Damped Online Newton Step for Portfolio Selection.
Annual Conference on Computational Learning Theory(2022)
摘要
We revisit the classic online portfolio selection problem, where at each round a learner selects a distribution over a set of portfolios to allocate its wealth. It is known that for this problem a logarithmic regret with respect to Cover’s loss is achievable using the Universal Portfolio Selection algorithm, for example. However, all existing algorithms that achieve a logarithmic regret for this problem have per-round time and space complexities that scale polynomially with the total number of rounds, making them impractical. In this paper, we build on the recent work by Luo et al. 2018 and present the first practical online portfolio selection algorithm with a logarithmic regret and whose per-round time and space complexities depend only logarithmically on the horizon. Behind our approach are two key technical novelties. We first show that the Damped Online Newton steps can approximate mirror descent iterates well, even when dealing with time-varying regularizers. Second, we present a new meta-algorithm that achieves an adaptive, logarithmic regret (i.e. a logarithmic regret on any sub-interval) for mixable losses.
更多查看译文
关键词
portfolio selection,online newton step
AI 理解论文
溯源树
样例
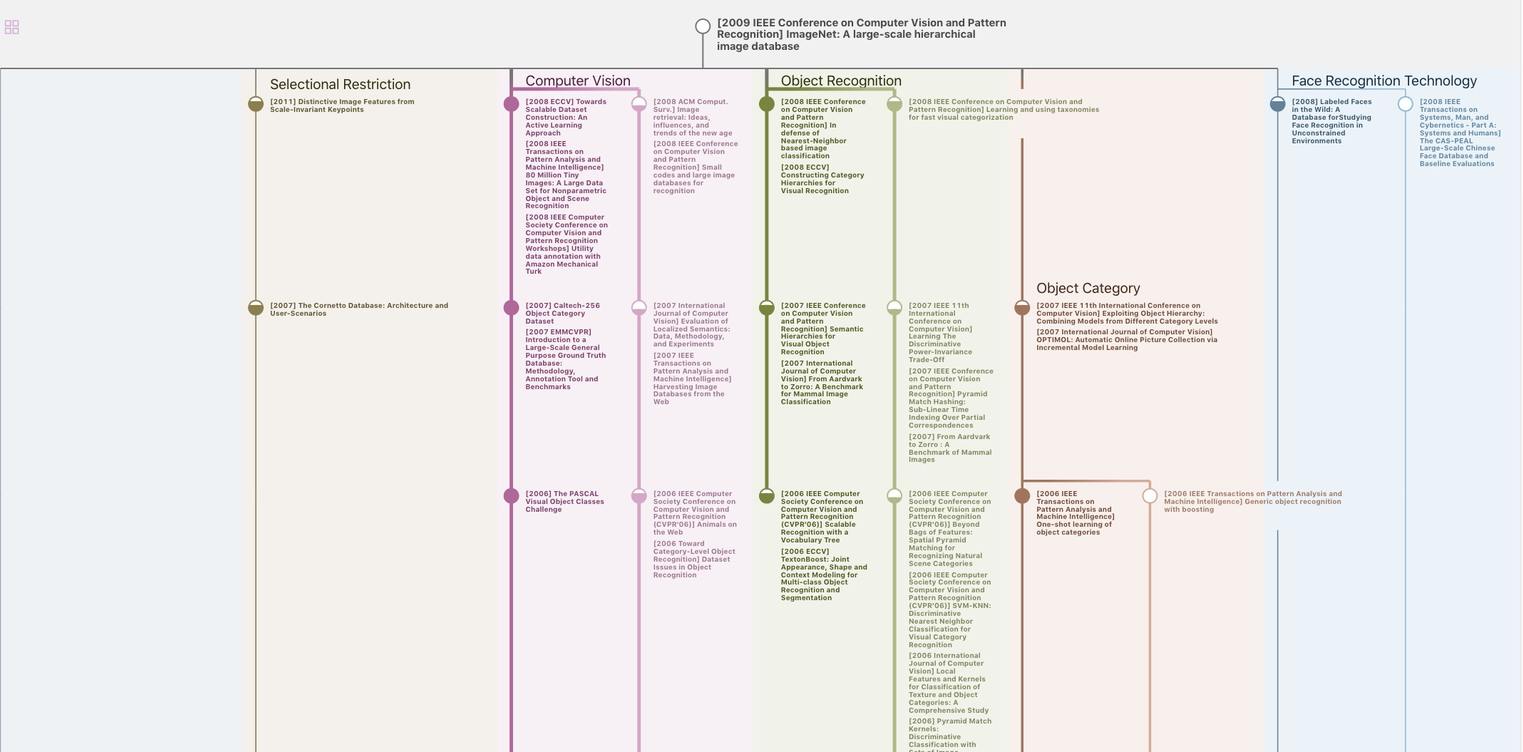
生成溯源树,研究论文发展脉络
Chat Paper
正在生成论文摘要