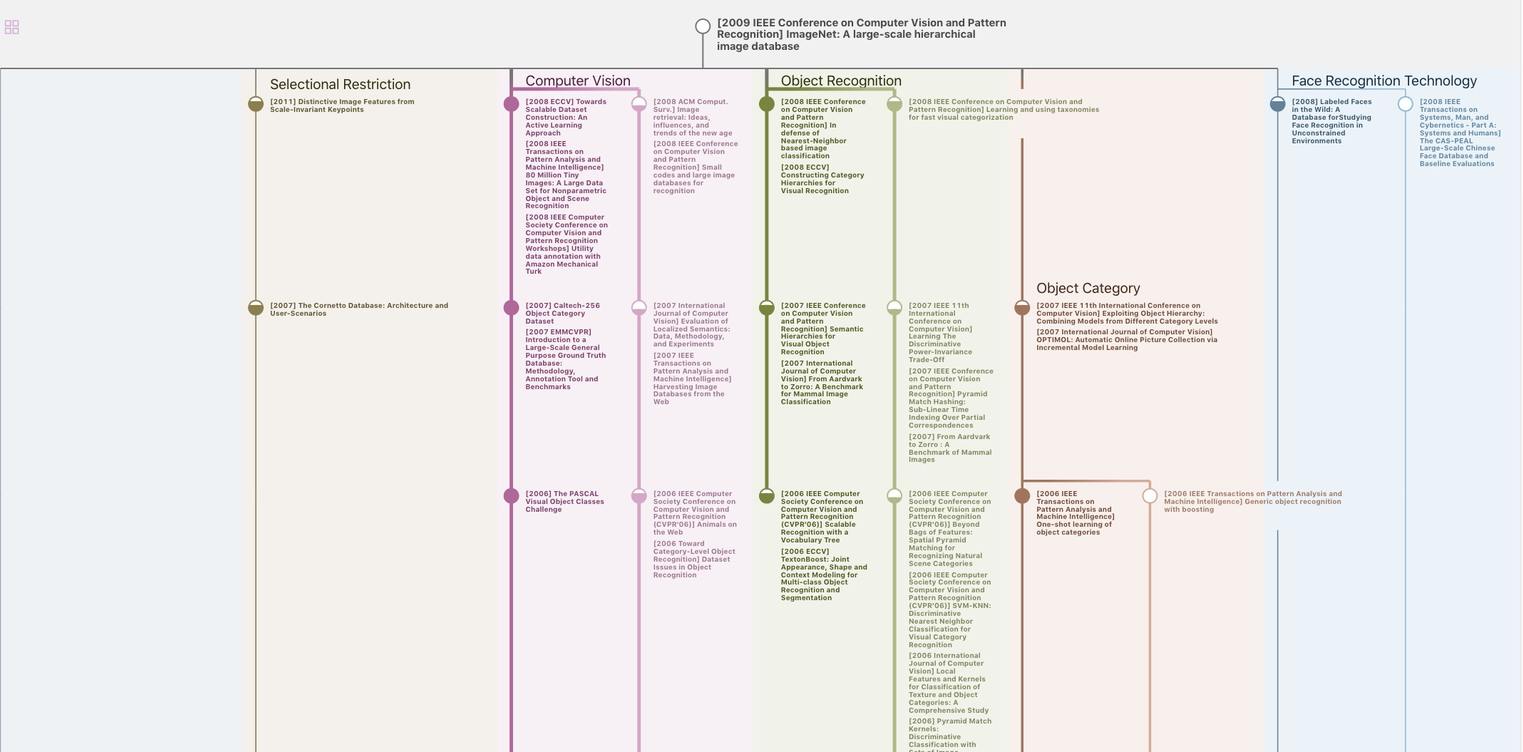
生成溯源树,研究论文发展脉络
Chat Paper
正在生成论文摘要